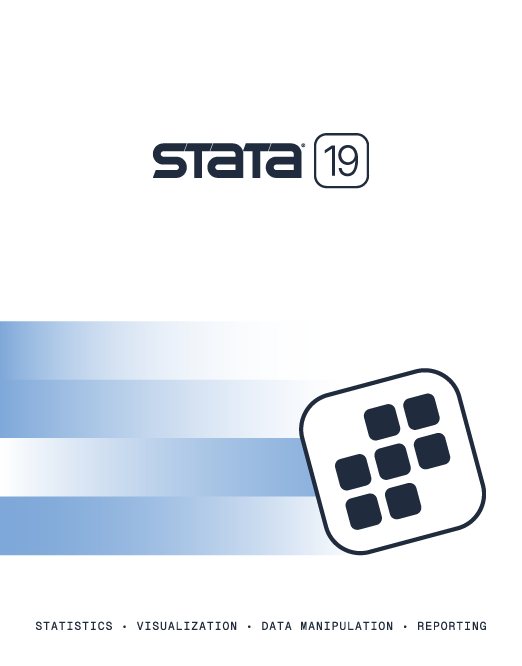
2025 Stata Conference • Nashville, TN • 31 July–01 August
Speakers: Adrian P. Mander, University Forvie Site, and David G. Clayton, Cambridge University |
Missing data can be a serious problem in many statistical analyses. The problems are manifested as a loss of efficiency or possible bias. If the data is Missing Completely At Random (MCAR) then analysis of the data using case deletion (ignoring the lines of data with any missing) will give unbiased answers but inflated confidence intervals. Missing At Random (MAR) can lead to bias if the data is analysed using case deletion. There are solutions using likelihood based approaches; however, these may rely on assumptions on the missing data process. Imputation is an alternative which can solve MCAR and MAR problems with relatively few assumptions. The function that will be discussed only imputes values for one variable in the dataset. The imputation method involves bootstrapping the observed data values and hence is called hotdeck imputation, a term first used by the survey practitioners. The method will be illustrated by using an example taken from a two-stage sample case-control study design. A comparison is made between the biased case deletion analysis and the imputed analysis.
Learn
Free webinars
NetCourses
Classroom and web training
Organizational training
Video tutorials
Third-party courses
Web resources
Teaching with Stata
© Copyright 1996–2025 StataCorp LLC. All rights reserved.
×
We use cookies to ensure that we give you the best experience on our website—to enhance site navigation, to analyze usage, and to assist in our marketing efforts. By continuing to use our site, you consent to the storing of cookies on your device and agree to delivery of content, including web fonts and JavaScript, from third party web services.
Cookie Settings
Last updated: 16 November 2022
StataCorp LLC (StataCorp) strives to provide our users with exceptional products and services. To do so, we must collect personal information from you. This information is necessary to conduct business with our existing and potential customers. We collect and use this information only where we may legally do so. This policy explains what personal information we collect, how we use it, and what rights you have to that information.
These cookies are essential for our website to function and do not store any personally identifiable information. These cookies cannot be disabled.
This website uses cookies to provide you with a better user experience. A cookie is a small piece of data our website stores on a site visitor's hard drive and accesses each time you visit so we can improve your access to our site, better understand how you use our site, and serve you content that may be of interest to you. For instance, we store a cookie when you log in to our shopping cart so that we can maintain your shopping cart should you not complete checkout. These cookies do not directly store your personal information, but they do support the ability to uniquely identify your internet browser and device.
Please note: Clearing your browser cookies at any time will undo preferences saved here. The option selected here will apply only to the device you are currently using.