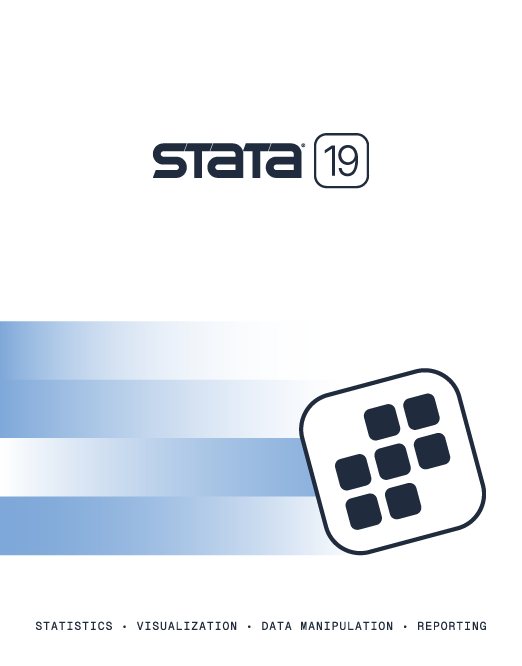
Outcomes and regression estimators
Types of models
Types of effects
Error (residual) structures for linear models
Small-sample inference in linear models (DDF adjustments)
Constraints
Survey data for generalized linear and survival models
Estimates of random effects
Predictions
Other postestimation analysis
Additional resources
See New in Stata 19 to learn about what was added in Stata 19.