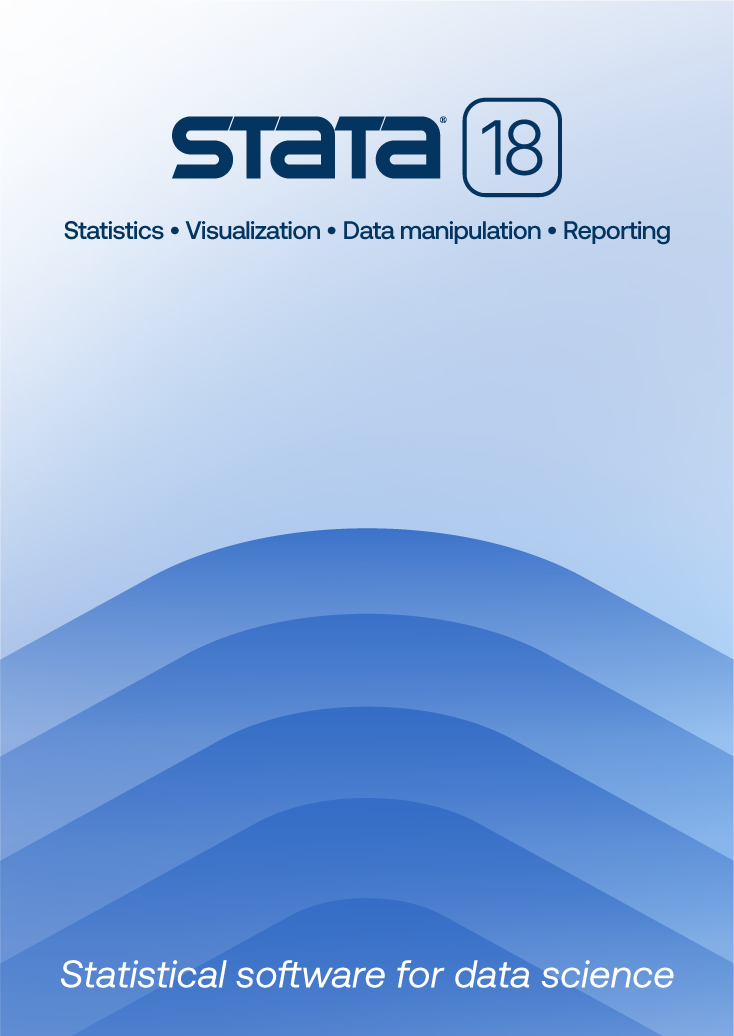
The 18th Italian Stata Conference will take place on 9 May 2024 at the Villa la Stella. There will also be an optional workshop on 10 May.
Meet researchers from different disciplinary areas, discover new applications highlighting Stata 18’s potential capabilities for applied research, exchange new community-contributed routines developed for Stata, and interact directly with statisticians from StataCorp. The second day of the conference will be dedicated to a Stata workshop.
All times are CEST (UTC +2)
8:30–9:00 | Registration |
9:00–9:45 | Session I: Invited speakerStructural equation modeling with partial least squares using Stata Abstract:
Structural equation modeling (SEM) is a multivariate statistical
framework that can model both observed and unobserved
(latent) variables through complex relationships. In this talk, we
present plssem, a community-contributed Stata package for partial
least-squares SEM, an approach to SEM which has attracted a
lot of interest in the last 20 years from an increasing number
of researchers and practitioners from many fields such as
marketing, information systems, economics, psychology, and
others. After introducing the topic to the audience, we will
illustrate the current architecture of the package and its main
features.
Contributor:
Mehmet Mehmetoglu
Norwegian University of Science and Technology
Sergio Venturini
Univeristà Cattolica del Sacro Cuore Cremona
|
9:45–11:30 | Session II: Community contributed, IOptimal policy learning using Stata Abstract:
This presentation introduces the Stata package opl for
optimal policy learning, facilitating ex ante policy impact
evaluation within the Stata environment. Despite theoretical
progress, practical implementations of policy-learning
algorithms are still poor within popular statistical software.
To address this limitation, the package implements three
popular policy learning algorithms in Stata (threshold-based,
linear-combination, and fixed-depth decision tree),
and provides practical demonstrations of them using a real
database. Also, I present a policy scenario development
proposing a menu strategy, which is particularly useful when selection
variables are affected by welfare monotonicity. Overall, the
package contributes to bridging the gap between theoretical
advancements and practical applications of policy learning.
Giovanni Cerulli
IRcRES
Using marginal effects for interpretation in item response theory and in tests of differential item functioning: Introducing Stata commands irt_me and irt_dif Abstract:
The field of categorical data analysis has largely shifted from
the limitations of coefficient interpretations to the more
flexible and powerful possibilities afforded by marginal effects,
spurred by Stata’s widespread implementation of the margins
command. Despite item response theory being the latent variable
corollary of categorical data analysis, a similar transformation
in interpretation tools and practices has yet to emerge. I
propose using tests of marginal effects for interpretation in
item response theory models, demonstrating the advantages to
this strategy over focusing on coefficients. Further, I show
how to solve several issues when translating the idea of
marginal effects to a latent variable model. A new command,
irt_me, automates the estimation of marginal effects after any
item response theory model (irt) in Stata, including models with
binary, ordinal, nominal, and count items (or a mix).
Differential item functioning is a method of detecting item bias that has traditionally relied on tests of interaction terms in the item response theory coefficients. However, it is well established in the categorical data analysis realm that coefficients are inappropriate for tests of interaction: tests of the equality of marginal effects are instead the recommended approach. A new command, irt_dif, provides tests of differential item functioning by testing the equality of marginal effects from item response theory models fit across separate groups.
Trenton D. Mize
Purdue University
(10:45–11:00 Break) Too much or too little? New tools for the CCE estimator Abstract:
This talk will cover new developments in the literature of
common correlated effects (CCE) and their implementation in
Stata. First, I will discuss regularized CCE. CCE is known to be
sensitive to the selection of the number of cross-section
averages. CCE overcomes the problem by regularizing the
cross-section averages. Secondly, I will discuss the test for
the rank condition based on DeVos, Everaert, and Sarafidis
(2024, Econometrics Reviews). If the rank condition fails,
CCE will be inconsistent and therefore testing the condition is key
for any empirical application. Finally, I will show the selection of
cross-section averages using the information criteria from
Karabiyik, Urbain and Westerlund (2019, Journal of Applied
Econometrics) and Margaritella and Westerlund (2023, The
Econometrics Journal).
Jan Ditzen
Free University of Bozen
|
11:30–12:30 | Session III: Exploiting the potential of Stata 18, ICausal mediation analysis with Stata Abstract:
Causal inference is an essential goal in many research areas and
aims at identifying and quantifying causal effects. By
decomposing causal effects into direct and indirect effects,
causal mediation provides further insight into underlying
mechanisms through which causal effects operate. This talk
presents the basic theoretical framework for causal mediation
analysis and discusses a variety of examples using Stata’s
mediate command. Examples will include linear and generalized
linear models using a variety of outcome and mediator variables
as well as different types of treatments.
Joerg Luedicke
StataCorp
|
12:30–1:30 | Lunch |
1:30–2:30 | Session IV: Stata tips and tricksnnls: Nonnegative least squares using Stata Abstract:
The nnls command enables users to carry out nonnegative
least squares using Stata calling Python in the background.
A simple application of the nnls Stata command on real data will
be provided.
Giovanni Cerulli
IRcRES
htmltab2stata: Converting HTML tables into a Stata dataset Abstract:
htmltab2stata parses HTML code from websites. It detects tables
enclosed with the HTML <table> environment and transforms the
table into a Stata dataset.
Jan Ditzen
Free University of Bozen
Implementing groupwise – heteroskedasticity – robust – variance – covariance estimators in fixed-effects panel-data regression with Stata Abstract:
Stock and Watson (2008) prove that the plain White
heteroskedasticity-robust VCE is generally inconsistent for fixed
T , N -> ∞ in fixed-effects panel-data regression. Bruno
(2024) proves that the aforementioned VCE is (fixed T , N _>
∞) consistent under groupwise heteroskedasticity (GH), that is,
when the conditional variance of the idiosyncratic error is
time-invariant but can vary across individuals. As is well
known, the vce(robust) option of xtreg in Stata implements the
cluster–robust VCE, not the White VCE. In this talk, I show
simple Stata procedures to implement the White VCE and a second
GH-robust VCE in fixed-effects panel-data regression. Monte
Carlo experiments prove that both VCEs, under GH, have good
finite-sample properties, compared to the bias-adjusted VCE by
Stock and Watson and the cluster–robust VCE.
Giovanni Bruno
Bocconi University
|
2:30–4:00 | Session V: Exploiting the potential of Stata 18, IIBayesian model averaging Abstract:
Are you unsure which predictors to include in your model?
Rather than choosing one model, aggregate results across all
candidate models to account for model uncertainty with Bayesian
model averaging (BMA). Which predictors are important given the
observed data? Which models are more plausible? How do
predictors relate to each other across different models? BMA can
answer these questions and many more.
Stata 18 introduced the bma suite of commands to perform BMA in linear regression models. In this talk, you will learn how to explore influential models, make inferences, and obtain better predictions with BMA. I will demonstrate the utility of BMA for any researcher—Bayesian, frequentist, and everyone in between! No prior knowledge of the Bayesian framework is required.
Meghan Cain
StataCorp
Text mining in economics and health economics using Stata Abstract:
Within the more relevant data science topics, text mining is an
important and active research area that offers various ways to
extract information and insights from text data. Its continued
use and improvement could drive innovation in several areas and
improve our ability to interpret, evaluate, and utilize the vast
amounts of unstructured text produced in the digital age.
Extracting insightful information from text data through text
mining in healthcare and business holds great promise. Text
mining in business can provide insightful information by
analyzing large amounts of text data, including research papers,
news, and financial reports. It can help analyze market
sentiment, identify emerging trends, and more accurately predict
economic indicators by economists. For example, economists can
find terms or phrases that reflect investment behavior and
sentiment changes by applying text-mining methods to financial
news. Text mining can provide essential insights into health
economics by examining various textual data, including patient
surveys, clinical trials, medical records, and health policy.
Researchers and policymakers can use it to understand healthcare
utilization patterns better, identify the variables that influence
patient outcomes, and evaluate the effectiveness of
different healthcare treatments. Text mining can examine
electronic health data and identify trends in disease incidence,
treatment effectiveness, and healthcare utilization. In this
presentation, I will illustrate the instruments currently
available in Stata to facilitate several text-mining methods.
Carlo Drago
University Niccolò Cusano
|
4:00–4:15 | Break |
4:15–5:30 | Session VI: Community-contributed, IIgeoplot: A new command to draw maps Abstract:
geoplot is a new command for drawing maps from shapefiles
and other datasets. Multiple layers of elements such as regions,
borders, lakes, roads, labels, and symbols can be freely
combined, and the look of elements (for example, color) can be varied
depending on the values of variables. Compared with previous
solutions in Stata, geoplot provides more user convenience, more
functionality, and more flexibility. In this talk, I will
introduce the basic components of the command and illustrate its
use with examples.
Ben Jann
University of Bern
Fitting spatial autoregressive logit and probit models using Stata: The spatbinary command Abstract:
Spatial regressions can be estimated in Stata using the
spregress, spxtregress, and spivregress commands.
These commands allow users to fit spatial autoregressive models in
cross-sectional and panel data. They are designed to estimate
regressions with continuous dependent variables. The spatbinary
command now allows Stata users to fit spatial logit and probit
models, which are important models in applied econometrics.
Daniele Spinelli
University of Milano-Bicocca
|
5:30–5:50 | Session VII: Application study using StataDo alternative work arrangements substitute standard employment? Evidence from worker-level data Abstract:
This study analyzes the impact of an alternative work
arrangement (AWA) called “voucher” on earnings of atypical
workers and on their alternative income sources using Italian
administrative data. Specifically, I investigate whether this
form of very flexible work substitutes income from more
standard labor contracts and welfare transfers related to
employment insurance (sick and parental leave and unemployment
benefits). I estimate cross-income elasticities using fixed
effects and difference-in-differences specifications that correct for sample
selection of individuals in the labor market. Results show that
vouchers increase overall labor income, but they also substitute
earnings derived from other labor contracts. I do not find
relevant associations between vouchers and welfare transfers.
The positive effect of vouchers on total income is smaller in
specifications that correct for sample selection bias, and the
substitution effect with other labor income sources is
substantially larger. Overall, my findings show that AWAs tend
to substitute standard employment, with small positive net
effects on earnings, which are larger for intensive users of
vouchers, and in geographic regions with a more sizable informal
sector.
Filippo Passerini
University of Bologna and LABORatorio R. Revelli
|
5:50–6:15 | Open panel discussion with Stata developers
Contribute to the Stata community by sharing your feedback with StataCorp's developers. From feature improvements to bug fixes and new ways to analyze data, we want to hear how Stata can be made better for our users.
|
Sergio Venturini (Università Cattolica del Sacro Cuore–Cremona)
Mehmet Mehmetoglu (Norges teknisk-naturvitenskapelige universitet)
10 May 2024
PLS-SEM, also referred to as partial least-squares path modeling, is an alternative approach to SEM that is being increasingly used in social sciences, psychology, business administration, and marketing. PLS-SEM can be viewed as a component-based SEM alternative to the covariance-based structural equation modeling, which can be described as a factor-based SEM technique. As such, the PLS-SEM approach provides researchers with a multivariate statistical technique that can readily be used to fit exploratory and complex SEM models. Although there are several standalone specialized PLS-SEM software packages available, this course introduces participants to the PLS-SEM methodology through the community-contributed Stata package plssem, which was developed by the course instructors.
The course is of particular interest to researchers and professionals working in social sciences, psychology, business administration, marketing and management. Because of its introductory nature, it is also accessible to individuals, regardless of their respective disciplines or fields, who need to acquire the requisite toolset to apply the PLS-SEM methodology to their own data. During the course, theoretical concepts are reinforced by applied case study examples in which the course instructors discuss current research issues, highlighting potential pitfalls and the advantages of individual techniques.
Participants are expected to have knowledge in basic statistics. More specifically, a working knowledge of linear regression analysis is required. Previous exposure to Stata or other statistical software packages would also be an advantage.
Conference fees include coffee breaks, lunch, course materials, and a temporary Stata license for those attending the workshop. TStat has also organized a limited number of single rooms at the venue (on a bed and breakfast basis) for those wishing to stay on the complex.
Conference fees (VAT not incl.) |
Student | Other |
---|---|---|
Conference only | € 65 | € 95 |
Conference + room (1 night) |
€ 145 | € 175 |
Conference + workshop | € 245 | € 375 |
Conference + workshop + room (2 nights) |
€ 405 | € 535 |
Registration deadline is 30 April 2024.
Visit the official conference page for more information.
TStat is delighted to sponsor, via our project “Investing in Young Researchers”, two (2) full-time PhD students from any of the countries for which TStat is the official Stata Distributor. Sponsorship covers both the first day of the conference and the workshop (including the bed and breakfast accommodation). Travel expenses are to be paid for the participant. To apply for sponsorship, please send your curriculum vitae to [email protected].
The logistics organizer for the 2024 Italian Stata Conference is TStat S.r.l., the distributor of Stata for Italy, Albania, Bosnia and Herzegovina, Greece, Kosovo, North Macedonia, Malta, Montenegro, Serbia, Slovakia, and Slovenia.
View the proceedings of previous Stata Conferences and Users Group meetings.