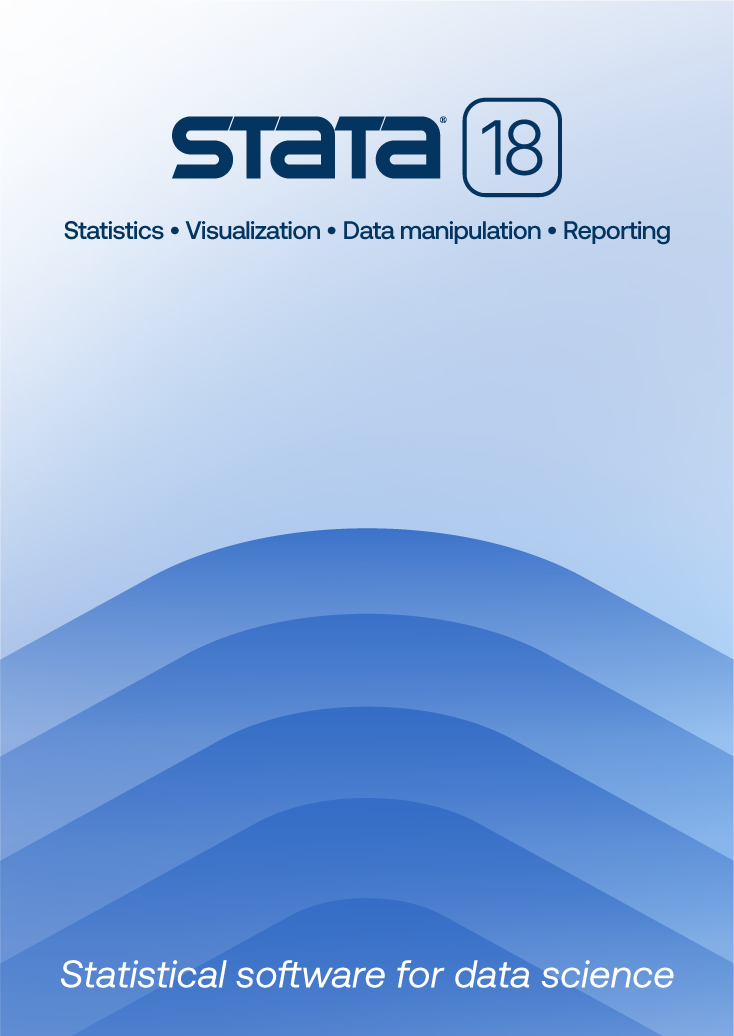
Regression Models for Categorical and Limited Dependent Variables |
||||||||||||||||||||||||||||||||||||||
![]() Click to enlarge See the back cover |
![]()
As an Amazon Associate, StataCorp earns a small referral credit from
qualifying purchases made from affiliate links on our site.
eBook not available for this title
eBook not available for this title |
|
||||||||||||||||||||||||||||||||||||
Comment from the Stata technical groupRegression Models for Categorical and Limited Dependent Variables, by J. Scott Long of Indiana University, is accessible to students and professionals alike. The author provides a unified treatment of the most prevalent and useful models for categorical and limited dependent variables. The book places a strong emphasis on model interpretation that is not found in most statistics texts. The mathematics is thorough but is complementary rather than focal. This is an excellent resource for an introduction to the categorical and limited dependent variables or as a desk reference. For Stata users, the book benefits greatly from the associated software. Dr. Long has developed and made available a suite of Stata commands to implement many of the techniques discussed in the book and programs to do the analyses that are contained in his book. The commands and programs can be accessed by pointing your web browser to http://www.indiana.edu/~jslsoc/spost.htm. They can also be installed directly in Stata with just a few net commands. |
||||||||||||||||||||||||||||||||||||||
Table of contentsView table of contents >> List of Figures
List of Tables
Series Editor’s Introduction
Abbreviations and Notation
1 Introduction
1.1. Linear and Nonlinear Models
1.2. Organization 1.3. Orientation 1.4. Bibliographic Notes 2 Continuous Outcomes: The Linear Regression Model
2.1. The Linear Regression Model
2.2. Interpreting Regression Coefficients 2.3. Estimation by Ordinary Least Squares 2.4. Nonlinear Linear Regression Models 2.5. Violations of the Assumptions 2.6. Maximum Likelihood Estimation 2.7. Conclusions 2.8. Bibliographic Notes 3 Binary Outcomes: The Linear Probability, Probit, and Logit Models
3.1. The Linear Probability Model
3.2. A Latent Variable Model for Binary Variables 3.3. Identification 3.4. A Nonlinear Probability Model 3.5. ML Estimation 3.6. Numerical Methods for ML Estimation 3.7. Interpretation 3.8. Interpretation Using Odds Ratios 3.9. Conclusions 3.10. Bibliographic Notes 4 Hypothesis Testing and Goodness of Fit
4.1. Hypothesis Testing
4.2. Residuals and Influence 4.3. Scalar Measures of Fit 4.4. Conclusions 4.5. Bibliographic Notes 5 Ordinal Outcomes: Ordered Logit and Ordered Probit Analysis
5.1. A Latent Variable Model for Ordinal Variables
5.2. Identification 5.3. Estimation 5.4. Interpretation 5.5. The Parallel Regression Assumption 5.6. Related Models for Ordinal Data 5.7. Conclusions 5.8. Bibliographic Notes 6 Nominal Outcomes: Multinomial Logit and Related Models
6.1. Introduction to the Multinomial Logit Model
6.2. The Multinomial Logit Model 6.3. ML Estimation 6.4. Computing and Testing Other Contrasts 6.5. Two Useful Tests 6.6. Interpretation 6.7. The Conditional Logit Model 6.8. Independence of Irrelevant Alternatives 6.9. Related Models 6.10. Conclusions 6.11. Bibliographic Notes 7 Limited Outcomes: The Tobit Model
7.1. The Problem of Censoring
7.2. Truncated and Censored Distributions 7.3. The Tobit Model for Censored Outcomes 7.4. Estimation 7.5. Interpretation 7.6. Extensions 7.7. Conclusions 7.8. Bibliographic Notes 8 Count Outcomes: Regression Models for Counts
8.1. The Poisson Distribution
8.2. The Poisson Regression Model 8.3. The Negative Binomial Regression Model 8.4. Models for Truncated Counts 8.5. Zero Modified Counted Models 8.6. Comparisons Among Count Models 8.7. Conclusions 8.8. Bibliographic Notes 9 Conclusions
9.1. Links Using Latent Variable Models
9.2. The Generalized Linear Model 9.3. Similarities Among Probability Models 9.4. Event History Analysis 9.5. Log-Linear Models A Answers to Exercises
References
Author Index
Subject Index
About the Author
|
Learn
NetCourses
Classroom and web training
Organizational training
Webinars
Video tutorials
Third-party courses
Web resources
Teaching with Stata
© Copyright 1996–2024 StataCorp LLC. All rights reserved.
×
We use cookies to ensure that we give you the best experience on our website—to enhance site navigation, to analyze usage, and to assist in our marketing efforts. By continuing to use our site, you consent to the storing of cookies on your device and agree to delivery of content, including web fonts and JavaScript, from third party web services.
Cookie Settings
Last updated: 16 November 2022
StataCorp LLC (StataCorp) strives to provide our users with exceptional products and services. To do so, we must collect personal information from you. This information is necessary to conduct business with our existing and potential customers. We collect and use this information only where we may legally do so. This policy explains what personal information we collect, how we use it, and what rights you have to that information.
These cookies are essential for our website to function and do not store any personally identifiable information. These cookies cannot be disabled.
This website uses cookies to provide you with a better user experience. A cookie is a small piece of data our website stores on a site visitor's hard drive and accesses each time you visit so we can improve your access to our site, better understand how you use our site, and serve you content that may be of interest to you. For instance, we store a cookie when you log in to our shopping cart so that we can maintain your shopping cart should you not complete checkout. These cookies do not directly store your personal information, but they do support the ability to uniquely identify your internet browser and device.
Please note: Clearing your browser cookies at any time will undo preferences saved here. The option selected here will apply only to the device you are currently using.