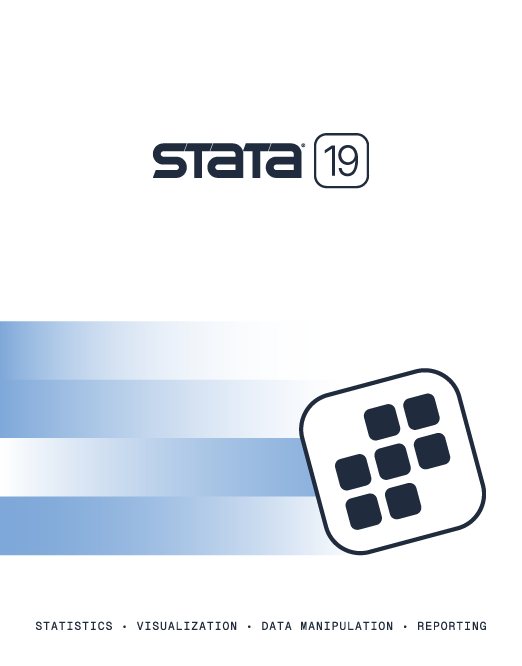
Stata 19 is here—explore all the features.
Discovering Structural Equation Modeling Using Stata |
||||||||||||||||||||||||||||||||||||
![]() Click to enlarge See the back cover |
eBook not available for this title
eBook not available for this title --> |
|
||||||||||||||||||||||||||||||||||
Comment from the Stata technical groupDiscovering Structural Equation Modeling Using Stata, by Alan Acock, successfully introduces both the statistical principles involved in structural equation modeling (SEM) and the use of Stata to fit these models. The book uses an application-based approach to teaching SEM. Acock demonstrates how to fit a wide variety of models that fall within the SEM framework and provides datasets that enable the reader to follow along with each example. As each type of model is discussed, concepts such as identification, handling of missing data, model evaluation, and interpretation are covered in detail. In Stata, structural equation models can be fit using the command language or the graphical user interface (GUI) for SEM, known as the SEM Builder. The book demonstrates both of these approaches. Throughout the text, the examples use the sem command. Each chapter also includes brief discussions on drawing the appropriate path diagram and performing estimation from within the SEM Builder. A more in-depth coverage of the SEM Builder is given in one of the book’s appendixes. The first two chapters introduce the building blocks of SEM. Chapter 1 begins with overviews of Cronbach’s alpha as a measure of reliability and of exploratory factor analysis. Then, building on these concepts, Acock demonstrates how to perform confirmatory factor analysis, discusses a variety of statistics available for assessing the fit of the model, and shows a more general measurement of reliability that is based on confirmatory factor analysis. Chapter 2 focuses on using SEM to perform path analysis. It includes examples of mediation, moderation, cross-lagged panel models, and nonrecursive models. Chapter 3 demonstrates how to combine the topics covered in the first two chapters to fit full structural equation models. The use of modification indices to guide model modification and computation of direct, indirect, and total effects for full structural equation models are also covered. Chapter 4 details the application of SEM to growth curve modeling. After introducing the basic linear latent growth curve model, Acock extends this to more complex cases such as the inclusion of quadratic terms, time-varying covariates, and time-invariant covariates. In chapter 5, Acock discusses testing for differences across groups in SEM. He introduces the specialized sem syntax for multiple-group models and discusses the intricacies of testing for group differences for the different types of models presented in the preceding chapters. Discovering Structural Equation Modeling Using Stata is an excellent resource both for those who are new to SEM and for those who are familiar with SEM but new to fitting these models in Stata. It is useful as a text for courses covering SEM as well as for researchers performing SEM. |
||||||||||||||||||||||||||||||||||||
Table of contentsView table of contents >> Dedication
List of tables
List of figures
Acknowledgments
1 Introduction to confirmatory factor analysis
1.1 Introduction
1.2 The "do not even think about it" approach 1.3 The principal component factor analysis approach 1.4 Alpha reliability for our nine-item scale 1.5 Generating a factor score rather than a mean or summative score 1.6 What can CFA add? 1.7 Fitting a CFA model 1.8 Interpreting and presenting CFA results 1.9 Assessing goodness of fit
1.9.1 Modification indices
1.10 A two-factor model 1.9.2 Final model and estimating scale reliability
1.10.1 Evaluating the depression dimension
1.11 Parceling 1.10.2 Estimating a two-factor model 1.12 Extensions and what is next 1.13 Exercises 1.A Using the SEM Builder to run a CFA
1.A.1 Drawing the model
1.A.2 Estimating the model 2 Using structural equation modeling for path models
2.1 Introduction
2.2 Path model terminology
2.2.1 Exogenous predictor, endogenous outcome, and endogenous mediator variables
2.3 A substantive example of a path model 2.2.2 A hypothetical path model 2.4 Estimating a model with correlated residuals
2.4.1 Estimating direct, indirect, and total effects
2.5 Auxiliary variables 2.4.2 Strengthening our path model and adding covariates 2.6 Testing equality of coefficients 2.7 A cross-lagged panel design 2.8 Moderation 2.9 Nonrecursive models
2.9.1 Worked example of a nonrecursive model
2.10 Exercises 2.9.2 Stability of a nonrecursive model 2.9.3 Model constraints 2.9.4 Equality constraints 2.B Using the SEM Builder to run path models 3 Structural equation modeling
3.1 Introduction
3.2 The classic example of a structural equation model
3.2.1 Identification of a full structural equation model
3.3 Equality constraints 3.2.2 Fitting a full structural equation model 3.2.3 Modifying our model 3.2.4 Indirect effects 3.4 Programming constraints 3.5 Structural model with formative indicators
3.5.1 Identification and estimation of a composite latent variable
3.6 Exercises 3.5.2 Multiple indicators, multiple causes model 4 Latent growth curves
4.1 Discovering growth curves
4.2 A simple growth curve model 4.3 Identifying a growth curve model
4.3.1 An intuitive idea of identification
4.4 An example of a linear latent growth curve 4.3.2 Identifying a quadratic growth curve
4.4.1 A latent growth curve model for BMI
4.5 How can we add time-invariant covariates to our model? 4.4.2 Graphic representation of individual trajectories (optional) 4.4.3 Intraclass correlation (ICC) (optional) 4.4.4 Fitting a latent growth curve 4.4.5 Adding correlated adjacent error terms 4.4.6 Adding a quadratic latent slope growth factor 4.4.7 Adding a quadratic latent slope and correlating adjacent error terms
4.5.1 Interpreting a model with time-invariant covariates
4.6 Explaining the random effects—time-varying covariates
4.6.1 Fitting a model with time-invariant and time-varying covariates
4.7 Constraining variances of error terms to be equal (optional) 4.6.2 Interpreting a model with time-invariant and time-varying covariates 4.8 Exercises 5 Group comparisons
5.1 Interaction as a traditional approach to multiple-group comparisons
5.2 The range of applications of Stata’s multiple-group comparisons with sem
5.2.1 A multiple indicators, multiple causes model
5.3 A measurement model application 5.2.2 A measurement model 5.2.3 A full structural equation model
5.3.1 Step 1: Testing for invariance comparing women and men
5.4 Multiple-group path analysis 5.3.2 Step 2: Testing for invariant loadings 5.3.3 Step 3: Testing for an equal loadings and equal errorvariances model 5.3.4 Testing for equal intercepts 5.3.5 Comparison of models 5.3.6 Step 4: Comparison of means 5.3.7 Step 5: Comparison of variances and covariance of latent variables
5.4.1 What parameters are different?
5.5 Multiple-group comparisons of structural equation models 5.4.2 Fitting the model with the SEM Builder 5.4.3 A standardized solution 5.4.4 Constructing tables for publications 5.6 Exercises 6 Epilogue—what now?
A The graphical user interface
A.1 Introduction
A.2 Menus for Windows, Unix, and Mac
A.2.1 The menus, explained
A.3 Designing a structural equation model A.2.2 The vertical drawing toolbar A.4 Drawing an SEM model A.5 Fitting a structural equation model A.6 Postestimation commands A.7 Clearing preferences and restoring the defaults B Entering data from summary statistics
References
|
Learn
Free webinars
NetCourses
Classroom and web training
Organizational training
Video tutorials
Third-party courses
Web resources
Teaching with Stata
© Copyright 1996–2025 StataCorp LLC. All rights reserved.
×
We use cookies to ensure that we give you the best experience on our website—to enhance site navigation, to analyze usage, and to assist in our marketing efforts. By continuing to use our site, you consent to the storing of cookies on your device and agree to delivery of content, including web fonts and JavaScript, from third party web services.
Cookie Settings
Last updated: 16 November 2022
StataCorp LLC (StataCorp) strives to provide our users with exceptional products and services. To do so, we must collect personal information from you. This information is necessary to conduct business with our existing and potential customers. We collect and use this information only where we may legally do so. This policy explains what personal information we collect, how we use it, and what rights you have to that information.
These cookies are essential for our website to function and do not store any personally identifiable information. These cookies cannot be disabled.
This website uses cookies to provide you with a better user experience. A cookie is a small piece of data our website stores on a site visitor's hard drive and accesses each time you visit so we can improve your access to our site, better understand how you use our site, and serve you content that may be of interest to you. For instance, we store a cookie when you log in to our shopping cart so that we can maintain your shopping cart should you not complete checkout. These cookies do not directly store your personal information, but they do support the ability to uniquely identify your internet browser and device.
Please note: Clearing your browser cookies at any time will undo preferences saved here. The option selected here will apply only to the device you are currently using.