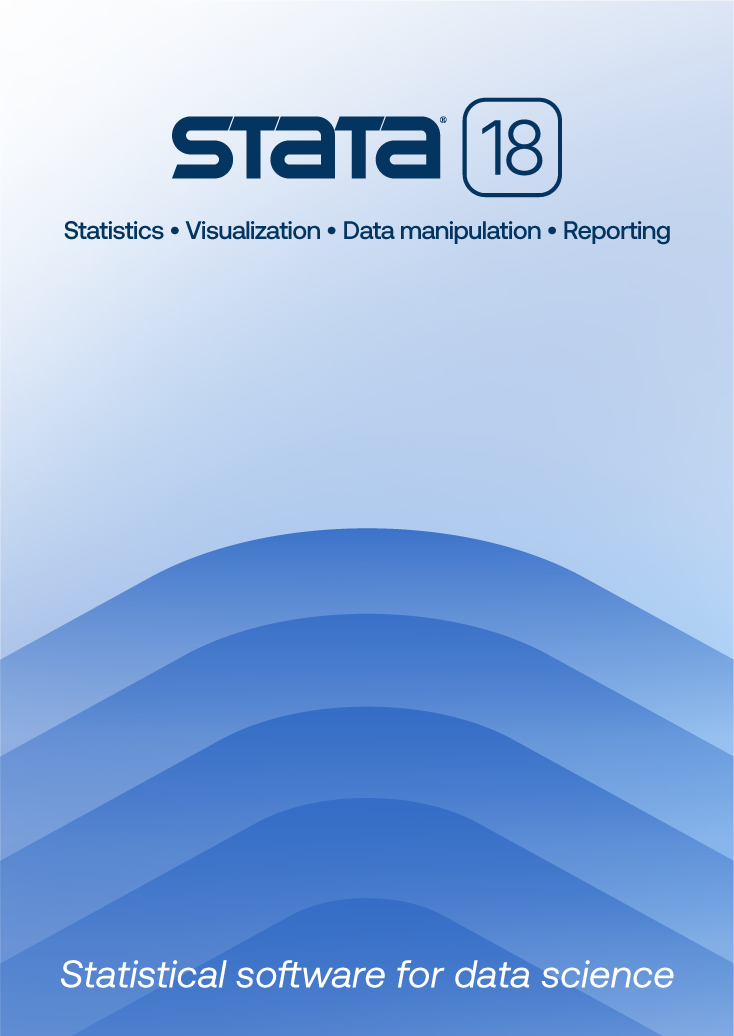
Title | How to calculate power using simulation | |
Authors |
Alan Feiveson, NASA Chuck Huber, StataCorp Mia Lv, StataCorp |
Power and sample size analysis is an important tool for planning your experiments. Stata's power command has several methods implemented that allow us to compute power or sample size for tests on means, proportions, variances, regression slopes, case-control analysis, and survival analysis, among others. For those complicated models that are not directly supported by the power suite of commands, we can consider using simulations to obtain the power. In Stata, we can use simulate to perform Monte Carlo simulations. We can also integrate our simulations into Stata's power commands so that we can easily create custom tables and graphs for a range of parameter values.
This FAQ is organized as follows:
Statistical power is the probability of rejecting the null hypothesis when the null hypothesis is false. The general procedure for calculating power using Monte Carlo simulations is
The proportion of times that the null hypothesis is rejected (out of N) is our estimate of power.
Note: As a check, always run the simulation for the null case to make sure the estimate power is approximately α (to within the sampling error of the iteration number). More generally, in the null case, the distribution of the p-values should present a uniform distribution within the [0,1] interval.
Let's look at one example. Assume that we wish to test the effect of a drug dose on a Poisson-distributed response y in rats. For a sample of k rats, we have the Poisson regression model
log \( \mu_{i} = b_{1}x_{i} \)
\( y_{i} \tilde\ \mathrm{Poisson}(\mu_{i}) \)
for i=1, ..., k, where \( x_i \) is the dose in milligrams given to the \(i_{th} \) rat. Suppose we are trying to decide what sample size would give reasonable power for the test \( b_1=0 \) (the null hypothesis) if the true value of \( b_1 \) were 0.64 (the alternative hypothesis), under an experimental design with three levels of dosage (0.2, 0.5, and 1.0) repeated n times on \( k = 3n \) different rats.
Let's define a program to implement the steps 1–3 that we introduced above. For more instruction on how to use program to write a simple program in Stata, please read the documentation for [P] program.
capture program drop powersimu program powersimu, rclass version 17.0 // DEFINE THE INPUT PARAMETERS AND THEIR DEFAULT VALUES syntax, n(integer) /// sample size for each dosage group b1(real) /// b1 under the alternative [ alpha(real 0.05) /// alpha level d1(real 1) /// fixed dosage level 1 d2(real 2) /// fixed dosage level 2 d3(real 3) /// fixed dosage level 3 ] // GENERATE THE RANDOM DATA AND TEST THE NULL HYPOTHESIS clear set obs 3 generate x=`d1' in 1 replace x=`d2' in 2 replace x=`d3' in 3 expand `n' generate mu=exp(`b1'*x) // generate random numbers as dependent variable in the Poisson regression gen y = rpoisson(mu) // fit the Poisson regression poisson y x, noconstant // RETURN RESULTS mat a=r(table) local p1=el(a,rownumb(a,"pvalue"),colnumb(a,"x")) return scalar pvalue = `p1' return scalar reject = (`p1'<`alpha') end
You could save the above program in an ado-file called powersimu.ado. Now we can run the program powersimu with the input parameters, and it will give you the result of one iteration. Please note that the above program calls the random-number generator so that we use set seed to get reproducible results.
. set seed 1234 . powersimu, n(10) b1(0.64) d1(.2) d2(.5) d3(1.0) alpha(0.05) . return list
The output is
scalars: r(reject) = 1 r(pvalue) = .0000330350943392
In this iteration, the null hypothesis \( (b_1 = 0) \) was rejected because the p-value is smaller than α.
In order to estimate the power by repeating the above procedure, we can run this program with the simulate prefix.
. simulate reject=r(reject) pvalue=r(pvalue), reps(1000) seed(1234): powersimu, n(10) b1(0.64) d1(.2) d2(.5) d3(1.0) alpha(0.05)
In the above line, we specify 1000 as the number of replications in the simulation. We also set the random-number seed for the simulation by specifying the seed() option in order to get reproducible results. After we run the simulation, the test results and p-values are saved in the new variables reject and pvalue, respectively. You can use summarize to calculate the mean of reject, which equals the proportion of times out of 1000 iterations that the null hypothesis was rejected. That proportion is your estimate of statistical power given the input parameters.
. summarize reject
Variable | Obs Mean Std. dev. Min Max | |
reject | 1,000 .788 .4089294 0 1 |
In this example, the proportion equals 0.788, which means that you can expect 78.8% power for this test when the coefficient of drug dose equals 0.64, given a sample size of 30. You could increase the number of replications in the simulation to get a more accurate power estimate.
Now, we would like to run the program with \( b1 = 0 \) with a relatively large value of N (replication number) to see if the proportion of rejections is close to α. If not, then either the normal approximation used by the command is not adequate for this case or we may have programmed the procedure incorrectly. Let's run the simulation with N = 1500.
. simulate reject=r(reject) pvalue=r(pvalue), reps(1500) seed(1234): powersimu, n(10) b1(0) d1(.2) d2(.5) d3(1.0) alpha(0.05)
Then, we can estimate the proportion of observations with reject = 1 using ci proportion. The point estimate of proportion is .0546667, and a 95% confidence interval is (0.0437109, 0.0674042), given α = 0.05.
. ci proportion reject
Binomial exact | ||
Variable | Obs Proportion Std. err. [95% conf. interval] | |
reject | 1,500 .0546667 .0058696 .0437109 .0674042 |
Now, let's check the entire distribution of simulated p-values for a uniform distribution using histogram. We can see that it is approximately uniform distributed.
. histogram pvalue
These results suggest that the normal approximation z test is quite good for 30 observations (n = 10).
With the power suite of commands, we can specify multiple values for each parameter such as the coefficient, the standard deviation, the means, or the α level. And power will create custom tables and graphs that present the results under multiple parameter values. In addition, with power we can add our own methods to the suite of commands. We will show you how to add the Poisson regression simulation program we just created to power. First, we need to define a program named power_cmd_mymethod. In this program, we need to save the power, sample size, and other parameters as the stored results. Because we already know how to obtain power using the program powersimu and simulate, the new program will simply call powersimu to obtain the power.
capture program drop power_cmd_powersimu program power_cmd_powersimu, rclass version 17.0 // DEFINE THE INPUT PARAMETERS AND THEIR DEFAULT VALUES syntax, n(integer) /// sample size for each dosage group b1(real) /// b1 under the alternative [ alpha(real 0.05) /// Alpha level d1(real 1) /// fixed dosage level 1 d2(real 2) /// fixed dosage level 2 d3(real 3) /// fixed dosage level 3 reps(integer 100) /// Number of repetitions ] // GENERATE THE RANDOM DATA AND TEST THE NULL HYPOTHESIS // call the powersimu program quietly simulate reject=r(reject), reps(`reps') seed(12345): /// powersimu, n(`n') b1(`b1') d1(`d1') d2(`d2') d3(`d3') alpha(`alpha') quietly summarize reject // RETURN RESULTS return scalar power = r(mean) return scalar N = 3*`n' return scalar alpha = `alpha' return scalar b1 = `b1' end
You can save the above program in an ado-file called power_cmd_powersimu.ado.
We've almost finished the work now. But you will need to write one more small initializer program if you wish to enter a range of values for the parameters other than n() or alpha(). The program must be named power_cmd_mymethod_init, so we will name our program power_cmd_powersimu_init.
The initializer program is defined as a sclass program. In the program, the line sreturn local pss_colnames initializes columns in the output table for the parameters listed in double quotes. The line sreturn local pss_numopts allows you to specify numlists for the parameters placed in double quotes. For more detailed information regarding writing the initializer program for user-defined power commands, please read the entry to [PSS] power usermethod.
You can save the following program in an ado-file called power_cmd_powersimu_init.ado.
capture program drop power_cmd_powersimu_init program power_cmd_powersimu_init, sclass sreturn clear sreturn local pss_numopts "b1" sreturn local pss_colnames "b1" end
Now, we can use power powersimu to calculate power for a range of coefficient values assuming different alternative hypotheses and a range of sample sizes. Please note that power powersimu calls the program power_cmd_powersimu rather than powersimu. In the generated table, we can easily see the estimated power values for different input parameters. And we can plot the power analysis results by specifying the graph option. You can also change the x-dimension variable in your plot by specifying xdimension in the graph option. Please note that the sample size equals 3*n (repeat time in each dosage level) in this power analysis because we have three levels of drug dosage.
. program drop _all . power powersimu, reps(1000) n(10(10)30) b1(0.5(0.05)0.85) d1(.2) d2(.5) d3(1.0) table graph(xdimension(b1)) alpha(0.05)
Estimated power Two-sided test
alpha power N b1 |
.05 .58 30 .5 |
.05 .673 30 .55 |
.05 .745 30 .6 |
.05 .813 30 .65 |
.05 .849 30 .7 |
.05 .912 30 .75 |
.05 .945 30 .8 |
.05 .974 30 .85 |
.05 .842 60 .5 |
.05 .896 60 .55 |
.05 .951 60 .6 |
.05 .977 60 .65 |
.05 .985 60 .7 |
.05 .994 60 .75 |
.05 1 60 .8 |
.05 1 60 .85 |
.05 .946 90 .5 |
.05 .979 90 .55 |
.05 .992 90 .6 |
.05 .997 90 .65 |
.05 1 90 .7 |
.05 1 90 .75 |
.05 1 90 .8 |
.05 1 90 .85 |
As we can see in the graph, a larger true value of \( b_1 \) will lead to a larger power because there is a bigger chance to reject the null hypothesis \( b_1=0 \) when the true value of \( b_1 \) is larger. The graph also suggests that the increase in power is substantial between N = 30 and N = 60 and becomes less beneficial when N is beyond 60.
On the other hand, if we do not specify the xdimension of graph, the sample size N will be the xdimension by default. If we run the following power command with the notable option, the table will be suppressed and we will see only the graph.
. power powersimu, reps(1000) n(10(5)30) b1(0.5(0.1)0.7) d1(.2) d2(.5) d3(1.0) graph alpha(0.05) notable
As expected, as sample size increases, power increases. As power gets closer to 1, the effect of increasing the sample size becomes smaller.