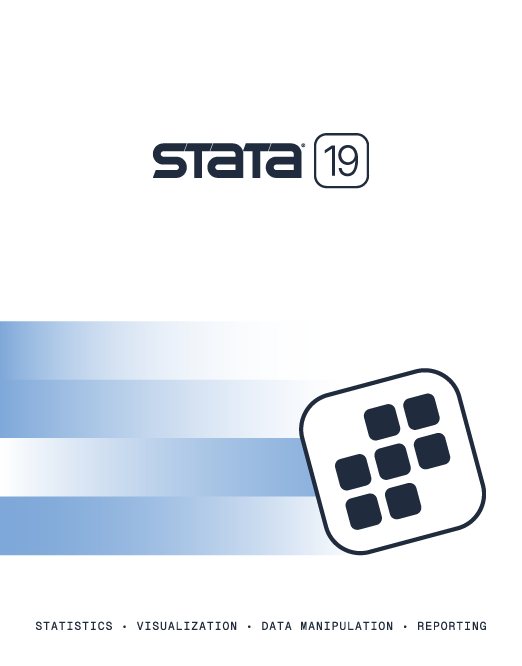
9:00–10:00 | Drivers of COVID-19 deaths in the United States: A two-stage modeling approach
Abstract:
We offer a two-stage (time-series and cross-section) econometric
modeling approach to examine the drivers behind the spread of
COVID-19 deaths across counties in the United States.
Our empirical strategy exploits the availability of two years
(January 2020 through January 2022) of daily data on the number
of confirmed deaths and cases of COVID-19 in the 3,000 U.S.
counties of the 48 contiguous states and the District of
Columbia.
In the first stage of the analysis, we use daily time-series data on COVID-19 cases and deaths to fit mixed models of deaths against lagged confirmed cases for each county. Because the resulting coefficients are county specific, they relax the homogeneity assumption that is implicit when the analysis is performed using geographically aggregated cross-section units. In the second stage of the analysis, we assume that these county estimates are functions of economic and sociodemographic factors that are taken as fixed over the course of the pandemic. Here we employ the novel one-covariate-at-a-time variable-selection algorithm proposed by Chudik et al. (2018) to guide the choice of regressors.
Contributors:
Andrés Garcia-Suaza
Universidad del Rosario
Miguel Henry
Greylock McKinnon Associates
Jesús Otero
Universidad del Rosario
Additional information:
Christopher F. Baum
Boston College
|
10:00–10:30 | Discrete-time multistate regression models in Stata
Abstract:
Multistate life tables (MSLTs), or multistate survival models,
have become a widely used analytical framework among
epidemiologists, social scientists, and demographers.
MSLTs can be cast in continuous time or discrete time. While the
choice between the two approaches depends on the concrete
research question and available data, discrete-time models have
several appealing features: they are easy to apply; the
computational cost is typically low; and today's empirical
studies are frequently based on regularly spaced longitudinal
data, which naturally suggests modeling in discrete time.
Despite these appealing features, Stata community-contributed packages have so far been developed only for continuous-time models (Crowther and Lambert 2017; Metzger and Jones 2018) or for traditional demographic life-table calculations that do not allow for covariate adjustment (Muniz 2020). This presentation introduces the recently published Stata package dtms, which seeks to fill the gap in software availability for discrete-time multistate model estimation. The dtms package provides a well-documented and easy-to-apply set of commands that cover a large set of discrete-time MSLT techniques that currently exist in the literature. It also features inference based on newly derived asymptotic covariance matrices as well as inference on group contrasts. References:Crowther, M. J., and P. C. Lambert. 2017. Parametric multistate survival models: Flexible modelling allowing transition-specific distributions with application to estimating clinically useful measures of effect differences. Statistics in Medicine 36: 4719–4742. https://doi.org/10.1002/sim.7448 Metzger, S. K., B. T. Jones. 2018. mstatecox: A package for simulating transition probabilities from semiparametric multistate survival models. The Stata Journal 18: 533–563. Muniz, J. O. 2020. Multistate life tables using Stata. The Stata Journal 20: 721–45. doi: 10.1177/1536867X20953577.
Additional information:
Daniel C. Schneider
MPI for Demographic Research
|
10:30–10:45 | mfcurve: Visualizing results from multifactorial designs
Abstract:
Multifactorial designs are used to study the (joint) impact of
two or more factors on an outcome.
They typically occur in conjoint, choice, and factorial survey
experiments but have recently gained increasing popularity in
field experiments, too. Technically, they allow researchers to
investigate moderation as an instance of treatment heterogeneity
by crossing multiple treatments.
Naturally, multifactorial designs quickly spawn a spiraling number of distinct treatment combinations: even a moderately complex design of two factors with three levels each yields 32 unique combinations. For more elaborate setups, full factorials can easily produce dozens of distinct combinations, rendering the visualization of results difficult. This presentation introduces the new Stata command mfcurve as a potential remedy. Mimicking the appearance of a specification curve, mfcurve produces a two-part chart: the graph’s upper panel displays average effects for all distinct treatment combinations; its lower panel indicates the presence or absence of any level given the respective treatment condition. Unlike existing visualization techniques, this enables researchers to plot and inspect results from multifactorial designs much more comprehensively. Highlighting potential applications, the presentation will demonstrate mfcurve’s most important features and options, which currently include replacing point estimates by box plots and testing results for statistical significance.
Additional information:
Daniel Krähmer
Ludwig-Maximilians-University
|
11:15–11:45 | Estimating the price elasticity of gasoline demand in correlated random coefficient models with endogeneity
Abstract:
We propose a per-cluster instrumental-variables approach (PCIV)
for estimating correlated random coefficient models in the
presence of contemporaneous endogeneity and two-way fixed
effects.
We use variation across clusters to estimate coefficients with
homogeneous slopes (such as time effects) and within-cluster
variation to estimate the cluster-specific heterogeneity
directly. We then aggregate them to population averages. We
demonstrate consistency, showing robustness over standard
estimators, and provide analytic standard errors for robust
inference. Basic implementation is straightforward using
standard software such as Stata.
In Monte Carlo simulation, PCIV performs relatively well against pooled 2SLS and fixed-effects IV (FEIV) with a finite number of clusters or finite observations per cluster. We apply PCIV in estimating the price elasticity of gasoline demand using state fuel taxes as instrumental variables. PCIV estimation allows for greater transparency of the underlying data. In our setting, we provide evidence of correlation between heterogeneity in the first and second stages, violating a key assumption underpinning consistency of standard estimators. We see significant divergence in the implicit weighting when applying FEIV from the natural weights applied in PCIV. Overlooking effect heterogeneity with standard estimators is consequential. Our estimated distribution of elasticities reveals significant heterogeneity and meaningful differences in estimated averages.
Contributor:
Seolah Kim
University of California
Additional information:
Michael Bates
University of California
|
11:45–12:15 | Influence analysis with panel data using Stata
Abstract:
The presence of anomalous cases in a dataset (for example,
vertical outliers, good and bad leverage points) can severely
affect least-squares estimates (coefficients or standard
errors) that are sensitive to extreme cases by construction.
Cook (1979)’s distance is usually used to detect such
anomalies in cross-sectional data. This metric may fail to
flag multiple atypical cases (Atkinson 1985; Chatterjee and
Hadi 1988; Rousseeuw and Van Zomeren 1990), while a local
approach overcomes this limit (Lawrance 1995).
I formalize statistical measures to quantify the degree of leverage and outlyingness of units in a panel-data framework. I hence develop a unitwise method to visually detect the type of anomaly, quantify its joint and conditional influence, and quantify the direction of the enhancing and masking effects. I conduct the proposed influence analysis using two community-contributed commands. First, xtinfluence calculates the joint and conditional influence of unit i on unit j and the relative enhancing and masking effects. A two-way scatter plot or the SSC heatplot can be used to visualize the influence exerted by each unit in the sample. Second, xtlvr2plot (a panel-data version for lvr2plot) produces unitwise plots displaying the average individual influence and the average normalized squared residual of unit i. References: Atkinson, A. C. 1985. Plots, transformations and regression; an introduction to graphical methods of diagnostic regression analysis. Technical report. Chatterjee, S., and A. S. Hadi. 1988. Impact of simultaneous omission of a variable and an observation on a linear regression equation. Computational Statistics & Data Analysis, 6: 129–144. Cook, R. D. 1979. Influential observations in linear regression. Journal of the American Statistical Association, 74: 169–174. Lawrance, A. 1995. Deletion influence and masking in regression. Journal of the Royal Statistical Society: Series B (Methodological), 57: 181–189. Rousseeuw, P. J., and B. C. Van Zomeren. 1990. Unmasking multivariate outliers and leverage points. Journal of the American Statistical association, 85: 633–639.
Additional information:
Annalivia Polselli
Essex University
|
12:15–12:45 | nopo: An implementation of a matching-based decomposition technique with postestimation commands
Abstract:
Ñopo (2008) proposed a nonparametric decomposition
technique based on matching, which decomposes the observed gap
in an outcome between groups into four components.
Among the matched sample, the explained component is the
part of the gap attributed to compositional differences between
groups in predictors of the outcome, and the unexplained
component is the part of the gap that would remain if these
compositional differences were eliminated. Two additional
components capture how unmatched individuals in group A and
group B contribute to the gap in the outcome.
Ñopo’s technique directly addresses the issue of
lacking common support between groups that can bias
linear-regression-based decompositions, exhibits a general
robustness against functional-form misspecification, and allows
the evaluation of gaps over the full distribution of the outcome.
However, high dimensionality means that there is always a tradeoff between the detail of the matching set (to achieve balance between groups) and common support (the share of matches), particularly in small samples. Extending the community-contributed Stata command nopomatch (Atal et al, 2010.), our command nopo provides a comprehensive implementation of Ñopo’s matching, including different matching procedures. Postestimation commands investigate the balance after matching, explore the lack of common support, and visualize the unexplained component over the outcome distribution. We highlight the merit of this approach and our command by comparing matching with regression-based techniques using a simulation and observational data. References:Ñopo, H. 2008. Matching as a tool to decompose wage gaps. The Review of Economics and Statistics 90: 290–299. Atal, J. P., A. Hoyos, and H. Ñopo. 2010. NOPOMATCH: Stata module to implement Nopo's decomposition. Statistical Software Components S457157, Boston College Department of Economics.
Contributor:
Maximilian Sprengholz
Humboldt University of Berlin
Additional information:
Maik Hamjediers
Humboldt University of Berlin
|
1:45–2:45 | Linking frames in Stata
Additional information:
Jeff Pitblado
StataCorp
|
2:45–3:45 | Causal inference and treatment-effect decomposition with Stata
Additional information:
Joerg Luedicke
StataCorp
|
4:15–4:45 | lgrgtest: Lagrange multiplier test after constrained maximum-likelihood estimation using Stata
Abstract:
Besides the Wald and the likelihood-ratio test, the
Lagrange multiplier test (Rao 1948; Aitchison and Silvey 1958;
Silvey, 1959)—also known as the score test—is the third
canonical approach to testing hypotheses after
maximum likelihood estimation.
While the Stata commands test and lrtest implement
the former two, real Stata does not have a general command
for implementing the latter. This presentation introduces the
new community-contributed Stata postestimation command
lgrgtest that allows for straightforwardly using
Lagrange multiplier test after constrained maximum-likelihood
estimation.
lgrgtest is intended to be compatible with all Stata estimation commands that use maximum likelihood and allow for the options constraints(), iterate(), and from() and obey Stata's standards for the syntax of estimation commands. lgrgtest can also be used after cnsreg. lgrgtest draws on Stata’s constraint command and the accompanying option constraints(), which only allows for imposing linear restrictions on a model. This results in the limitation of lgrgtest being confined to testing linear constraints only. A (partial) replication of Egger et al. (2011) illustrates the use of lgrgtest in applied empirical work. References: Aitchison, J., and S. D. Silvey. 1958. Maximum-likelihood estimation of parameters subject to restraints. The Annals of Mathematical Statistics 29: 813–828. Egger, P., M. Larch, K. E. Staub, and R. Winkelmann. 2011. The trade effects of endogenous preferential trade agreements. American Economic Journal: Economic Policy 3: 113–43. Rao, C. R. 1948. Large sample tests of statistical hypotheses concerning several parameters with applications to problems of estimation. Mathematical Proceedings of the Cambridge Philosophical Society 44: 50–57. Silvey, S. D. 1959: The Lagrangian multiplier test. The Annals of Mathematical Statistics 30: 389–407.
Additional information:
Harald Tauchmann
FAU Erlangen-Nürnberg
|
5:00–5:30 | Power boost or source of bias? Monte Carlo evidence on ML covariate adjustment in randomized trials in education
Abstract:
Statistical theory makes ambiguous predictions about covariate
adjustment in randomized trials.
While proponents highlight possible efficiency gains, opponents
point to possible finite-sample bias, a loss of precision in
the case of many and weak covariates, and as the increasing
danger of false-positive results due to repeated model
specification. This theoretical reasoning suggests that
machine learning (variable selection) methods may be promising
tools to keep the advantages of covariate adjustment,
while simultaneously protecting against its downsides.
In this presentation, I rely on recent developments of machine learning methods for causal effects and their implementation in Stata to assess the performance of ML methods in randomized trials. I rely on real-world data and simulate treatment effects on a wide range of different data structures, including different outcomes and sample sizes. (Preliminary) results suggests that ML adjusted estimates are unbiased and show considerable efficiency gains compared with unadjusted analysis. The results are fairly similar between different data structures used and robust to the choice of tuning parameters of the ML estimators. These results tend to support the more optimistic view on covariate adjustment and highlight the potential of ML methods in this field.
Additional information:
Lukas Fervers
University of Cologne and Leibniz-Centre for Life-Long Learning
|
5:30–6:00 | Open panel discussion with Stata developers
Contribute to the Stata community by sharing your feedback with StataCorp's developers. From feature improvements to bug fixes and new ways to analyze data, we want to hear how Stata can be made better for our users.
|
The workshop introduces how to use Python from within Stata and how to use Stata from within Python.
The logistics organizer for the 2023 German Stata Conference is DPC Software GmbH, the official distributor of Stata in Germany, the Netherlands, Austria, the Czech Republic, and Hungary.
View the proceedings of previous Stata Conferences and Users Group meetings.