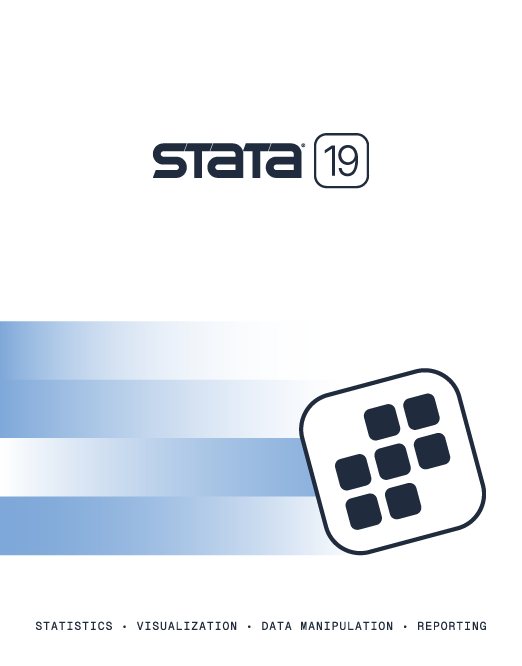
Stata's causal-inference suite allows you to estimate experimental-type causal effects from observational data. Whether you are interested in a continuous, binary, count, fractional, or survival outcome; whether you are modeling the outcome process or treatment process; Stata can estimate your treatment effect. With the most comprehensive set of causal-inference estimators available in any software package, you will find the one that's right for you.
Learn about causal inference and causal-inference analysis.
See what's new in causal inference.
Conditional average treatment effects with honest random forest New
Endogeneity, Heckman-style selection, and panel data with causal effects
Statistics
Treatments
Diagnostics
Difference-in-differences (DID) and triple-differences (DDD)
estimation
Treatment effects with high-dimensional controls
Additional resources
Watch Tour of treatment-effects estimators in Stata.
Watch Introduction to treatment effects, part 1.
Watch Introduction to treatment effects, part 2.
See New in Stata 19 to learn about what was added in Stata 19.