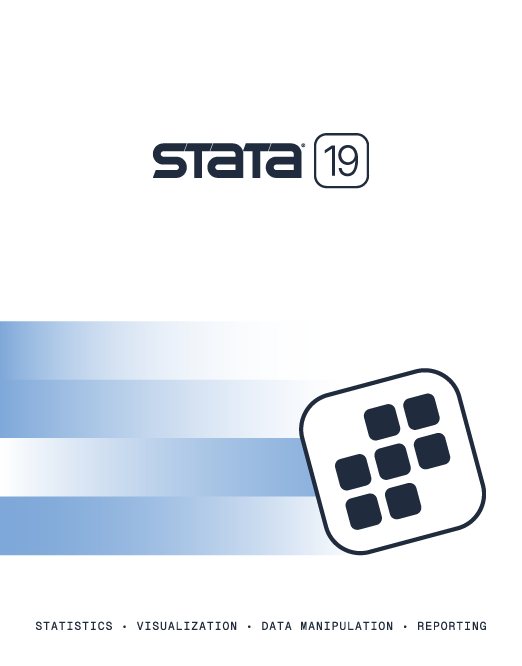
9:05–9:30 | Drivers of COVID-19 deaths in the United States: A two-stage modeling approach
Abstract:
We offer a two-stage (time-series and cross-section) econometric
modeling approach to examine the drivers behind the spread of
COVID-19 deaths across counties in the United States.
Our empirical strategy exploits the availability of two years
(January 2020 through January 2022) of daily data on the number
of confirmed deaths and cases of COVID-19 in the 3,000 U.S.
counties of the 48 contiguous states and the District of
Columbia. In the first stage of the analysis, we use daily
time-series data on COVID-19 cases and deaths to fit mixed
models of deaths against lagged confirmed cases for each county.
Because the resulting coefficients are county specific, they
relax the homogeneity assumption that is implicit when the
analysis is performed using geographically aggregated
cross-section units. In the second stage of the analysis, we
assume that these county estimates are a function of economic
and sociodemographic factors that are taken as fixed over the
course of the pandemic. Here we employ the novel
one-covariate-at-a-time variable-selection algorithm proposed by
Chudik et al. (2018) to guide the choice of regressors.
Additional information:
Kit Baum
Boston College
|
9:30–9:55 | Estimation of two-stage models in individual participant data meta-analysis with missing data
Abstract:
Individual participant data (IPD) meta-analysis often has
missing data and is analyzed in two-steps: estimates are first
obtained within each individual study and then averaged across
studies.
The current mi suite of commands for dealing with missing
data does not allow a two-stage approach in fitting
regression models. Therefore, I introduce a new command,
twostage, that offers to fit two-stage regression models
for IPD meta-analysis with missing data. twostage has
been developed to accommodate systematic and sporadically
missing data in IPD meta-analysis. I first briefly describe the
challenges of missing data in IPD meta-analysis and then
illustrate applications of the twostage command in the
context of health-related studies.
Additional information:
Robert Thiesmeier
Karolinska Institutet
|
9:55–10:20 | Imputation of systematic missing data in individual participant data meta-analysis
Abstract:
Answering research questions in light of multiple studies is
challenged by one or more variables being 100% unobserved by
design, also known as systematic missing data.
The current imputation methods implemented in mi,
however, are mainly suited for one study and sporadically
missing data. Our aim is to introduce a new user-defined
imputation method within mi impute capable of handling
the main features of individual participant data (IPD)
meta-analysis. Realistic simulated studies will be used to
illustrate the logic and practice of imputing systematic missing
data.
Additional information:
Nicola Orsini
Karolinska Institutet
|
11:15–11:40 | A command for estimating regression parameters for the maximum agreement predictor
Abstract:
This presentation presents mareg, a command for
estimating the coefficients of maximum agreement regression
models for an outcome variable given predictors.
Recently introduced by Bottai et al. (The American Statistician.
2022. 76:4, 313–321), maximum agreement regression
maximizes the concordance correlation between the prediction and
the observed outcome, not the Pearson's correlation coefficient
maximized by ordinary linear regression. The syntax of the
command is nearly identical to that of regress, which
estimates least-squares regression. The presentation shows the
features of the command and its possible applications through
real data examples.
Additional information:
Matteo Bottai
Karolinska Institutet
|
11:40–12:05 | Regression to the mean and randomized control trials with continuous outcomes
Abstract:
Measurement errors in a study make the “regression to the
mean” occur to different degrees.
To remedy the “regression to the mean” effect in
randomized control trials, one should measure the continuous
outcome before randomization and adjust for the baseline outcome
value in the analysis. This adjustment requires the use of
regression constraints. The adjustment leads to lesser standard
errors. After presenting a real case, I introduce the concept
of “regression to the mean.” Then I introduce the
relation from “regression to the mean” to the
intraclass correlation and the measurement error. Using the
case, I compare the estimates from several approaches in
randomized control trials. Here I demonstrate the use of
constraints. Knowing the intraclass correlation in power
calculations will lead to a lesser required number of
observations, for example, higher power. Hence, randomized
control trials should report the intraclass correlation.
Additional information:
Nils Henrik Bruun
Aalborg University Hospital
|
1:10–2:10 | Heterogeneous difference-in-differences estimation
Abstract:
Treatment effects might differ over time and for groups that are
treated at different points in time.
These groups are known as treatment cohorts. In Stata 18, we
introduced two commands that estimate treatment effects that
vary over time and cohort. For repeated cross-sectional data, we
have hdidregress. For panel data, we have
xthdidregress. Both commands let you graph the evolution
of treatment over time. They also allow you to aggregate
treatment within cohort and time and visualize these effects. I
will show you how both commands work and briefly discuss the
theory underlying them.
Additional information:
Enrique Pinzón
StataCorp LLC
|
2:10–2:35 | Modeling hazard rates with multiple time scales: An application study
Abstract:
There are situations when we need to model multiple time scales
in survival analysis.
A usual approach would involve fitting Cox or Poisson models to
a time-split dataset. However, this leads to large datasets and
can be computationally intensive when model fitting, especially
if interest lies in displaying how the estimated hazard rate or
survival changes along multiple time scales continuously.
Flexible parametric survival models on the log-hazard scale are
an alternative method when modeling data with multiple
time scales. This can be achieved by using the Stata package
stmt, where one of the time scales is chosen to be a
primary time scale, and the other time scale(s) is(are)
specified by using the offset option. Through a case study,
I will demonstrate this method and provide examples of graphical
representations.
Additional information: Presentation not avilable
Nurgul Batyrbekova
Karolinska Institutet
|
3:00–3:25 | Hierarchical survival models: Estimation, prediction, interpretation
Abstract:
Hierarchical time-to-event data is common across various
research domains.
In the medical field, for instance, patients are often nested
within hospitals and regions, while in education, students are
nested within schools. In these settings, the outcome is
typically measured at the individual level, with covariates
recorded at any level of the hierarchy. This hierarchical
structure poses unique challenges and necessitates appropriate
analytical approaches. Traditional methods, like the widely used
Cox model, assume the independence of study subjects,
disregarding the inherent correlations among subjects nested
within the same higher-level unit (such as a hospital).
Consequently, failing to account for the multilevel structure
and within-cluster correlation can yield biased and inefficient
results.
To address these issues, one can use mixed-effects models, which incorporate both population-level fixed effects and cluster-specific random effects at various levels of the hierarchy. Stata users can leverage several powerful commands to fit hierarchical survival models, such as mestreg and stmixed. With this presentation, I introduce and demonstrate the use of these commands, including a range of postestimation predictions. Moreover, I delve into measures that quantify the impact of the hierarchical structure, commonly referred to as contextual effects in the literature, and discuss the interpretation of model-based predictions, focusing on the difference between conditional and marginal effects.
Additional information:
Alessandro Gasparini
Red Door Analytics AB
|
3:25–3:50 | Modeling excess mortality comparing with a control population: A combined additive and relative hazards model
Abstract:
In this presentation, I propose a flexible parametric excess
hazard model on the log-hazard scale, incorporating a modeled
expected rate from a control population (for example, matched
comparators).
Covariate effects are assumed to be multiplicative within both
the expected hazard and the excess hazard, while the presence of
disease among the studied group has an additive effect, hence
the excess hazard. By modeling the expected rate, we can
appropriately allow for uncertainty. The model is extended to
include time-dependent effects, multiple time scales, and more.
Following estimation, we quantify results through the prediction
of the survival, hazard, and cumulative incidence functions, as
well as transformations of these, and crucially with associated
confidence intervals on all measures. The proposed method has
been implemented in the Stata package stexcess
(github.com/RedDoorAnalytics/stexcess).
Additional information:
Caroline Weibull
Karolinska Institutet and Red Door Analytics AB
|
3:50–4:15 | Health technology assessment and Stata: Reviewing the old and coding the new
Abstract:
Health technology assessment (HTA) utilizes a wide variety of
statistical methods to evaluate clinical and cost effectiveness
of treatments, including survival analysis and meta-analysis.
In this presentation, I will briefly review some of the
available features in Stata that have been developed over the
years, with a focus towards their use in HTA, and describe some
ongoing work to improve their applicability in such settings.
This will include flexible survival modeling with merlin,
Markov, semi-Markov and non-Markov multistate modeling with
multistate, and efficient and generalizable individual
patient simulation with survsim. Finally, I will
introduce some new tools, such as the maic command for
conducting matched-adjusted indirect comparisons, and a new
prefix command for stmerlin, providing Bayesian flexible
survival models.
Additional information:
Michael Crowther
Red Door Analytics AB
|
4:15–5:00 | Open panel discussion with Stata developers
Contribute to the Stata community by sharing your feedback with StataCorp's developers. From feature improvements to bug fixes and new ways to analyze data, we want to hear how Stata can be made better for our users.
|
The 2023 Northern European Stata Conference is jointly organized by Metrika Consulting AB, the official distributor of Stata for Russia and the Nordic and Baltic countries, and the Biostatistics Team at the Department of Global Public Health, Karolinska Institutet.
View the proceedings of previous Stata Conferences and Users Group meetings.