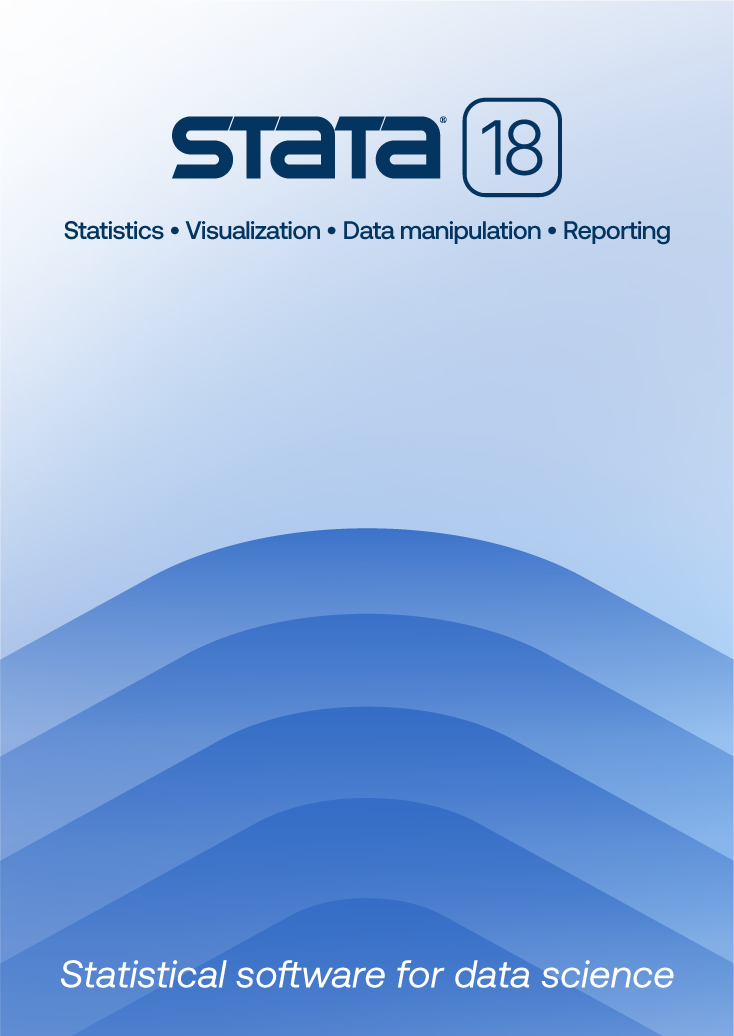
Causal Inference: The Mixtape |
||||||||||||||||||||||||||||||||
![]() Click to enlarge |
![]()
As an Amazon Associate, StataCorp earns a small referral credit from
qualifying purchases made from affiliate links on our site.
eBook not available for this title
eBook not available for this title |
|
||||||||||||||||||||||||||||||
Comment from the Stata technical groupCausal Inference: The Mixtape is a book for practitioners. The purpose of the book is to allow researchers to understand causal inference and work with their data to answer relevant questions in the area. It is the emphasis on the use of statistical software that sets Cunningham's book apart. In each chapter, theoretical details are clearly presented, followed by how to apply the theory to answer causal inference problems using statistical software. The examples are accompanied by readily available data and replication code. The book starts with some basic concepts and then moves into the most commonly used causal inference models and estimators. Topics include directed acyclical graphs, potential outcome models, matching and subclassification, regression discontinuity, instrumental variables, difference in differences, and synthetic control. The inclusion and discussion of synthetic control and directed acyclical graphs differentiates this book from others in the literature, which do not cover these topics or do so tangentially. The structure of the book lends itself to teaching a course on causal inference, but at the same time it is a useful reference for any researcher delving into causal inference. |
||||||||||||||||||||||||||||||||
Table of contentsView table of contents >> Acknowledgments
Introduction
What is Causal Inference?
Do Not Confuse Correlation with Causality Optimization Makes Everything Endogenous Example: Identifying Price Elasticity of Demand Conclusion Probability and Regression Review
Directed Acyclic Graphs
Introduction to DAG Notation
Potential Outcomes Causal Model
Physical Randomization
Randomization Inference Conclusion Matching and Subclassification
Subclassification
Exact Matching Approximate Matching Regression Discontinuity
Huge Popularity of Regression Discontinuity
Estimation Using an RDD Challenges to Identification Replicating a Popular Design: The Close Election Regression Kink Design Conclusion Instrumental Variables
History of Instrumental Variables: Father and Son
Intuition of Instrumental Variables Homogeneous Treatment Effects Parental Methamphetamine Abuse and Foster Care The Problem of Weak Instruments Heterogeneous Treatment Effects Applications Popular IV Designs Conclusion Panel Data
DAG Example
Estimation Data Exercise: Survey of Adult Service Providers Conclusion Difference-in-Differences
John Snow's Cholera Hypothesis
Estimation Inference Providing Evidence for Parallel Trends Through Event Studies and Parallel Leads The Importance of Placebos in DD Twoway Fixed Effects with Differential Timing Conclusion Synthetic Control
Introducing the Comparative Case Study
Prison Construction and Black Male Incarceration Conclusion
Bibliography
Permissions
Index
|
Learn
Free webinars
NetCourses
Classroom and web training
Organizational training
Video tutorials
Third-party courses
Web resources
Teaching with Stata
© Copyright 1996–2024 StataCorp LLC. All rights reserved.
×
We use cookies to ensure that we give you the best experience on our website—to enhance site navigation, to analyze usage, and to assist in our marketing efforts. By continuing to use our site, you consent to the storing of cookies on your device and agree to delivery of content, including web fonts and JavaScript, from third party web services.
Cookie Settings
Last updated: 16 November 2022
StataCorp LLC (StataCorp) strives to provide our users with exceptional products and services. To do so, we must collect personal information from you. This information is necessary to conduct business with our existing and potential customers. We collect and use this information only where we may legally do so. This policy explains what personal information we collect, how we use it, and what rights you have to that information.
These cookies are essential for our website to function and do not store any personally identifiable information. These cookies cannot be disabled.
This website uses cookies to provide you with a better user experience. A cookie is a small piece of data our website stores on a site visitor's hard drive and accesses each time you visit so we can improve your access to our site, better understand how you use our site, and serve you content that may be of interest to you. For instance, we store a cookie when you log in to our shopping cart so that we can maintain your shopping cart should you not complete checkout. These cookies do not directly store your personal information, but they do support the ability to uniquely identify your internet browser and device.
Please note: Clearing your browser cookies at any time will undo preferences saved here. The option selected here will apply only to the device you are currently using.