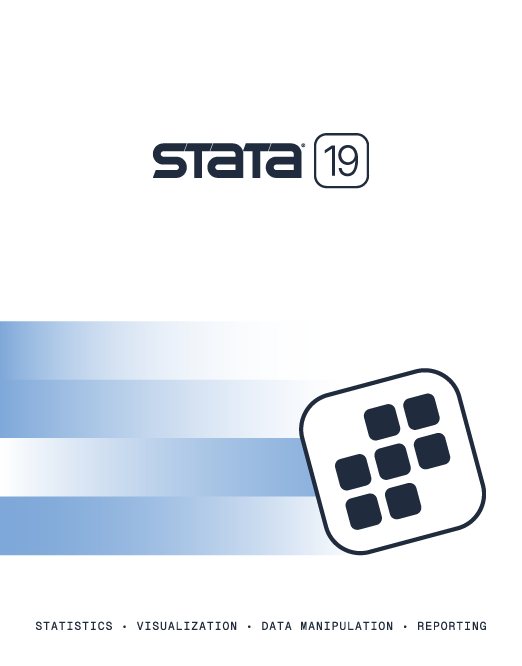
2025 Stata Conference • Nashville, TN • 31 July–01 August
Title | Likelihood-ratio test after survey/robust ML estimation | |
Author | William Sribney, StataCorp |
The “likelihood” for pweighted or clustered MLEs is not a true likelihood; i.e., it is NOT the distribution of the sample. When there is clustering, individual observations are no longer independent, and the “likelihood” does not reflect this. Where there are pweights, the “likelihood” does not fully account for the “randomness” of the weighted sampling.
The “likelihood” for pweighted or clustered MLEs is used only for the computation of the point estimates and should not be used for variance estimation using standard formulas. Thus the standard likelihood-ratio test should NOT be used after estimating pweighted or clustered MLEs.
Instead of likelihood-ratio tests (the lrtest command), Wald tests (the test command) should be used.
The svy commands allow the use of the test command, which computes an adjusted Wald test. This adjustment is useful when the total number of clusters is small (<∼100).
The test command also has a mtest(bonferroni) option. Some statisticians argue that the mtest(bonferroni) option of test gives a better test than an adjusted Wald test. See
Korn, E. L., and B. I. Graubard. 1990.
Simultaneous testing of regression coefficients with complex survey
data: use of Bonferroni t statistics.
The American Statistician 44: 270–276.
for such an argument.
The Bonferroni adjustment carries the tacit assumption that the multidimensional test (let k = the dimension) being conducted consists of k hypotheses that individually make sense as different research questions—or said more precisely, a priori you should have no knowledge that the individual hypotheses are highly collinear. If the individual hypotheses ARE highly collinear, then the Bonferroni adjustment can be overly conservative. So if you suspect this in advance, you might want to stay away from the Bonferroni adjustment. But, in statistics at least, being conservative is safe, so doing the Bonferroni adjustment should be fine.
Learn
Free webinars
NetCourses
Classroom and web training
Organizational training
Video tutorials
Third-party courses
Web resources
Teaching with Stata
© Copyright 1996–2025 StataCorp LLC. All rights reserved.
×
We use cookies to ensure that we give you the best experience on our website—to enhance site navigation, to analyze usage, and to assist in our marketing efforts. By continuing to use our site, you consent to the storing of cookies on your device and agree to delivery of content, including web fonts and JavaScript, from third party web services.
Cookie Settings
Last updated: 16 November 2022
StataCorp LLC (StataCorp) strives to provide our users with exceptional products and services. To do so, we must collect personal information from you. This information is necessary to conduct business with our existing and potential customers. We collect and use this information only where we may legally do so. This policy explains what personal information we collect, how we use it, and what rights you have to that information.
These cookies are essential for our website to function and do not store any personally identifiable information. These cookies cannot be disabled.
This website uses cookies to provide you with a better user experience. A cookie is a small piece of data our website stores on a site visitor's hard drive and accesses each time you visit so we can improve your access to our site, better understand how you use our site, and serve you content that may be of interest to you. For instance, we store a cookie when you log in to our shopping cart so that we can maintain your shopping cart should you not complete checkout. These cookies do not directly store your personal information, but they do support the ability to uniquely identify your internet browser and device.
Please note: Clearing your browser cookies at any time will undo preferences saved here. The option selected here will apply only to the device you are currently using.