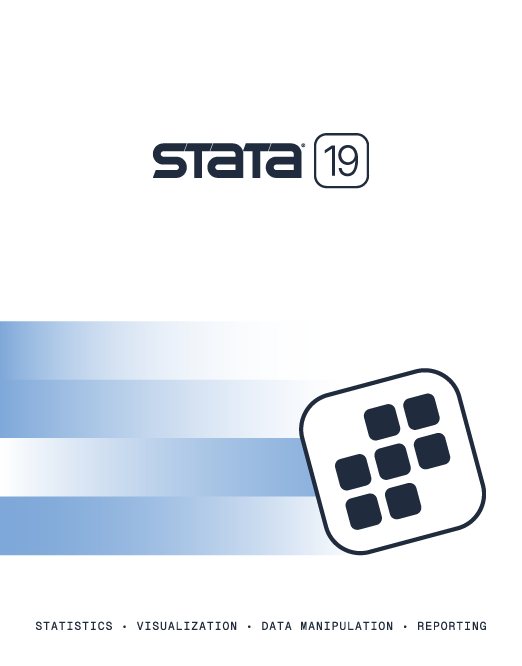
2025 Stata Conference • Nashville, TN • 31 July–01 August
Quantile Regression |
||||||||||||||||||||||||||||||||||||||
![]() Click to enlarge See the back cover |
![]()
As an Amazon Associate, StataCorp earns a small referral credit from
qualifying purchases made from affiliate links on our site.
eBook not available for this title
eBook not available for this title |
|
||||||||||||||||||||||||||||||||||||
Comment from the Stata technical groupQuantile Regression, by Lingxin Hao and Daniel Q. Naiman, provides an excellent introduction to quantile-regression methods. The intuitive explanations and many examples make this book easy to read and understand. An appendix provides Stata commands to replicate the examples using the datasets available at http://www.ams.jhu.edu/~hao/QRbook_data_codes/. After showing the advantages that quantile regression has over least squares, the authors discuss the estimation technique, the statistical inference, and how to interpret the results. The example-based approach is exceptionally clear and avoids swamping the reader in technical details. The final section of the monograph applies the techniques to changes in U.S. income equality between 1991 and 2001. This application illustrates both how to use the methods and how to interpret the results. |
||||||||||||||||||||||||||||||||||||||
Table of contentsView table of contents >> Series Editor’s Introduction
Acknowledgments
1. Introduction
2. Quantiles and Quantile Functions
CDFs, Quantiles, and Quantile Functions
Sampling Distribution of a Sample Quantile Quantile-Based Measures of Location and Shape Quantile as a Solution to a Certain Minimization Problem Properties of Quantiles Summary Note Chapter 2 Appendix: A Proof: Median and Quantiles as Solutions to a Minimization Problem 3. Quantile-Regression Model and Estimation
Linear-Regression Modeling and Its Shortcomings
Conditional-Median and Quantile-Regression Models QR Estimation Transformation and Equivariance Summary Notes 4. Quantile-Regression Inference
Standard Errors and Confidence Intervals for the LRM
Standard Errors and Confidence Intervals for the QRM The Bootstrap Method for the QRM Goodness of Fit of the QRM Summary Note 5. Interpretation of Quantile-Regression Estimates
Reference and Comparison
Conditional Means Versus Conditional Medians Interpretation of Other Individual Conditional Quantiles Tests for Equivalence of Coefficients Across Quantiles Using the QRM Results to Interpret Shape Shifts Summary Notes 6. Interpretation of Monotone-Transformed QRM
Location Shifts on the Log Scale
From Log Scale Back to Raw Scale Graphical View of Log-Scale Coefficients Shape-Shift Measures from Log-Scale Fits Summary Notes 7. Application to Income Inequality in 1991 and 2001
Observed Income Disparity
Descriptive Statistics Notes on Survey Income Data Goodness of Fit Conditional-Mean Versus Conditional-Median Regression Graphical View of QRM Estimates from Income and Log-Income Equations Quantile Regressions at Noncentral Positions: Effects in Absolute Terms Assessing a Covariate’s Effect on Location and Shape Shifts Summary Appendix: Stata Codes
References
Index
About the Authors
|
Learn
Free webinars
NetCourses
Classroom and web training
Organizational training
Video tutorials
Third-party courses
Web resources
Teaching with Stata
© Copyright 1996–2025 StataCorp LLC. All rights reserved.
×
We use cookies to ensure that we give you the best experience on our website—to enhance site navigation, to analyze usage, and to assist in our marketing efforts. By continuing to use our site, you consent to the storing of cookies on your device and agree to delivery of content, including web fonts and JavaScript, from third party web services.
Cookie Settings
Last updated: 16 November 2022
StataCorp LLC (StataCorp) strives to provide our users with exceptional products and services. To do so, we must collect personal information from you. This information is necessary to conduct business with our existing and potential customers. We collect and use this information only where we may legally do so. This policy explains what personal information we collect, how we use it, and what rights you have to that information.
These cookies are essential for our website to function and do not store any personally identifiable information. These cookies cannot be disabled.
This website uses cookies to provide you with a better user experience. A cookie is a small piece of data our website stores on a site visitor's hard drive and accesses each time you visit so we can improve your access to our site, better understand how you use our site, and serve you content that may be of interest to you. For instance, we store a cookie when you log in to our shopping cart so that we can maintain your shopping cart should you not complete checkout. These cookies do not directly store your personal information, but they do support the ability to uniquely identify your internet browser and device.
Please note: Clearing your browser cookies at any time will undo preferences saved here. The option selected here will apply only to the device you are currently using.