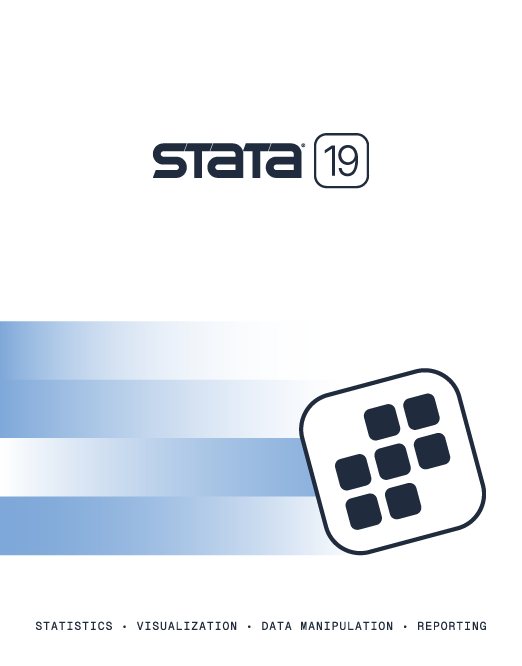
Title | Taylor linearization approach | |
Author | Roberto Gutierrez, StataCorp | |
Date | October 2001 |
The documentation states that the program only needs to know about the first stage of sampling (i.e., the primary sampling units) to use Taylor linearization approach, and that the results will be conservative. How conservative will this approach be?
The "conservativeness" of the standard errors obtained from linearization methods which only use information concerning the first stage of sampling is hard to quantify, since it depends on the specific problem. However, as a general rule the more complicated the sampling scheme becomes in the second and subsequent sampling stages, the closer the linearization standard errors will approach their more complicated counterparts which exploit the information contained in the sampling at the later stages.
Let's consider an example which I hope will illustrate this point and also serve as something I can refer to when answering your later questions below:
Suppose we have a two-stage stratified sample design, where the strata are states (Texas, California, and Florida). The first level PSU's are school districts with a certain number selected from each state. Each district will contain a certain number of schools from which the second stage of sampling is merely a simple random sample of schools within each district, within each stratum.
Let's examine how linearization is conservative here. By only specifying the first level PSU's (districts) we are basically allowing for the fact that schools within a given district are correlated, or said better, our variance estimator has to allow for the fact that two schools in the same district share the same fate, i.e. school A will be in our sample IF AND ONLY IF school B is in our sample, provided school A and school B are in the same district, call it District 105 in the state of Texas, say.
If the actual sampling scheme only consisted of a stratified random sample of districts from each state, then our model is correct and as efficient can possibly be without making further model assumptions about the correlation of schools within district (but that is not a question of sampling design and thus besides the point). Given the one-stage sampling scheme, schools A and B do share the same fate: they are either both in the sample, or are both not.
Now what happens when we add the second stage of sampling, i.e. we will now take a random sample of, say, half the schools in each district. Do schools A and B in District 105 in Texas still share the same fate? Well, yes and no. If we fail to pick district 105, then yes, they both have zero probability of being in our final sample. But what happens if we do pick district 105? Then the answer is no. Given that we pick district 105, then whether school A is in our sample is now INDEPENDENT of whether school B is in out sample, and all of a sudden schools A and B don't look as dependent as they once did.
Thus linearization, by estimating the variance in such a way which allows A and B to be dependent is still correct, but is giving away too much, i.e., it is being too conservative. There is indeed dependence (if we do not pick district 105, then the fates of school's A and B are still tied together), but once the first stage is completed and district 105 is picked, the dependence goes away. Alas, linearization methods are not sophisticated enough to take back the precision to be gained from conditional independence that may be present in latter-stage sampling, and this is where more specialized methods can come to the rescue.
Now let's take this same example and instead make the second stage sampling more complicated. Instead of a simple random sample of half the schools in each district, let's take a cluster sample of towns (which each contain several schools) within each district. In this case, if schools A and B are in same town then there fates are identical no matter whether district 105 is picked in the first stage. If schools A and B are not in the same town, then we are back to the case where schools A and B may be conditionally independent, where the "condition" is whether district 105 is picked. If district 105 is not picked, schools A and B both have probability zero of being in our sample. If district 105 is picked, then the fates of A and B are independent only if they are in different towns. Linearization is still being conservative by assuming dependence of all schools in district 105, but we are already starting to see that there is less conditional independence to be exploited by the more complex multi-stage variance estimator.
In general, we can thus see that as the second- and latter-stage sampling schemes become more complicated, the loss in efficiency by going the conservative linearization route diminishes, since the amount of conditional independence that can be exploited decreases.
My current plans for the design are as follows. First, we will select a stratified random sample of districts without replacement. The sampling fractions will vary from stratum to stratum. Second, we will identify all of the schools in need of improvement in those districts, and divide them into strata, and determine how many I want from each of these school strata. From each of these strata, I will select a sample of schools in need of improvement, without replacement, with probability inversely proportional to the probability of selection of the district. This should equalize the weights for the schools within each school stratum.
I don't know whether the Stata package ever considered this kind of design. The usual multi-stage approach uses constant sampling fractions (or constant sample size) within PSU. We would also need to assure ourselves that the statement of conservativeness continues to hold for this design.
The statement of conservativeness does continue to hold, and given the complicated nature of the second stage sampling (i.e. re-stratification), becomes less of something that we need to worry about. For the above design which you describe, you have your strata, districts are the PSU's, and as long as you can calculate the observation weights for each school in your sample to be the inverse of the probability of ultimately making it in (which seems fairly straight-forward), then Stata will do fine here. And yes, sampling weights may differ within PSU's in Stata.
I also have a third question. In addition to using linearization methods, they are planning on developing a set of replication weights to go with the data. I believe that it would be quite easy to develop a program for Stata to employ those weights to generate standard errors for an arbitrary statistic from an estimation command, simply by running the command once with each set of the K weights (specified as pweights), and no other survey design features specified, and then calculatingse(theta) = sum (theta_i - theta_bar) / Kwhere theta_i is the estimated theta using the i'th set of weights, theta_bar is the estimate of theta using all the data (again with pweights but no other survey design features), and K is the number of weights. [Weighted by 1/(1-f)^2 if Fay's adjustment was used in calculating the weights.] I'm working from Korn & Graubard (1999), Analysis of Health Surveys here, page 34. Am I missing something more complicated about this?
I just took a look at Korn & Graubard, and agree with your assessment. It is indeed that simple.