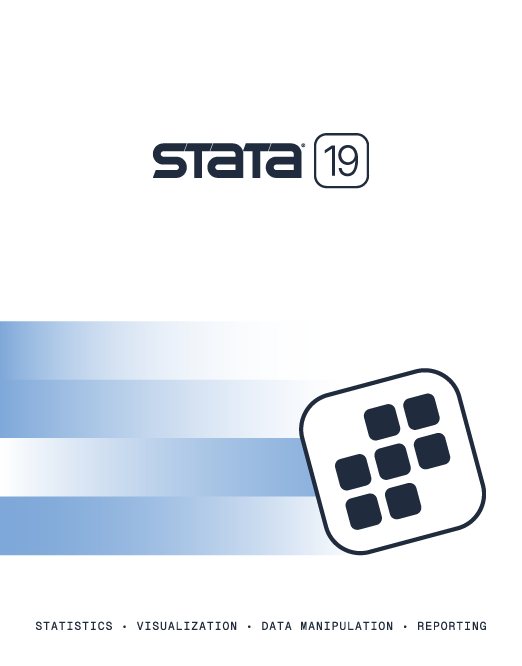
2025 Stata Conference • Nashville, TN • 31 July–01 August
Econometric Evaluation of Socio-Economic Programs, Second Edition |
||||||||||||||||||||||||||||||||||||
![]() Click to enlarge See the back cover |
![]()
As an Amazon Associate, StataCorp earns a small referral credit from
qualifying purchases made from affiliate links on our site.
eBook not available for this title
eBook not available for this title |
|
||||||||||||||||||||||||||||||||||
Comment from the Stata technical groupEconometric Evaluation of Socio-Economic Programs by Giovanni Cerulli, is an excellent introduction to estimating treatment effects from observational data. This book provides readers with the theoretical and practical knowledge required to design rigorous and precise program evaluations. The statistical models that stem from each evaluation technique are thoroughly discussed and followed by empirical examples illustrating their implementation in Stata. The wide array of models covered can be fit with the commands teffects, etregress, etpoisson, eteffects, didregress, and hdidregress. They can also be fit with the community-contributed commands treatrew and ivtreatreg. The book first overviews the econometrics of program evaluation and the concept of treatment effect. It then shows how to estimate treatment effects under the assumption of conditional independence between treatment and potential outcomes. Methods that drop this assumption are presented next, thereby allowing for endogenous treatment effects. The following chapters cover more advanced topics, including local average treatment effects and regression discontinuity designs. The second edition features two new chapters on advanced difference-in-differences methods and synthetic control methods. The author provides a nice mix of intuition, mathematics, and Stata examples. Professors, graduate students, practitioners, and researchers will find this book useful in the classroom and for self-study in preparation for research projects. |
||||||||||||||||||||||||||||||||||||
Table of contentsView table of contents >> 1 An Introduction to the Econometrics of Program Evaluation
1.1 Introduction
1.2 Statistical Setup, Notation, and Assumptions
1.2.1 Identification Under Random Assignment
1.3 Selection on Observables and Selection on Unobservables
1.2.2 A Bayesian Interpretation of ATE Under Randomization 1.2.3 Consequences of Nonrandom Assignment and Selection Bias
1.3.1 Selection on Observables (or Overt Bias) and Conditional Independence Assumption
1.4 Characterizing Selection Bias
1.3.2 Selection on Unobservables (or Hidden Bias) 1.3.3 The Overlap Assumption
1.4.1 Decomposing Selection Bias
1.5 The Rationale for Choosing the Variables to Control for 1.6 Partial Identification of ATEs: The Bounding Approach 1.7 A Guiding Taxonomy of the Econometric Methods for Program Evaluation 1.8 Policy Framework and the Statistical Design for Counterfactual Evaluation 1.9 Available Econometric Software 1.10 A Brief Outline of the Book References 2 Methods Based on Selection on Observables
2.1 Introduction
2.2 Regression-Adjustment
2.2.1 Regression-Adjustment as Unifying Approach Under Observable Selection
2.3 Matching
2.2.2 Linear Parametric Regression-Adjustment: The Control-Function Regression 2.2.3 Nonlinear Parametric Regression-Adjustment 2.2.4 Nonparametric and Semi-parametric Regression-Adjustment
2.3.1 Covariates and Propensity-Score Matching
2.4 Reweighting
2.3.2 Identification of ATEs Under Matching 2.3.3 Large Sample Properties of Matching Estimator(s) 2.3.4 Common Support 2.3.5 Exact Matching and the "Dimensionality Problem" 2.3.6 The Properties of the Propensity-Score 2.3.7 Quasi-exact Matching Using the Propensity-Score 2.3.8 Methods for Propensity-Score Matching 2.3.9 Inference for Matching Methods 2.3.10 Assessing the Reliability of CMI by Sensitivity Analysis 2.3.11 Assessing Overlap 2.3.12 Coarsened-Exact Matching
2.4.1 Reweighting and Weighted Least Squares
2.5 Doubly-Robust Estimation 2.4.2 Reweighting on the Propensity-Score Inverse-Probability 2.4.3 Sample Estimation and Standard Errors for ATEs 2.6 Implementation and Application of Regression-Adjustment 2.7 Implementation and Application of Matching
2.7.1 Covariates Matching
2.8 Implementation and Application of Reweighting
2.7.2 Propensity-Score Matching 2.7.3 An Example of Coarsened-Exact Matching Using cem
2.8.1 The Stata Routine treatrew
References
2.8.2 The Relation Between treatrew and Stata 13 teffects ipw 2.8.3 An Application of the Doubly-Robust Estimator 3 Methods Based on Selection on Unobservables
3.1 Introduction
3.2 Instrumental-Variables
3.2.1 IV Solution to Hidden Bias
3.3 Selection-Model
3.2.2 IV Estimation of ATEs 3.2.3 IV with Observable and Unobservable Heterogeneities 3.2.4 Problems with IV Estimation
3.3.1 Characterizing OLS Bias within a Selection-Model
3.4 Difference-in-Differences
3.3.2 A Technical Exposition of the Selection-Model 3.3.3 Selection-Model with a Binary Outcome
3.4.1 DID with Repeated Cross Sections
3.5 Implementation and Application of IV and Selection-Model
3.4.2 DID with Panel Data 3.4.3 DID with Matching
3.5.1 The Stata Command ivtreatreg
3.6 Implementation and Application of DID
3.5.2 A Monte Carlo Experiment 3.5.3 An Application to Determine the Effect of Education on Fertility 3.5.4 Applying the Selection-Model Using etregress
3.6.1 DID with Repeated Cross Sections
References
3.6.2 DID Application with Panel Data 4 Local Average Treatment Effect and Regression-Discontinuity-Design
4.1 Introduction
4.2 Local Average Treatment Effect
4.2.1 Randomization Under Imperfect Compliance
4.3 Regression-Discontinuity-Design
4.2.2 Wald Estimator and LATE 4.2.3 LATE Estimation 4.2.4 Estimating Average Response for Compliers 4.2.5 Characterizing Compliers 4.2.6 LATE with Multiple Instruments and Multiple Treatment
4.3.1 Sharp RDD
4.4 Application and Implementation
4.3.2 Fuzzy RDD 4.3.3 The Choice of the Bandwidth and Polynomial Order 4.3.4 Accounting for Additional Covariates 4.3.5 Testing RDD Reliability 4.3.6 A Protocol for Practical Implementation of RDD
4.4.1 An Application of LATE
References
4.4.2 An Application of RDD by Simulation 4.4.3 An Application of RDD to Real Data 5 Difference-in-Differences with Many Pre- and Post-Treatment Times
5.1 Introduction
5.2 The TVDIFF Model
5.2.1 Testing the "Common Trend" Assumption
5.3 The TFDIFF Model
5.2.2 An Application of the TVDIFF Model
5.3.1 Generalization to More Than Three Times
5.4 Parallel-Trend Test in the Presence of Effect Anticipation 5.3.2 Testing the Parallel-Trend Assumption 5.3.3 An Application of the TFDIFF Model 5.5 Conclusion References 6 Synthetic Control Method
6.1 Introduction
6.2 The SCM Model 6.3 SCM Inference 6.4 Application 6.5 Conclusions References |
Learn
Free webinars
NetCourses
Classroom and web training
Organizational training
Video tutorials
Third-party courses
Web resources
Teaching with Stata
© Copyright 1996–2025 StataCorp LLC. All rights reserved.
×
We use cookies to ensure that we give you the best experience on our website—to enhance site navigation, to analyze usage, and to assist in our marketing efforts. By continuing to use our site, you consent to the storing of cookies on your device and agree to delivery of content, including web fonts and JavaScript, from third party web services.
Cookie Settings
Last updated: 16 November 2022
StataCorp LLC (StataCorp) strives to provide our users with exceptional products and services. To do so, we must collect personal information from you. This information is necessary to conduct business with our existing and potential customers. We collect and use this information only where we may legally do so. This policy explains what personal information we collect, how we use it, and what rights you have to that information.
These cookies are essential for our website to function and do not store any personally identifiable information. These cookies cannot be disabled.
This website uses cookies to provide you with a better user experience. A cookie is a small piece of data our website stores on a site visitor's hard drive and accesses each time you visit so we can improve your access to our site, better understand how you use our site, and serve you content that may be of interest to you. For instance, we store a cookie when you log in to our shopping cart so that we can maintain your shopping cart should you not complete checkout. These cookies do not directly store your personal information, but they do support the ability to uniquely identify your internet browser and device.
Please note: Clearing your browser cookies at any time will undo preferences saved here. The option selected here will apply only to the device you are currently using.