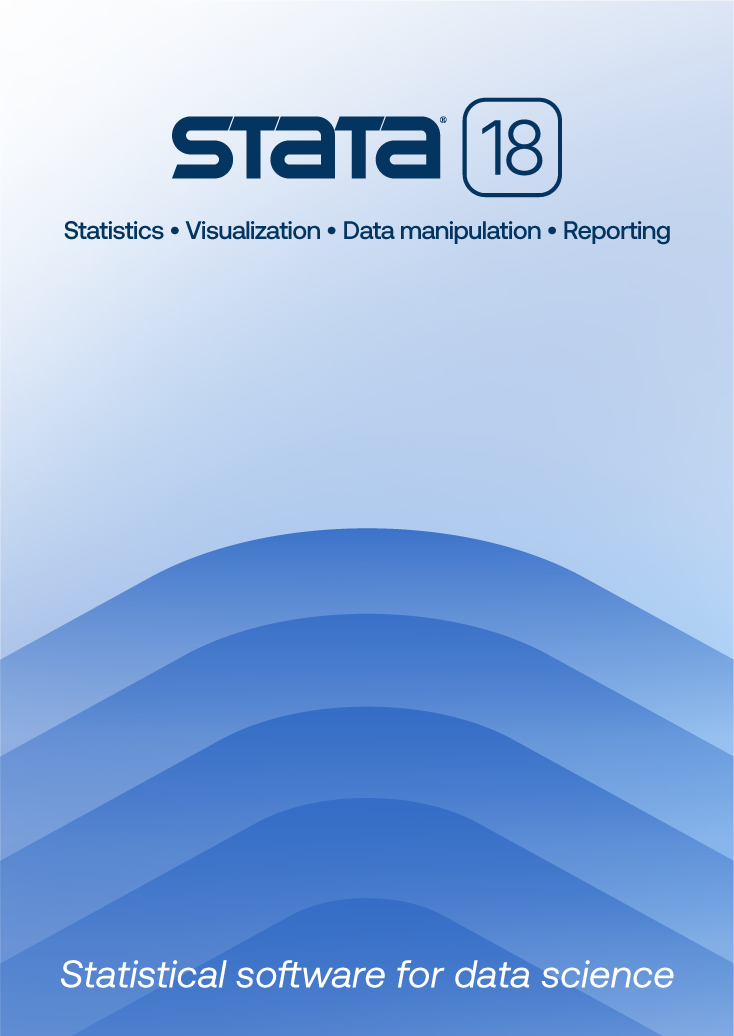
Title | Treatment endogeneity versus sample selection bias | |
Author | Charles Lindsey, StataCorp Daniel Millimet, Southern Methodist University |
Many individuals have posted questions using the terms "sample selection bias" and "treatment endogeneity" interchangeably or incorrectly. I do not intend to single out one individual, but consider the case of being in a trade union on workers' wages. Using a dummy variable to pick up this effect in a pooled sample of union and nonunion workers is inappropriate, because workers in unions may self-select, and workers being in a union may not be random.
One approach I have read is to use a probit model to estimate the probability of being in a union (1 being union worker and 0 being nonunion worker). Then from the probit equation, obtain predicted probabilities of being a union worker for the entire sample of union and nonunion workers. Then use these predicted probabilities in place of a union dummy variable to estimate the effect of being in a union. This approach should control for sample selection bias.
I am trying to relate this procedure with the standard Heckman’s two-stage procedure that uses the inverse Mills’ ratio. Any help will be much appreciated.
Endogenous sample selection and endogenous treatment assignment are common problems in observational data. They may occur separately or together. Stata has many tools to deal with sample selection and endogenous treatment in the linear regression model that you mentioned. Stata can also deal with sample selection and endogenous treatment in nonlinear models like a Poisson regression and a probit regression.
Sample selection is an ambiguous term because different authors have used it to mean different things. To add more ambiguity, sample selection has been equated with nonresponse bias and selection bias in some disciplines. Much of the ambiguity arises from authors being imprecise about when sample selection is ignorable. Under sample selection, a process maps each individual into or out of the sample. This process depends on observable covariates and unobservable factors. When unobservable factors that affect who is in the sample are independent of unobservable factors that affect the outcome, the sample selection is not endogenous. In this case, the sample selection is ignorable—our estimator that ignores sample selection (e.g. regress in the linear case) is still consistent. In contrast, when the unobservable factors that affect who is included in the sample are correlated with the unobservable factors that affect the outcome, the sample selection is endogenous and not ignorable, because estimators that ignore endogenous sample selection are not consistent in this case.
Treatment-effect regressions model the effect of a discrete treatment or intervention on the outcome. In observational data, we cannot randomly assign a treatment of interest to individuals. Treatment status may be related to other covariates that we measure. It may even be related to the unobserved factors that affect the outcome and be endogenous. The treatment may be interpreted as a covariate that affects the outcome. Estimators that ignore the endogeneity of the treatment will be inconsistent, just like estimators that ignore covariate endogeneity.
Stata provides several commands to estimate treatment effects in linear regressions with an endogenous treatment. eregress with the option entreat() can be used to estimate the parameters of a linear regression with an endogenous treatment. eregress and the other extended regression model (ERM) commands can also accommodate endogenous sample selection and endogenous covariates. The treatment may have an intercept effect on the outcome, or both intercept and slope effects (where the betas of the other covariates differ by treatment level). The estat teffects command can be used after eregress to estimate average treatment effects (ATEs) and potential-outcome means (POMs). The eteffects command can also be used. Additionally, etregress can be used to estimate the parameters of a linear regression with an endogenous treatment. etregress allows different correlations between treatment assignment errors and the outcome errors in the control and treatment groups. The variance for each treatment group may differ as well.
The parameters of Poisson regressions with an endogenous treatment can be estimated by using the etpoisson command. The ERM commands eprobit and eoprobit can be used with the entreat() option to fit probit and ordinal probit regressions with an endogenous treatment. The ERM command eintreg can also be used with the entreat() option to fit an interval regression with an endogenous treatment. eteffects can also be used to estimate treatment effects in nonlinear models.
The heckman command can be used to estimate the parameters of a linear regression that suffers from endogenous sample selection. With the select() option, eregress can also be used. heckprobit and heckoprobit can be used to fit probit and ordinal probit models with endogenous sample selection. A Poisson regression with endogenous sample selection can be fit using heckpoisson. The select() option can also be used with the eprobit, eoprobit, and eintreg commands to fit probit, ordinal probit, and interval regression models with endogenous sample selection.
select() and entreat() can be specified together in eregress, allowing a user to estimate the parameters of a model with an endogenous treatment drawn from an endogenously selected sample. select() and entreat() can also be specified together when using eprobit, eoprobit, and eintreg.
The xteregress command is the equivalent alternative to eregress to fit random-effects linear models. The other three currently available equivalent alternatives for random-effects models are xteprobit, xteoprobit, and xteintreg. All four panel-data commands referred to here support the options select() and entreat().
Stata 15 announced, available now
Using mlexp to estimate endogenous treatment effects in a probit model
Probit model with sample selection by mlexp
Multiple-equation models: Estimation and marginal effects using gmm