In the spotlight: eteffects and the challenges of making causal inferences
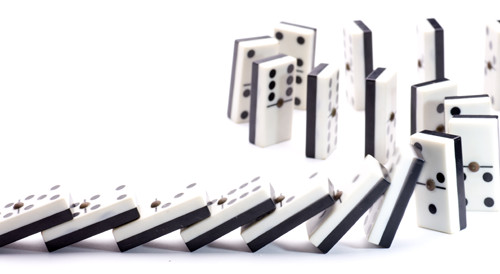
The challenge
Extracting causal relationships from data is one of the fundamental endeavors of researchers. Ideally, we could conduct a controlled experiment to extract causal relations. However, a controlled experiment is rarely feasible for researchers or individuals who need to make informed decisions based on their available observational data.
In the absence of experimental data, we construct models to capture the relevant features of the causal relationship we are interested in. This is the purview of everything in Stata's [TE] Causal Inference and Treatment-Effects Estimation Reference Manual.
The estimators in teffects help us obtain estimates of the effect of a treatment (for example, a job training program or an increase in out-of-pocket contributions for a health plan) on an outcome (for example, probability of employment or enrollment in a health plan). With these traditional treatment-effect models, in order to interpret our results causally, assignment to a treatment must be independent of the outcome. What if that is not true? What if, for example, the individuals that participate in a job training program are highly motivated? Then the outcome of a higher probability of employment might be correlated to the person's inherent motivation rather than participation in the job training program.
Stata's endogenous treatment-effects command, eteffects, is designed for such cases. The key assumption behind the model is that treatment assignment is not independent of outcomes because the unobservables that affect treatment assignment and outcomes are correlated. If we incorporate this correlation into our model, we can obtain a causal effect. Using this model, we can also test whether the correlation is statistically significant; in other words, we can test for endogeneity.
The tool at work
Say we are interested in the effect of attending a private high school (private) on college grade point average (gpa). We conjecture that the quality of the available private schools affects the decision of parents to send their kids there and affects the college GPA. If this is the case, eteffects is a good alternative.
We model private as a function of parental income (income), whether the student lived in an urban area (urban), and, because of the prevalence of Catholic private schools, whether the student's parents are Catholic (catholic). We model gpa as a function of high school GPA (hgpa) and the parents' joint educational attainment (pedu).
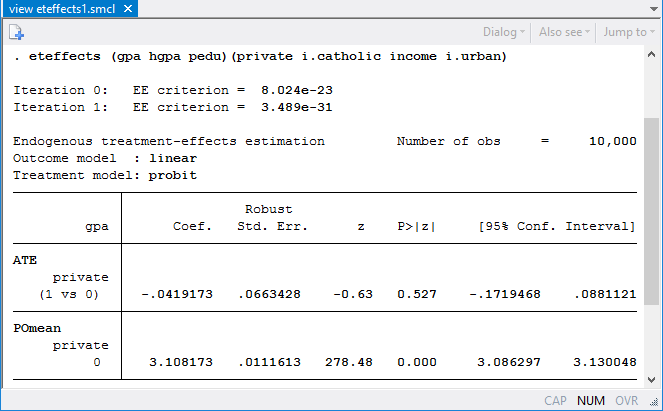
Our estimates show no evidence that the average treatment effect of attending private school is not zero. That is, in terms of college GPA, we have no evidence of differences between all kids attending a private school versus all kids attending a public school.
We can also test the assumption that the unobservables that affect the treatment assignment also affect the outcome.
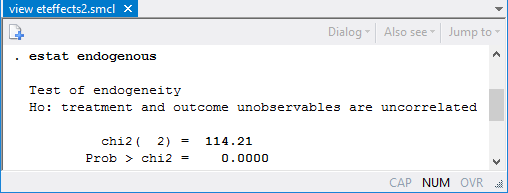
In this case, we reject the null hypothesis. We have strong evidence that the unobservables are correlated.
What if we had assumed that the unobservables that affect treatment assignment do not affect the outcome? We could have used one of the teffects estimators, say, the inverse-probability-weighted regression adjustment. We would get the following:
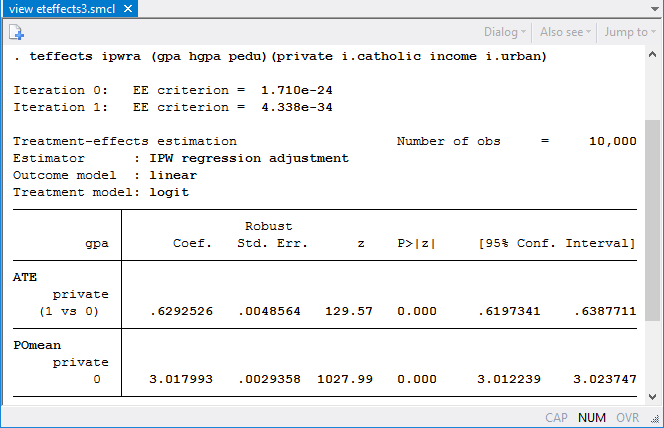
This suggests that the average treatment effect of attending private school is an increase in GPA of 0.63, which is a very different conclusion than we reached when we accounted for the unobservables affecting both GPA and choice to attend private school.
Closing remarks
The example above uses artificial data. I know that the assumptions necessary for using eteffects are met and that the true average treatment effect should be exactly 0. Obviously, researchers face a much more daunting challenge to ascertain causality, but eteffects is a valuable tool in this endeavor.
—Enrique Pinzon
Senior Econometrician, StataCorp LP