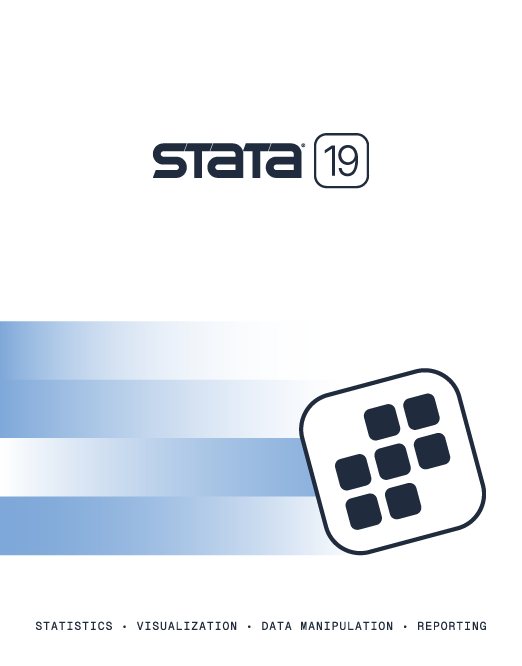
2025 Stata Conference • Nashville, TN • 31 July–01 August
Bayesian analysis is a statistical paradigm that answers research questions about unknown parameters using probability statements.
For example, what is the probability that the average male height is between 70 and 80 inches or that the average female height is between 60 and 70 inches? What is the probability that people in a particular state vote Republican or vote Democratic? What is the probability that a person accused of a crime is guilty? What is the probability that treatment A is more cost effective than treatment B for a specific health care provider? What is the probability that a patient's blood pressure decreases if he or she is prescribed drug A? What is the probability that the odds ratio is between 0.3 and 0.5? What is the probability that three out of five quiz questions will be answered correctly by students? What is the probability that children with ADHD underperform relative to other children on a standardized test? What is the probability that there is a positive effect of schooling on wage? What is the probability that excess returns on an asset are positive? And many more.
Such probabilistic statements are natural to Bayesian analysis because of the underlying assumption that all parameters are random quantities. In Bayesian analysis, a parameter is summarized by an entire distribution of values instead of one fixed value as in classical frequentist analysis. Estimating this distribution, a posterior distribution of a parameter of interest, is at the heart of Bayesian analysis.
A posterior distribution comprises a prior distribution about a parameter and a likelihood model providing information about the parameter based on observed data. Depending on the chosen prior distribution and likelihood model, the posterior distribution is either available analytically or approximated by, for example, one of the Markov chain Monte Carlo (MCMC) methods.
Bayesian inference uses the posterior distribution to form various summaries for the model parameters, including point estimates such as posterior means, medians, percentiles, and interval estimates known as credible intervals. Moreover, all statistical tests about model parameters can be expressed as probability statements based on the estimated posterior distribution.
Unique features of Bayesian analysis include an ability to incorporate prior information in the analysis, an intuitive interpretation of credible intervals as fixed ranges to which a parameter is known to belong with a prespecified probability, and an ability to assign an actual probability to any hypothesis of interest.
To learn more about Bayesian analysis, see [BAYES] intro. For a quick overview of Bayesian features, click here.
Read the overview from the Stata News.
Upgrade now Order StataLearn
Free webinars
NetCourses
Classroom and web training
Organizational training
Video tutorials
Third-party courses
Web resources
Teaching with Stata
© Copyright 1996–2025 StataCorp LLC. All rights reserved.
×
We use cookies to ensure that we give you the best experience on our website—to enhance site navigation, to analyze usage, and to assist in our marketing efforts. By continuing to use our site, you consent to the storing of cookies on your device and agree to delivery of content, including web fonts and JavaScript, from third party web services.
Cookie Settings
Last updated: 16 November 2022
StataCorp LLC (StataCorp) strives to provide our users with exceptional products and services. To do so, we must collect personal information from you. This information is necessary to conduct business with our existing and potential customers. We collect and use this information only where we may legally do so. This policy explains what personal information we collect, how we use it, and what rights you have to that information.
These cookies are essential for our website to function and do not store any personally identifiable information. These cookies cannot be disabled.
This website uses cookies to provide you with a better user experience. A cookie is a small piece of data our website stores on a site visitor's hard drive and accesses each time you visit so we can improve your access to our site, better understand how you use our site, and serve you content that may be of interest to you. For instance, we store a cookie when you log in to our shopping cart so that we can maintain your shopping cart should you not complete checkout. These cookies do not directly store your personal information, but they do support the ability to uniquely identify your internet browser and device.
Please note: Clearing your browser cookies at any time will undo preferences saved here. The option selected here will apply only to the device you are currently using.