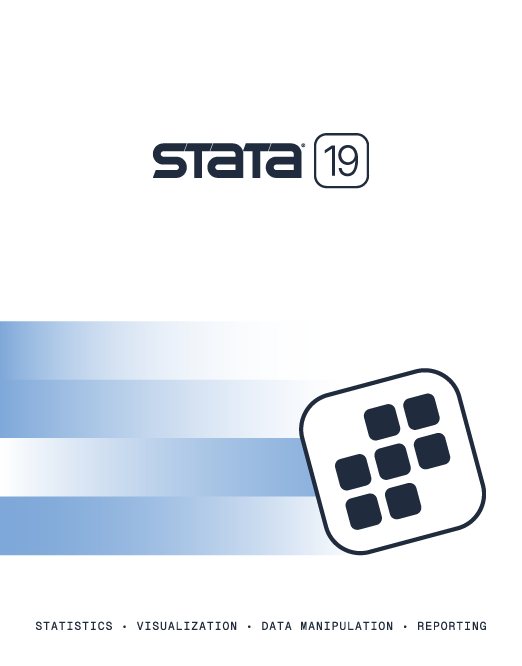
Title | Fisher’s exact test two-sided idiosyncrasy | |
Author | Wesley Eddings, StataCorp |
Stata’s exact confidence interval for the odds ratio inverts Fisher’s exact test. We might expect the interval and test to agree on statistical significance, but this is not always the case. Here is an example:
. cci 2 31 136 15532, exact Proportion | Exposed Unexposed | Total Exposed -----------------+------------------------+------------------------ Cases | 2 31 | 33 0.0606 Controls | 136 15532 | 15668 0.0087 -----------------+------------------------+------------------------ Total | 138 15563 | 15701 0.0088 | | | Point estimate | [95% Conf. Interval] |------------------------+------------------------ Odds ratio | 7.368121 | .845817 29.44578 (exact) Attr. frac. ex. | .8642802 | -.1822888 .9660393 (exact) Attr. frac. pop | .0523806 | +------------------------------------------------- 1-sided Fisher's exact P = 0.0339 2-sided Fisher's exact P = 0.0339
The p-value is significant at the 5% level, but the confidence interval is not (it includes the null value of one). The test and interval disagree even though they were derived from the same model.
There is no problem with Stata’s implementation of the test or interval. The problem is the difficulty in two-sided inference from asymmetric sampling distributions. Fisher’s exact test handles the difficulty in one way, the interval in another way.
The test naturally gives a one-sided p-value, and there are at least four different ways to convert it to a two-sided p-value (Agresti 2002, 93). One way, not implemented in Stata, is to double the one-sided p-value; doubling is simple but can result in p-values larger than one.
Stata instead adds the probabilities of all the tables at least as unlikely as the observed table. (For a rigorous statement, see Methods and Formulas of [R] tabulate twoway). In our example, all the “unlikelier” tables are in the same tail as the observed table. The other tail does not contribute to the p-value, so the one-sided and two-sided p-values are equal.
However, the other tail is included in the confidence interval, because the confidence interval inverts two one-sided tests, not a two-sided test (Example 10 of [R] epitab; Breslow and Day 1980, 128–129). That is why the interval disagrees with the p-value.
The interval and p-value can disagree even though they are both “exact” because it is not the coverage probability and type I error probability that are exact. The coverage probability is not exactly 0.95, and the type I error probability is not exactly 0.05. (The 0.95 is a lower bound, and the 0.05 is an upper bound.) The underlying sampling distribution is discrete, so it is not possible to create a nonrandomized confidence interval with a coverage probability of 0.95 or a nonrandomized test with a type I error probability of 0.05.