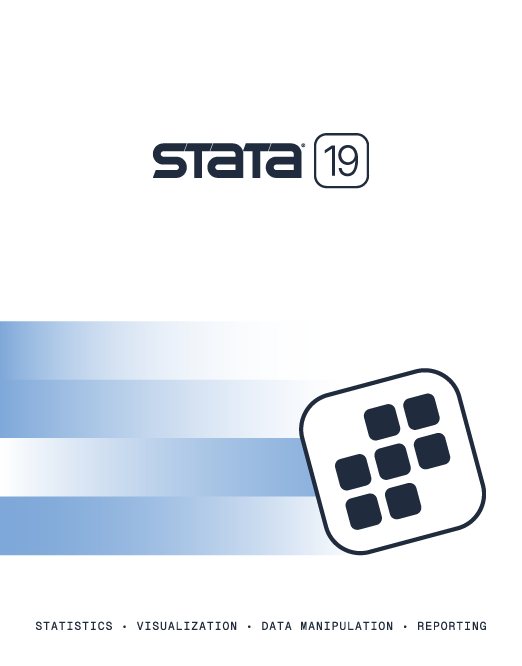
9:05–9:30 | Too much or too little? New tools for the CCE estimator
Abstract:
This talk will cover new developments in the literature of
common correlated effects (CCE) and their implementation into
Stata. First, I will discuss regularized CCE (Juodis, 2022,
Journal of Applied Econometrics). CCE is known to be
sensitive to the selection of the number of cross-section
averages. rCCE overcomes the problem by regularizing the
cross-section averages. Second, I will discuss the test for the
rank condition based on DeVos, Everaert, and Sarafidis (2024,
Econometrics Reviews). If the rank condition fails, CCE
will be inconsistent, and therefore testing the condition is key
for any empirical application. Finally, I will discuss the
selection of cross-section averages using the information
criteria from Karabiyik, Urbain, and Westerlund (2019, Journal of
Applied Econometrics) and Margaritella and Westerlund (2023,
Econometrics Journal).
Additional information:
Jan Ditzen
Freie Universität Bozen-Bolzano
|
9:30–9:55 | The SCCS design
Abstract:
The SCCS design, in contrast to standard epidemiological
observational designs like the cohort and case–control
design, offers a more time- and cost-efficient approach. This
efficiency is due to the larger sample sizes required by the
standard designs. Further, the SCCS method automatically adjusts
for known and unknown fixed confounders. The latter can be a
significant challenge in standard designs. The SCCS method
splits an observation period into one or more risk periods and
one or more control periods. The risk periods are relative to an
exposure event, whereas the observation period is either fixed
or relative to the exposure event. Often, one adds time or age
adjustments during the observation period. The basic idea is to
compare incidence rates for the risk periods with the control
period while adjusting for time or age and cases. The SCCS
design originates from the desire to estimate the relative
effect of vaccines, such as the MMR, on adverse events like
meningitis. Compared with the classical design, it is a matter of
asking when instead of who. I will discuss the SCCS design and
present the Stata command sccsdta, which transforms
datasets of times for events and exposures by cases into
datasets marked into risk and control periods as well as time or
age periods. After the dataset transformation, the analysis is
simple, using fixed-effect Poisson regression.
Additional information:
Niels Henrik Bruun
Aalborg University Hospital
|
9:55–10:20 | Improving the speed and accuracy when fitting flexible parametric survival models on the log-hazard scale
Abstract:
Flexible parametric survival models are an alternative to the
Cox proportional hazards model and more standard parametric
models for the modeling of survival (time-to-event) data. They
are flexible in that spline functions are used to model the
baseline and potentially complex time-dependent effects. In this
talk, I will discuss using splines on the log-hazard scale.
Models on this scale have some computational challenges because
numerical integration is required to integrate the hazard function
during estimation. The numerical integration is required for all
individuals and for each call to likelihood/gradient/Hessian
functions and can therefore be slow in large datasets. In
addition, the models may have a singularity for the hazard
function at t=0, which leads to precision issues. I will
describe two recent updates to the stpm3 command that
make these models faster to fit in large datasets and have
improved accuracy for the numerical integration. First, the
python option makes use of the mlad optimizer, which
calls python, leading to major speed gains in large datasets.
Second, there are different options for numerical integration of
the hazard function, including tanh-sinh quadrature, which is now
the default when the hazard function has a singularity at
t=0. This leads to more accurate estimates compared with
the more standard Gauss–Legendre quadrature. These speed and
accuracy improvements make the use of these models more feasible
in large datasets.
Additional information:
Paul Lambert
Cancer Registry of Norway–Norwegian Institute of Public Health, and Karolinska Institutet
|
10:35–10:50 | Example of modeling survival with registry data to assist with clinical decision making
Abstract:
The Cancer Registry of Norway contains several clinical
registries with rich information on the diagnosis, treatment, and
follow up of cancer patients. Since 2013, the Clinical Registry
for Gynecological Cancer has collected information on residual
disease (RD) diameter following ovarian cancer surgery, which is
prognostic for survival. Internationally, attaining 1cm or less
RD is considered “adequate” debulking. This cutoff has been
widely used for making treatment decisions and is used to define
high-risk patients in Norwegian treatment guidelines.
However, few studies have evaluated ovarian cancer survival across continuous RD diameter. In flexible parametric models, I compared excess mortality of stage III–IV ovarian cancer patients across continuous RD diameter using restricted cubic splines. This presentation is an example of using survival analyses on epidemiological data to assist with clinical decision making.
Additional information:
Cassie Trewin-Nybråten
Cancer Registry of Norway–Norwegian Institute of Public Health
|
10:50–11:05 | Limitations and comparison of the DFA, PP, and KPSS unit-root tests: Evidence for laboral market variables in Mexico
Abstract:
Unit-root tests have represented a great contribution to
time-series analysis by detecting when a variable is stationary or
not. However, they present limitations, which, although known,
are still used, and it seems that these limitations go unnoticed
when applied in time-series studies. Examples of these
limitations, mainly Dickey–Fuller (DF) and Phillips–Perron (PP),
are that they could be detecting the presence of a unit root
when the series does not have it. Consequently, this
presentation includes some of the criticisms that have been made
to the unit-root tests to consequently execute in Stata the
three best-known unit root tests (DFA, PP, and KPSS) for the main
macroeconomic variables of Mexico, this with the intention of
analyzing, both graphically and technically, whether the series
are stationary or not. The main conclusion is that unit-root
tests are often more related to statistical than economic
issues.
Additional information:
Ricardo Rodolfo
The National Autonomous University of Mexico
|
11:05–11:20 | Using Stata with many datasets, methods, and variables
Abstract:
Complex data management and extensive analysis of data can be
challenging in research projects. Compared with a classical
textbook example with one clean dataset and a few selected
variables and models, medical research projects often involve
many datasets in different formats and use a range of
statistical methods and many variables and
outcomes. Stata has features for keeping track of datasets,
automating statistical analyses, and summarizing results. Some
experiences and practical tips with commands such as
import, foreach, putexcel, and
dtable in combination with the use of macros will be
presented. These can be helpful for efficiently solving complex
tasks, obtaining overviews of data and methods, and reporting
statistical results to a multidisciplinary research group.
Additional information:
Are Hugo Pripp
Oslo Centre for Biostatistics and Epidemiology (OCBE)
|
11:20–12:20 | Maps in Stata
Abstract:
This interactive talk will provide an introduction to the
packages and code required for producing high-quality maps in
Stata. I will show how to import shapefiles, plot different
layer types (points, lines, polygons), and generate different
types of choropleth and bivariate maps. Some basic customization
options will also be discussed.
Additional information:
Asjad Naqvi
Austrian Institute for Economic Research (WIFO) and Vienna University of Economics and Business (WI)
|
1:00–2:00 | Causal inference with time-to-event outcomes under competing risk
Abstract:
The occurrence of competing events often complicate the analysis
of time-to-event outcomes. While there is a rich literature in
the area of survival analysis on methods for handling competing
risk that goes back a long way, there has also for a long time been
some confusion regarding best approach and implementation when
facing competing events in applied research. Recent advances in
the use of estimands in causal inference has led to new
developments and insights (and discussions) on how to best
analyze time-to-event outcomes under competing risk. The role of
classical statistical estimands are now better understood, and
new causal estimands have been suggested for addressing more
advanced causal questions. In this talk, I will briefly review
this development and the estimation of the most basic estimands and
discuss some extensions, such as when interest is in the effect
of time-varying treatments.
Additional information:
Jon Michael Gran
Oslo Centre for Biostatistics and Epidemiology (OCBE)
|
2:10–2:30 | Extending standard reporting to improve communication of survival statistics
Abstract:
Routine reporting of cancer patient survival is important, both
to monitor the effectiveness of healthcare and to inform about
prognosis following a cancer diagnosis. A range of different
survival measures exist, each serving different purposes and
targeting different audiences. It is important that routine
publications expand on current practice and provide estimates on
a wider range of survival measures. Using data from The Cancer
Registry of Norway, we examine the feasibility of automated
production of such statistics.
Additional information:
Tor Åge Myklebust
Cancer Registry of Norway–Norwegian Institute of Public Health
|
2:30–3:10 | Balancing the privacy-utility trade-off for synthetic time-to-event data
Abstract:
Introduction: Generation of synthetic patient records can
preserve the structure and statistical properties of the
original data while maintaining privacy, providing access to
high-quality data for research and innovation. Few
synthesization methods account for the censoring mechanisms in
time-to-event data, and formal privacy evaluations are often
lacking. Improvements in synthetic data utility come with
increased risks of privacy disclosure, necessitating a careful
evaluation to obtain the proper balance.
Methods: We generate synthetic time-to-event data based on colon cancer data from the Cancer Registry of Norway, using a sequence of conditional regression models and flexible parametric modeling of event times. Different levels of model complexity are used to investigate the impact on data utility and disclosure risk. The privacy risk is evaluated using Bayesian estimation of disclosure risks, which form the basis for a differential privacy audit. Results: Including more interaction terms and increasing degrees of freedom improves synthetic data utility and elevates privacy risks. While certain interactions substantially improve utility, others reduce privacy without much utility gain. The most complex model displays near-optimal utility scores. Conclusions: The results demonstrated a clear tradeoff between synthetic data utility and privacy risks. Interestingly, the relationship is nonlinear, because certain modeling choices increase synthetic data utility with little privacy loss, and vice versa.
Additional information:
Sigrid Leithe
Cancer Registry of Norway–Norwegian Institute of Public Health
|
3:20–3:45 | How can Stata enable federated computing for decentralized data analysis?
Abstract:
Federated computing offers a transformative approach to data
analysis, enabling the processing of distributed datasets
without the need for centralization, thus aiming to preserve
privacy and security. In this talk, I will explore how these
principles can be applied within the Stata environment to
address the growing challenges of data sharing and computational
limits. I will highlight the current features in Stata that make
federated computing possible and the challenges and future
directions, setting the stage for innovation in decentralized
data analysis. By integrating federated computing with Stata,
researchers can perform complex analyses on sensitive,
geographically dispersed data while maintaining the software's
robust statistical capabilities.
Additional information:
Narasimha Raghavan
Cancer Registry of Norway–Norwegian Institute of Public Health
|
3:45–4:45 | Causal mediation
Abstract:
Causal inference aims to identify and quantify a causal effect.
With traditional causal inference methods, we can estimate the
overall effect of a treatment on an outcome. When we want to
better understand a causal effect, we can use causal mediation
analysis to decompose the effect into a direct effect of the
treatment on the outcome and an indirect effect through another
variable, the mediator. Causal mediation analysis can be
performed in many situations—the outcome and mediator
variables may be continuous, binary, or count, and the treatment
variable may be binary, multivalued, or continuous.
In this talk, I will introduce the framework for causal
mediation analysis and demonstrate how to perform this analysis
with the mediate command, which was introduced in Stata
18. Examples will include various combinations outcome,
mediator, and treatment types.
Additional information:
Kristin MacDonald
StataCorp LLC
|
5:00–5:25 | Multivariate random-effects meta-analysis for sparse data using smvmeta
Abstract:
Multivariate meta-analysis is used to synthesize estimates of
multiple quantities (“effect sizes”), such as risk
factors or treatment effects, accounting for correlation and
typically also heterogeneity. In the most general case,
estimation can be intractable if data are sparse (for example,
many risk factors but few studies) because the number of model
parameters that must be estimated scales quadratically with the
number of effect sizes. I will present a new meta-analysis model
and Stata command, smvmeta, that make estimation
tractable by modeling correlation and heterogeneity in a
low-dimensional space via random projection and that provide
more precise estimates than meta-regression (a reasonable
alternative model that could be used when data are sparse). I
will explain how to use smvmeta to analyze data from a
recent meta-analysis of 23 risk factors for pain after total
knee arthroplasty.
Additional information:
Chris Rose
Norwegian Institute of Public Health
|
5:25–5:50 | Advanced data visualizations with Stata, part VI: Visualizing more than two variables
Abstract:
The presentation will showcase how Stata can be utilized for
visualizing data with more than two dimensions. The
presentation will introduce extensions to existing visualization
packages and will also launch two new packages.
Additional information:
Asjad Naqvi
Austrian Institute for Economic Research (WIFO) and Vienna University of Economics and Business (WI)
|
5:50–6:15 | Open panel discussion with Stata developers
Contribute to the Stata community by sharing your feedback with StataCorp's developers. From feature improvements to bug fixes and new ways to analyze data, we want to hear how Stata can be made better for our users.
|
The 2024 Northern European Stata Conference is jointly organized by Metrika Consulting AB, the official distributor of Stata for Russia and the Nordic and Baltic countries, the Cancer Registry of Norway at the Norwegian Institute of Public Health, and Oslo Centre for Biostatistics and Epidemiology (University of Oslo and Oslo University Hospital).
View the proceedings of previous Stata Conferences and international meetings.