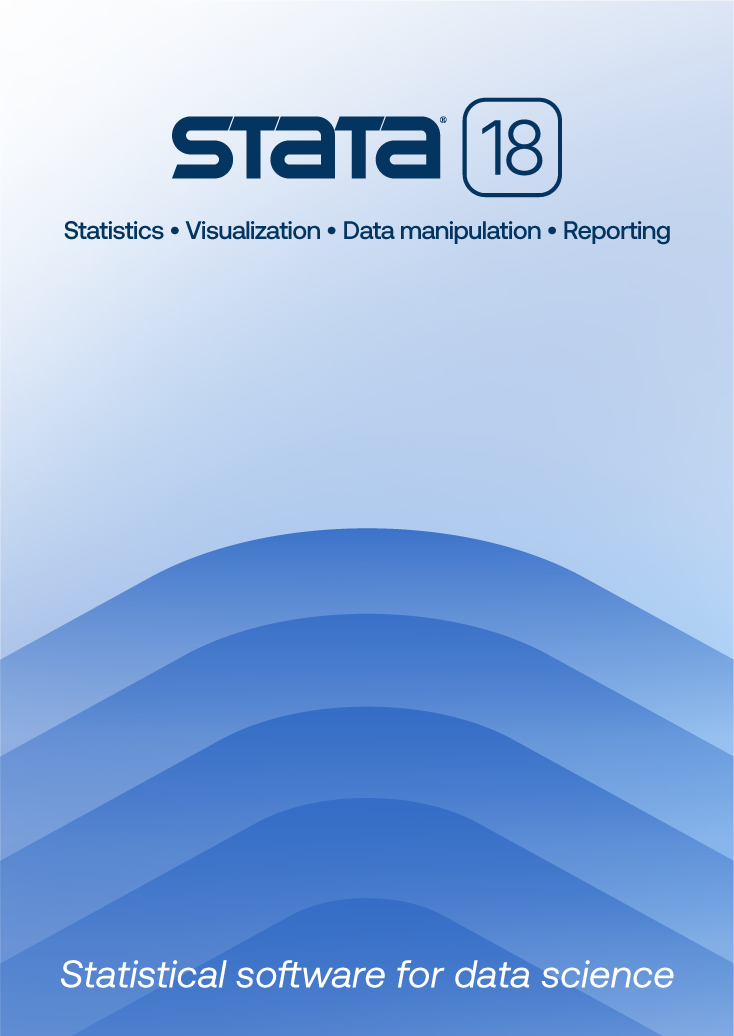
Applied Statistical Learning: With Case Studies in Stata |
||||||||||||||||||||||||||||||||||||
![]() Click to enlarge See the back cover |
![]()
As an Amazon Associate, StataCorp earns a small referral credit from
qualifying purchases made from affiliate links on our site.
eBook not available for this title
eBook not available for this title |
|
||||||||||||||||||||||||||||||||||
Comment from the Stata technical groupMatthias Schonlau’s Applied Statistical Learning is an outstanding resource for anyone eager to learn statistical and machine learning with practical examples in Stata. Tailored for an applied audience, the book seamlessly blends conceptual understanding with hands-on exercises. Readers with an inclination toward mathematical insights will find the author’s explanation in select chapters delightful. The book adeptly explores pivotal topics in statistical and machine learning, making it an indispensable read for individuals unfamiliar with the jargon and concepts of this field. The first three chapters serve as invaluable stepping stones, clarifying specific terminologies and laying a strong foundation for the rest of the book. From there on, the book journeys through methods and algorithms, from logistic regression and lasso regularization to the intriguing worlds of ensembling, stacking, and neural networks. Each chapter features a practical case study, enabling readers to tackle real-world problems using Stata’s official and community-contributed commands. With an abundance of advice, the author guides readers on how to embark on and successfully implement their machine learning projects. Armed with knowledge from this book, readers will not only grasp a profound understanding of popular machine learning algorithms but also find themselves well prepared to tackle their own projects. |
||||||||||||||||||||||||||||||||||||
Table of contentsView table of contents >> Preface
1 Prologue
1.1 Who Should Read This Book?
1.2 How Is This Book Structured? 1.3 Data Sets 1.4 Stata 1.5 For Instructors 1.6 Book Web Page
2 Statistical Learning: Concepts
2.1 Introduction
2.2 What is Statistical Learning?
2.2.1.Statistical Learning, Machine Learning, and Data Science
2.3 Supervised and Unsupervised Learning
2.2.2 Computer Science Terminology
2.3.1 Supervised Learning: Classification
2.4 Interpretation Versus Prediction 2.3.2 Supervised Learning: Regression 2.3.3 Unsupervised Learning 2.5 The Bias-Variance Tradeoff 2.6 Bayes Error
2.6.1 Simulated Example in One Dimension
2.7 Stata Corner 2.6.2 Simulated Example in Two Dimensions 2.8 Summary and Remarks 2.9 Exercises
2.9.1 Conceptual
References
2.9.2 Using Software
3 Statistical Learning: Practical Aspects
3.1 Introduction
3.2 Overfitting 3.3 Assessment on Hold-Out Data
3.3.1 Training/Test Data Sets
3.4 Evaluation Criteria for Assessment
3.3.2 Training/Validation/Test Data Sets 3.3.3 Cross-Validation 3.3.4 LOO Cross-Validation
3.4.1 Criteria for Outcomes with Two Classes
3.5 Regulating the Bias-Variance Tradeoff with Tuning Parameters 3.4.2 Criteria for Multi-class Outcomes 3.4.3 Criteria for Continuous Outcomes 3.6 One-Hot Encoding of Categorical Variables 3.7 Variable Scaling 3.8 Reproducibility 3.9 Stata Corner
3.9.1 Area Under the ROC Curve
3.10 Summary and Remarks 3.9.2 One-Hot Encoding 3.9.3 Scaling 3.9.4 Reproducibility 3.11 Exercises
3.11.1 Conceptual
References
3.11.2 Using Software
4 Logistic Regression
4.1 Introduction
4.2 The Logistic Regression Model
4.2.1 Introduction to Logistic Regression
4.3 Case Study: Pharmacy Compliance 4.2.2 Prediction 4.2.3 Interpretation of the Coefficients 4.2.4 Estimation 4.4 Summary and Remarks 4.5 Exercises
4.5.1 Conceptual
References
4.5.2 Using Software
5 Lasso and Friends
5.1 Introduction
5.2 Regularized Linear Regression
5.2.1 Ridge Regression
5.3 Regularized Logistic Regression 5.2.2 Lasso 5.2.3 Why Can the Lasso Shrink Coefficients to Zero? 5.2.4 Elastic Net 5.4 Inference 5.5 Case Study: Birth Weight
5.5.1 Imputation of Missing Values
5.6 Summary and Remarks 5.5.2 Variable Selection and Prediction 5.5.3 Inference 5.5.4 Stata Corner 5.7 Exercises
5.7.1 Conceptual
References
5.7.2 Using Software
6 Working with Text Data
6.1 Introduction
6.2 Turning Text into Variables
6.2.1 Text as a Bag-of-Words
6.3 Languages Other Than English
6.2.2 Stopwords 6.2.3 Stemming and Lemmatization 6.2.4 N-Gram Variables 6.2.5 Normalization 6.2.6 Rescaling Counts Using TF-IDF 6.2.7 Misspelled Words
6.3.1 Example Spanish: Don Quijote
6.4 The Need for Methods Beyond Linear and Logistic Regression 6.3.2 Example French: Le Petit Prince 6.3.3 Example Swedish: Pippi Longstocking 6.5 Case Study: Beliefs About Immigrants
6.5.1 Writing Two Helper Programs
6.6 Summary and Remarks 6.5.2 An Experiment 6.5.3 Stata Corner 6.7 Exercises
6.7.1 Conceptual
References
6.7.2 Using Software
7 Nearest Neighbors
7.1 Introduction
7.2 k-Nearest Neighbors
7.2.1 Prediction
7.3 Properties of kNN
7.2.2 Distance and Similarity
7.3.1 kNN Accommodates Nonlinear Behavior
7.4 Case Study: Smokers' Helpline Data
7.3.2 kNN Approximates the Bayes Classifier 7.3.3 k Regulates the Bias-Variances Tradeoff 7.3.4 kNN Is Sensitive to Scaling 7.3.5 kNN Is Slow and Memory Hungry
7.4.1 Grid Search for k and Distance Metrics
7.5 Summary and Remarks 7.4.2 Exploring Neighbors 7.4.3 Stata Corner 7.6 Exercises
7.6.1 Conceptual
References
7.6.2 Using Software
8 The Naive Bayes Classifier
8.1 Introduction
8.2 Naive Bayes
8.2.1 Estimation
8.3 Smoothing
8.2.2 Example: Shakespeare in Love
8.3.1 Laplace Smoothing and a Generalization
8.4 Case Study: Patient Joe 8.3.2 The m-estimator 8.5 Summary and Remarks 8.6 Exercises
8.6.1 Conceptual
References
8.6.2 Using Software
9 Trees
9.1 Introduction
9.2 Example: Classifying Shakespeare Plays 9.3 Example: Death Penalty Data 9.4 Trees
9.4.1 Model and Fitting Strategies
9.5 Case Study: Fitting a Tree Using Python
9.4.2 Regression Trees 9.4.3 Classification Trees 9.4.4 Splits with Categorical X-Variables and Missing X-Values 9.4.5 Stopping Rules and Pruning
9.5.1 Stata Corner
9.6 Summary and Remarks 9.7 Exercises
9.7.1 Conceptual
References
9.7.2 Using Software
10 Random Forests
10.1 Introduction
10.2 Bootstrap
10.2.1 Example: Shakespeare Data
10.3 Bagging 10.4 Random Forests
10.4.1 Tuning Parameters
10.5 Case Study: Portugal Student Data
10.4.2 Out-of-Bag Samples 10.4.3 Variable Importance
10.5.1 Tuning
10.6 Summary and Remarks 10.5.2 Out-of-Bag Error vs. Out-of-Sample Error 10.5.3 Comparison to Linear Regression 10.5.4 Variable Importance 10.5.5 Comparison to the Lasso 10.5.6 Stata Corner 10.7 Exercises
10.7.1 Conceptual
References
10.7.2 Using Software
11 Boosting
11.1 Introduction
11.2 Gradient Boosting
11.2.1 Gaussian Gradient Boosting
11.3 XGBoost
11.2.2 Logit Boost 11.2.3 Multinomial Boosting and Beyond 11.2.4 Tuning Parameters 11.2.5 Influence
11.3.1 XGBoost Derivations
11.4 Boosting as a Committee 11.3.2 XGBoost Example 11.3.3 Engineering Innovations 11.5 Case Study: Patient Joe
11.5.1 Pre-processing
11.6 Summary and Remarks 11.5.2 Tuning 11.5.3 Influence 11.5.4 Prediction 11.5.5 Stata Corner 11.7 Exercises
11.7.1 Conceptual
References
11.7.2 Using Software
12 Support Vector Machines
12.1 Introduction
12.2 Support Vector Classification (SVC)
12.2.1 Linearly Separable Classes
12.3 Multi-class Classification 12.2.2 Optimization for Linearly Separable Classes 12.2.3 Classes That Are Not Linearly Separable 12.2.4 Adding Derived Variables to Make Classes Linearly Separable 12.2.5 Nonlinear Kernels 12.2.6 SVM and Logistic Regression Have Similar Loss Functions 12.2.7 Probability Estimates 12.4 Support Vector Regression (SVR)
12.4.1 Illustration of the Effect of Parameters in an RBF Kernel
12.5 Case Study: Online News Popularity
12.5.1 Linear Regression
12.6 Summary and Remarks 12.5.2 SVM Tuning and Prediction 12.5.3 Conclusion 12.7 Exercises
12.7.1 Conceptual
References
12.7.2 Using Software
13 Feature Engineering
13.1 Introduction
13.2 Feature Engineering 13.3 Case Study: Adverse Medical Events
13.3.1 A Helper Program
13.4 Summary and Remarks
13.5 Exercises
13.3.2 Initial Analysis 13.3.3 Deriving Variables Using Regular Expressions 13.3.4 Analysis with Regular Expression Variables 13.3.5 Conclusion
13.5.1 Using Software
References
14 Neural Networks
14.1 Introduction
14.2 Feedforward Neural Networks
14.2.1 Estimation
14.3 Activation Functions
14.2.2 The Analogy to the Human Brain 14.2.3 Feedforward Neural Networks, Multilayer Perceptrons, and Deep Learning 14.2.4 Multi-class Classification and Regression
14.3.1 Activation Functions for Hidden Layers
14.4 Forward Pass/Prediction 14.3.2 Activation Functions for the Output Layer 14.5 Backward Pass
14.5.1 Gradient Descent
14.6 Issues and Improvements
14.5.2 Backpropagation for Regression 14.5.3 Backpropagation for Multi-class Classification
14.6.1 Vanishing and Exploding Gradients
14.7 Case Study: Predicting the Value of a Poker Hand
14.6.2 Weight Initialization 14.6.3 Stochastic Gradient Descent 14.6.4 Batch Normalization 14.6.5 Dropout 14.6.6 Regularization 14.6.7 Cross-entropy vs. Squared Error Loss for Classification
14.7.1 Pre-processing
14.8 Summary and Remarks 14.7.2 Tuning 14.7.3 Prediction 14.9 Exercises
14.9.1 Conceptual
References
14.9.2 Using Software
15 Stacking
15.1 Introduction
15.2 Stacking 15.3 Case Study: Online News Popularity
15.3.1 Stata Corner
References
Index
|
Learn
NetCourses
Classroom and web training
Organizational training
Webinars
Video tutorials
Third-party courses
Web resources
Teaching with Stata
© Copyright 1996–2024 StataCorp LLC. All rights reserved.
×
We use cookies to ensure that we give you the best experience on our website—to enhance site navigation, to analyze usage, and to assist in our marketing efforts. By continuing to use our site, you consent to the storing of cookies on your device and agree to delivery of content, including web fonts and JavaScript, from third party web services.
Cookie Settings
Last updated: 16 November 2022
StataCorp LLC (StataCorp) strives to provide our users with exceptional products and services. To do so, we must collect personal information from you. This information is necessary to conduct business with our existing and potential customers. We collect and use this information only where we may legally do so. This policy explains what personal information we collect, how we use it, and what rights you have to that information.
These cookies are essential for our website to function and do not store any personally identifiable information. These cookies cannot be disabled.
This website uses cookies to provide you with a better user experience. A cookie is a small piece of data our website stores on a site visitor's hard drive and accesses each time you visit so we can improve your access to our site, better understand how you use our site, and serve you content that may be of interest to you. For instance, we store a cookie when you log in to our shopping cart so that we can maintain your shopping cart should you not complete checkout. These cookies do not directly store your personal information, but they do support the ability to uniquely identify your internet browser and device.
Please note: Clearing your browser cookies at any time will undo preferences saved here. The option selected here will apply only to the device you are currently using.