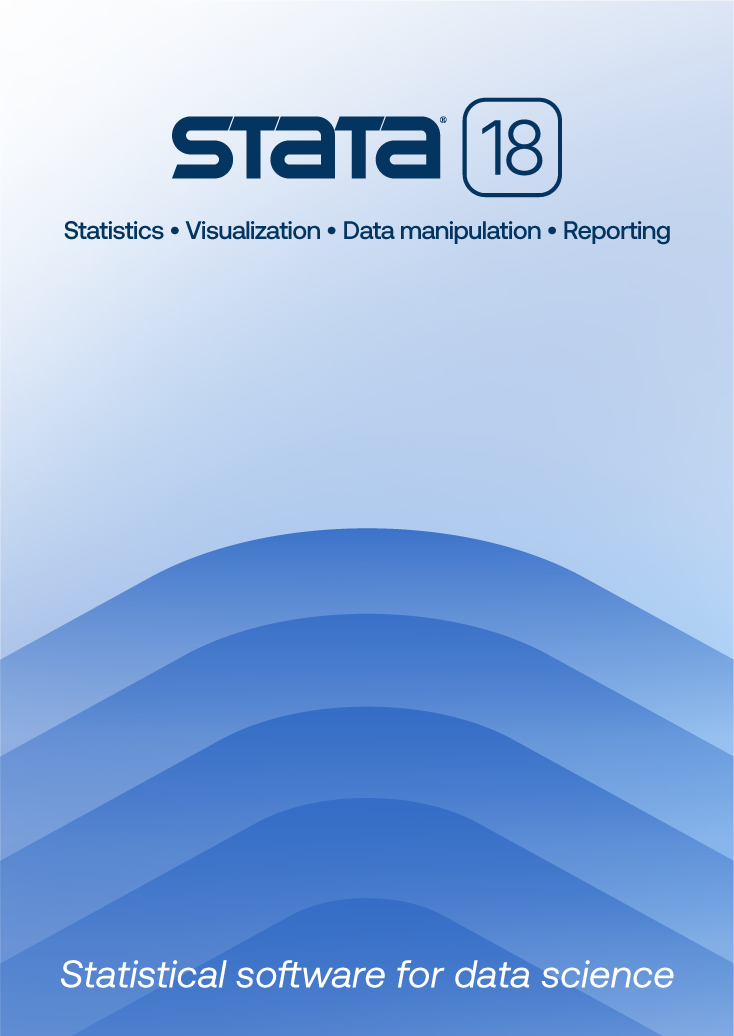
StataCorp will be exhibiting and hiring at the 2024 Joint Statistical Meetings. We're also hosting two computer technology workshops. Attending from StataCorp: Yulia Marchenko, Vice President, Statistics and Data Science; Aramayis Dallakyan, Senior Statistician and Software Developer; Meghan Cain, Assistant Director, Educational Services; and Yaojin Sun, Senior Statistician and Software Developer. For more information about the meeting, visit the JSM 2024 page.
Try Stata for free by requesting a short-term evaluation license. To be notified of future software releases, free webinars, classroom and web-based training courses, and more, sign up for email alerts.
Title: | Causal mediation analysis using Stata |
Presenter: | Aramayis Dallakyan, Senior Statistician and Software Developer, StataCorp |
Date: | Wednesday, 7 August 2024 |
Time: | 1:00–2:45 p.m. |
Location: | Room B113 of the Oregon Convention Center |
Description: | Causal mediation analysis (CMA) aims to explore and determine the mechanisms through which a treatment influences an outcome via a mediator. The objective of this workshop is to provide a practical guide, facilitating an understanding of CMA using Stata. We will begin by introducing the fundamental steps of causal analysis and applying them to CMA. We will highlight the differences between causal and traditional mediation analysis. The workshop will also delve into various types of direct and indirect effects. Examples demonstrating how to perform CMA within Stata using different types of outcomes and mediators (continuous, binary, and count) will be provided. No prior knowledge of Stata is required, although a basic understanding of causal inference will be beneficial. |
Title: | Bayesian model averaging using Stata |
Presenter: | Yulia Marchenko, Vice President, Statistics and Data Science, StataCorp |
Date: | Wednesday, 7 August 2024 |
Time: | 3:30–4:45 p.m. |
Location: | Room B113 of the Oregon Convention Center |
Description: | Model uncertainty accompanies many data analyses. Bayesian model averaging (BMA) helps address this uncertainty. For instance, in the regression context, we may want to know which predictors are important given the observed data, which models are more plausible, or how predictors relate to each other across different models. BMA can answer these and more questions. BMA uses the Bayes theorem to aggregate the results across multiple candidate models to account for model uncertainty during inference and prediction in a principled and universal way. In this workshop, I will describe the basics of BMA and demonstrate it with Stata's bma suite. I will also show how BMA can become a useful tool for your regression analysis, Bayesian or not! No prior knowledge is required, but familiarity with Bayesian analysis will prove useful. |
Aramayis Dallakyan is a Senior Statistician and Software Developer at StataCorp LLC. His research interests lie at the intersection of high-dimensional time series, causal discovery, and statistical/machine learning. Aramayis has published articles in renowned journals, including the Journal of Graphical and Computational Statistics, the Journal of Computational Statistics and Data Analysis, and the Stata Journal. Prior to joining StataCorp, he earned a PhD in statistics from Texas A&M University.
Yulia Marchenko is Vice President, Statistics and Data Science at StataCorp. Yulia has been with Stata for almost 20 years and has more than 25 years of software development experience. Her primary responsibility is to oversee Stata's scientific software development. Her areas of interest include Bayesian analysis, multiple imputation, meta-analysis, multilevel modeling, survival analysis, power analysis, causal analysis, and other areas of statistics, biostatistics, and econometrics. Yulia has a PhD in statistics from Texas A&M University.
Browse the Stata flyers below.
View all Stata features.
Visit stata-press.com for more information.
Visit stata-journal.com for more information.