The Oceania Stata Conference was held on 20 August 2019 at the Park Royal Parramatta. There were also optional workshops on 19 August.
Proceedings
9:20–10:00 |
Abstract:
Observational data often have issues which present challenges for
the data analyst. The treatment status or exposure of interest is
often not assigned randomly. Data are sometimes missing not at random
(MNAR) which can lead to sample selection bias. And many statistical
models for these data must account for unobserved confounding. This
presentation will demonstrate how to use standard maximum likelihood
estimation to fit extended regression models (ERMs) that deal with
all of these common issues alone or simultaneously.
Additional information: oceania19_Huber.pptx
Chuck Huber
StataCorp
|
10:00–10:40 |
Abstract:
This presentation introduces a community-contributed Stata program for
technology forecasting using data envelopment analysis (TFDEA). TFDEA
was applied to predict the technological dynamics of smartphones. Datasets
with more than 5,000 observations were collected on the website
using data mining techniques. We compare the results with previous studies
and discuss data management for large datasets.
Additional information: oceania19_Lee.pdf
Choonjoo Lee
Korea National Defense University
Jinwoo Lee
Auckland University of Technology
|
11:00–11:20 |
Abstract:
Under the National Registration and Accreditation Scheme, the Australian
Pharmacy Council Ltd (APC) is the designated independent accreditation
agency for Australian pharmacies until June 2024. As part of this delegation
from the Pharmacy Board of Australia, the APC is responsible for the
delivery of high-stakes computer-delivered pharmacy examinations. These
include examinations held overseas that are part of the assessment process
for provisional registration of pharmacists in Australia. APC offers 12
examinations per year and develops several parallel forms per examination.
Until 2019, examination questions were stored in an inhouse database. Results were produced using a combination of the database facility and Excel programs. Individual item responses were analyzed employing a classical item theory approach using an Excel add-on and imported back to the database to inform future use of the items. In early 2019, APC moved its item bank into the ExamDeveloper software, which provides a range of management tools and the ability to store a wide range of variables to inform development of examinations. During 2019, APC has also progressively moved to implement a Rasch modeling approach. This presentation will describe the progress and outcomes of a project using Stata aimed to develop automated resulting; improve data management, integrity, and accountability; improve efficiency of calculations of item statistics, graphics, and tools for analysis of individual questions and examinations as a whole; and automate reporting to stakeholders as much as practicable. Additional information: oceania19_Robinson.pdf
Peter Robinson
National Manager Examination and Assessment
|
11:20–11:40 |
Abstract:
Unemployment is an important predictor of one's future employment success. Yet,
much about the endurance of unemployment effects on workers' careers and how they
evolve and play out over time remains poorly understood. Our study complements
this knowledge gap by examining the quality of career trajectories following an
unemployment spell among a representative sample of previously unemployed workers
with different sociodemographic characteristics in Germany. We apply a new dynamic
measure for sequence quality that extends Stata's sqset package to quantify
the quality of binary sequences, distinguishing between "good" (for example, employment)
and "bad" labor-force status activities (for example, unemployment and inactivity).
The advantage of this newly developed measure is that it captures the volatility of
labor-force trajectories and their evolution since the occurrence of an initial
unemployment spell. In addition, and more importantly, it quantifies the quality of
labor-force status trajectories dynamicly such that the measure decreases with
unfavorable activities (such as unemployment and inactivity) and increases with
favorable employment experiences. As such, this is the first sequence-based measure that
quantifies the overall quality of labor-force outcomes and thus the career recovery
from unemployment. We use longitudinal data from the German Socio-Economic Panel (GSOEP)
before the Great Financial Recession over the period 1984–2005 and deploy a series of
hybrid models that control for unobserved heterogeneity. Our results demonstrate a
deteriorating trend in career quality since an initial spell of unemployment. This
finding provides evidence for a cumulating disadvantage process following unemployment.
Furthermore, we also find that recovery processes are contingent upon when respondents
experience unemployment.
Additional information: oceania19_Mooi.pdf
Irma Mooi–Reci
University of Melbourne
|
11:40–12:00 |
Abstract:
Evaluation studies with observation data have been vastly enriched by the possibilities
offered by Stata's extended regresion model (ERM) framework, which can account simultaneously
for endogeneity at the covariate, treatment-assignment, and sample-selection levels, with a
robust clustering option. This presentation examines the challenges of building and testing
an extended regression model for estimating the causal effect of online engagement on first-year
academic outcomes. With reference to an exploratory counterfactual model, the presentation
makes a number of suggestions for the further development of the extended regression framework.
Additional information: oceania19_Tyler.pdf
Bill Tyler
Charles Darwin University
|
12:00–12:20 |
Abstract:
What is the relationship between age and life satisfaction or happiness? Do we become happier
when we age? Or do we experience a similar level of happiness throughout our lives? Are there
any patterns of at what age we are most satisfied with our lives? These questions have been
of particular interest to researchers in the area of psychology, economics, sociology, and
medicine. Despite numerous studies attempting to explore the relationship between age and
happiness, no agreed opinion was arrived at in the literature. Qualitatively different results
were reported by different studies that each was supported by convincing evidences. One of the
major studies in the world, utilizing data on millions of people from a wide range of countries,
claims that the level of happiness is U shaped in age. This U-shaped relation between age and
happiness, repeatedly confirmed by many other studies, has given rise to the prevailing saying
of the "midlife crisis" in many countries, including Australia. According to this U shape,
happiness improves with age after midlife. This seems to contradict with people's general
expectations because human health often falls with age. Arguments against the claimed U shape
became extremely controversial after a group of studies found convincing evidence that happiness
decreases with age after midlife. This group of studies used longitudinal data, and they controlled
for individual fixed effects. At some stage, the same data set produced completely different
results when used by different research groups, hence a mystery in the age-happiness research.
Additional information: oceania19_Li.pdf
Ning Li
AMSI
|
12:20–12:40 |
Abstract:
The instrumental variable (IV) method is a standard econometric approach to address endogeneity
issues (for example, when an explanatory variable is correlated with the error term). It relies
on finding an instrument, excluded from the outcome equation (second stage), but which is a
determinant of the endogenous variable of interest (first stage). Many instruments rely on
cross-sectional variation produced by a dummy variable, which is discretized from a continuous
variable. There might be several reasons for converting a continuous variable into a binary
instrument. First, continuous instruments recoded as dummies have been shown to provide a
parsimonious nonparametric model for the underlying first-stage relation (Angrist & Pischke, 2009).
Second, it provides a simple tool to evaluate the IV strategy and the identification assumptions.
Unfortunately, the construction of the binary instrument often appears to be arbitrary, which
may raise concerns about the robustness of the second-stage results.
I propose a data-driven procedure to build this discrete instrument, implemented in a command called discretise. The boundaries of the discrete variable are chosen to maximize the F-statistic in the first stage. This procedure has two main advantages: First, it minimizes the weak instrument problem, which can arise in case of incorrect functional specification in the first stage. Second, it offers a transparent, data-driven procedure to select an instrument that does not depend on arbitrary decisions made by the researcher. Several options are available with the command to check graphically the robustness of the first- and second-stage parameters. The presentation includes an explanation of the functioning of the discretize command, as well as an illustration of its usefulness with an example that relates the raise of violent crime in city centers and the process of suburbanization. The endogenous relation is solved using lead poisoning as instrument. Additional information: oceania19_Masera.pdf
Federico Masera
University of New South Wales
|
1:40–2:40 |
Abstract:
I am in the process of developing extensive web-based resources that teach what data visualization
using Stata from first principles. Some of these materials form part of a course that I teach at
the University of Sydney on Visual Data Analytics.
The free web resources offer two approaches for learning data visualization. The homepage will offer
a random palette of graphs for browsing content by type; this unstructured learning suits those
who are interested to find out how to make one of the several advanced graphical forms—the
Stata code for exact reproduction is provided at the end of each page. The website's main objective,
however, is to teach a structural workflow approach to data visualization using theory of graphs,
visual perception and statistical tools specifically designed for visual analysis.
Demetris Christodoulou
University of Sydney
|
2:40–3:00 |
Abstract:
The luminance-response function of the brief flash full-field photopic ERG rises to a peak before
falling to a sub-maximal plateau. Previous work has shown that the on and off responses that are
inherent in this suggest that the function can be modeled by the sum of a Gaussian density function
and a logistic distribution function. This talk discusses the nonlinear modelling to used to obtain
these functions and the advantages of using both Stata 15 and sigmaplot to obtain results.
Additional information: oceania19_Quinn.pdf
Steve Quinn
Swinburne University of Technology
|
4:00–4:30 |
Open panel discussion with Stata developers
StataCorp
|
Workshops 9:00 a.m. to 5:00 p.m.
Workshop 1: Using panel data in Stata
by Prof. Robert Breunig, Crawford School of Business, Australian National University
Agenda
- Setting up the data
- Descriptive statistics with panel data
- Regression methods with panel data
- Pooled OLS
- First differences
- Second (+higher) differences
- Fixed Effects
- Random Effects
- Advanced panel techniques
- Hausman–Taylor estimation
- Arellano–Bond estimation
Workshop 2: Using Stata for social statistics
by Dr. Con Menictas, Strategic Precision
Agenda
Stata provides powerful features for applied analysis and modeling of social data. Examples of social data include asset data, demographic, employment data, happiness measures, political, satisfaction, values, etc. This course teaches social data analysis using Stata by:
- Introducing participants to the different types of social data and the analysis of social data.
- Teaching participants Stata and statistical skills to interrogate social data using a variety of routines to produce applied solutions.
- Helping participants to look beyond aggregate models by looking deeper into the datasets to achieve better segmentation using Stata's powerful toolsets.
- Providing "hands-on" experience writing Stata code and generating highly reproducible and meaningful output
Giving participants insights to leading practices in the modeling, visualizing and reporting of social data.
Scientific committee
Dr. Gwin Nyakuengama (Chair)
DatAnalytics
Dr. Lydia Pikyi Cheung
Auckland University of Technology
Arul Earnest
Monash University
Erik Mooi
University of Melbourne
Demetris Christodoulou
University of Sydney
Logistics organizer
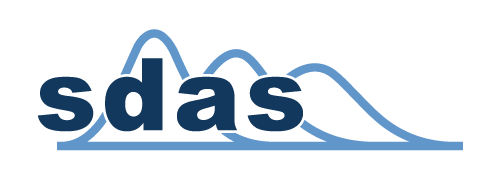
The logistics organizer for the 2019 Oceania Stata Conference is Survey Design and Analysis Services (SDAS), the distributor of Stata in Australia, Indonesia, and New Zealand.
View the proceedings of previous Stata Users Group meetings.