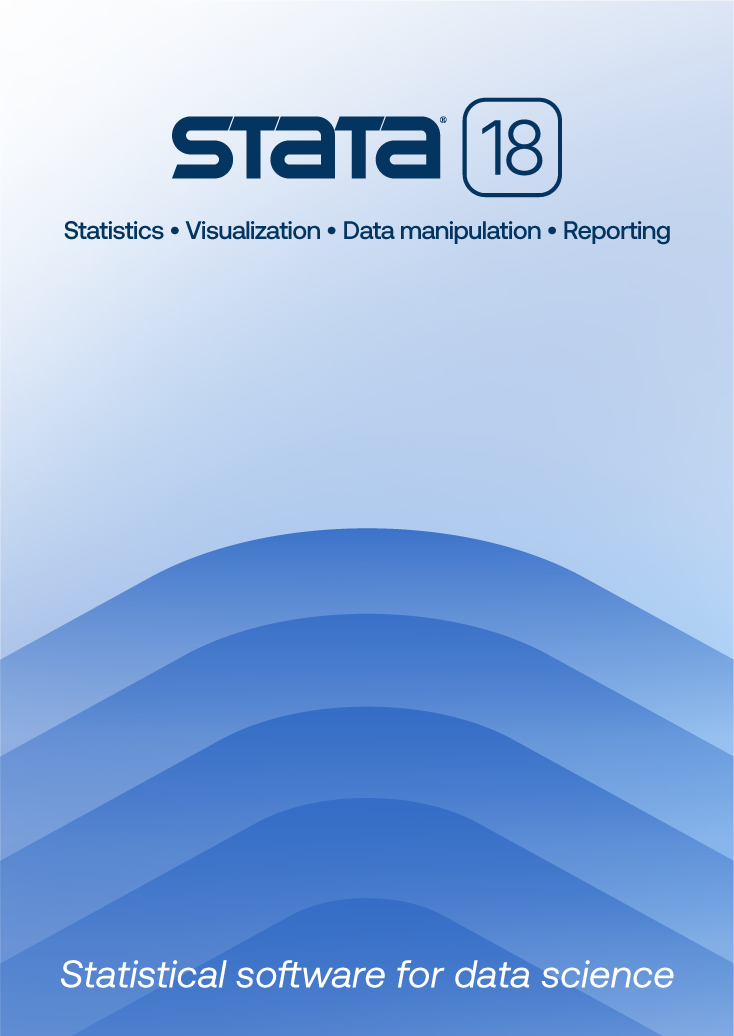
Last updated: 4 October 2013
Nobel Forum at Karolinska Institutet
Nobelkansliet
Box 270, STOCKHOLM
Sweden
Linear quantile mixed-effects models can estimate the median and other quantiles of an outcome variable of interest conditional on covariates when the data are dependent. Dependent data may arise from longitudinal, cluster, matched case–control studies and other popular study designs. A new command to estimate linear quantile mixed-effects models is being developed. The talk presents a prototype of the command and illustrates its use with an application to real data. The results are contrasted with those from ordinary linear mixed effects.
Additional materials:
se13_bottai.pdf
The talk describes recent updates for the popular glst command for estimating dose–response meta-regression models. The main features of the command are illustrated, and the major recent improvements are described. The update allows more flexibility when specifying covariance structures for correlated outcomes and the possibility of estimating two-stage multivariate models.
Additional materials:
se13_orsini.pdf
How to plot (and summarize) univariate distributions is a staple of introductory data analysis. Graphical (and numerical) assessment of marginal and conditional distributions remains important for much statistical modeling. Research problems can easily evoke needs for many comparisons, across groups, across variables, across models, and so forth. Over several centuries, many methods have been suggested, and their relative merits are a source of lively ongoing debate. I offer a selective but also detailed review of Stata functionality for univariate distributions. The presentation ranges from official Stata commands through various user-written commands, including some new programs, to suggestions on how to code your own graphics commands when other sources fail. I also discuss both continuous and discrete distributions. The tradeoff between showing detail and allowing broad comparisons is an underlying theme.
Additional materials:
se13_cox.ppt
After reviewing the potential-outcome framework for estimating treatment effects from observational data, this talk discusses how to estimate the average treatment effect and the average treatment effect on the treated by the regression-adjustment estimator, the inverse-probability weighted estimator, two doubly robust estimators, and two matching estimators implemented in teffects.
Additional materials:
se13_drukker.pdf
In this presentation, I discuss ways of measuring segregation and suggest that a measure of segregation as overexposure has many attractive properties. The measure proposed can be interpreted to mean that the average individual in a given group X has n times as many colleagues or neighbors of group Y as would be expected under random allocation conditional on the distribution of covariates. The implementation of this measure in Stata as well as some results based on analyses of Swedish register data will be presented.
Additional materials:
se13_skans.pptx
The study of the networks within which individuals are embedded has emerged as a central area of research in several social science disciplines. In this presentation, a new set of Stata tools for visualizing and analyzing core properties of social networks is presented.
Additional materials:
se13_grund.pptx
Data augmentation is a technique for conducting Bayesian or semi-Bayesian regression analysis. It exploits commands that are readily available in familiar statistical software, such as the Stata command glm. This technique is a form of penalized likelihood estimation where prior information, represented by one or more prior data records created ad hoc by the data analyst, generates a penalty function that imposes the desired priors on the regression coefficients. We illustrate the advantages, use, and interpretation of this procedure through examples from epidemiological studies.
Additional materials:
se13_discacciati.pdf
Visualizing the true effect of a predictor over a range of values can be difficult for models that are not parameterized in their natural metric, such as for logistic or (even more so) probit models. Interaction terms in such models cause even more difficulty. In this talk, I show how both the margins and the marginsplot commands can make for much clearer explanations of effects for both nonstatisticians and statisticians alike.
Additional materials:
se13_rising.pdf
Peter Hedström, Metrika Consulting and Institute for Futures StudiesNicola Orsini, Karolinska Institutet
Matteo Bottai, Karolinska Institutet
Metrika Consulting, the official distributor of Stata in the Nordic and Baltic regions, and the Karolinska Institutet.