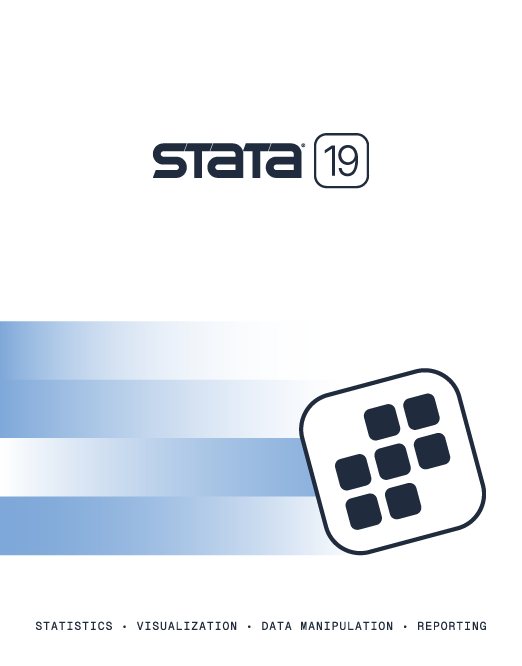
2025 Stata Conference • Nashville, TN • 31 July–01 August
Propensity Score Analysis: Statistical Methods and Applications, Second Edition |
||||||||||||||||||||||||||||||||||||
![]() Click to enlarge |
![]()
As an Amazon Associate, StataCorp earns a small referral credit from
qualifying purchases made from affiliate links on our site.
eBook not available for this title
eBook not available for this title |
|
||||||||||||||||||||||||||||||||||
Comment from the Stata technical groupThe second edition of Propensity Score Analysis by Shenyang Guo and Mark W. Fraser is an excellent book on estimating treatment effects from observational data. Researchers and graduate students interested in the analysis of observational data will find this book invaluable. This book is the first to provide step-by-step instructions for using Stata to estimate treatment effects by propensity-score analysis. In addition, this book also covers Heckman’s sample-selection estimator, nearest-neighbor matching estimators, propensity-score matching, and propensity-score nonparametric regression estimators. After providing a useful history of these techniques, the book includes a discussion on the counterfactual framework for estimating treatment effects involved in using observational data. The authors have consolidated the notation and theory found in the propensity-score literature, and this notation is carried throughout the text. This book introduces the philosophy and methods of matching estimation in an approachable manner, even for those unfamiliar with the subject. Readers embark on an interesting journey from a discussion on the early formulation of the Neyman–Rubin counterfactual framework to one on current methods and research issues. These ideas are discussed at theoretical and applied levels. The authors of the book formally describe the assumptions underlying the analyses to enlighten readers about the issues related to applying these techniques to practical problems. Propensity-score analysis has roots in both economics and statistics. Economists will find the discussion of Heckman’s sample-selection estimator familiar, and statisticians will find the Rosenbaum and Rubin counterfactual framework familiar. The authors do a great job of bringing these two viewpoints together in a unified, balanced manner. New to the second edition are sections on multivalued treatments, generalized propensity-score estimators, and enhanced sections on propensity-score weighting estimators. Most of the examples in this book use Stata, and many of the estimators discussed in this new edition are implemented in the teffects command available in Stata 13. While this book makes an excellent text for a graduate-level course on the analysis of observational data, it is readily accessible to researchers using observational data to estimate treatment effects. This book should be a required read for students in economics and statistics. |
||||||||||||||||||||||||||||||||||||
Table of contentsView table of contents >>
List of Tables
List of Figures Preface
1. What the Book Is About
2. New in the Second Edition 3. Acknowledgments
About the Authors
1. Introduction
1.1 Observational Studies
2. Counterfactual Framework and Assumptions
1.2 History and Development 1.3 Randomized Experiments
1.3.1 Fisher's Randomized Experiment
1.4 Why and When a Propensity Score Analysis Is Needed 1.3.2 Types of Randomized Experiments and Statistical Tests 1.3.3 Critiques of Social Experimentation 1.5 Computing Software Packages 1.6 Plan of the Book
2.1 Causality, Internal Validity, and Threats
3. Conventional Methods for Data Balancing
2.2 Counterfactuals and the Neyman-Rubin Counterfactual Framework 2.3 The Ignorable Treatment Assignment Assumption 2.4 The Stable Unit Treatment Value Assumption 2.5 Methods for Estimating Treatment Effects
2.5.1 Design of Observational Study
2.6 The Underlying Logic of Statistical Inference 2.5.2 The Seven Models 2.5.3 Other Balancing Methods 2.5.4 Instrumental Variables Estimator 2.5.5 Regression Discontinuity Designs 2.7 Types of Treatment Effects 2.8 Treatment Effect Heterogeneity
2.8.1 The Importance of Studying Treatment Effect Heterogeneity
2.9 Heckman's Econometric Model of Causality
2.10 Conclusion
2.8.2 Checking the Plausability of the Unconfoundedness Assumption 2.8.3 A Methodological Note About the Hausman Test of Endogeneity 2.8.4 Tests of Treatment Effect Heterogeneity 2.8.5 Example
3.1 Why Is Data Balancing Necessary? A Heuristic Example
4. Sample Selection and Related Models
3.2 Three Methods for Data Balancing
3.2.1 The Ordinary Least Squares Regression
3.3 Design of the Data Simulation 3.2.2 Matching 3.2.3 Stratification 3.4 Results of the Data Simulation 3.5 Implications of the Data Simulation 3.6 Key Issues Regarding the Application of OLS Regression 3.7 Conclusion
4.1 The Sample Selection Model
5. Propensity Score Matching and Related Models
4.1.1 Truncation, Censoring, and Incidental Truncation
4.2 Treatment Effect Model 4.1.2 Why Is It Important to Model Sample Selection? 4.1.3 Moments of an Incidentally Truncated Bivariate Normal Distribution 4.1.4 The Heckman Model and Its Two-Step Estimator 4.3 Overview of the Stata Programs and Main Features of treatreg 4.4 Examples
4.4.1 Application of the Treatment Effect model to Analysis of Observational Data
4.5 Conclusion
4.4.2 Evaluation of Treatment Effects from a Program With a Group Randomization Design 4.4.3 Running the Treatment Effect Model After Multiple Imputations of Missing Data
5.1 Overview
6. Propensity Score Subclassification
5.2 The Problem of Dimensionality and the Properties of Propensity Scores 5.3 Estimating Propensity Scores
5.3.1 Binary Logistic Regression
5.4 Matching
5.3.2 Strategies to Specify a Correct Model—Predicting Propensity Scores 5.3.3 Hirano and Imbens's Method for Specifying Predictors Relying on Predetermined Critical t Values 5.3.4 Generalized Boosted Modeling
5.4.1 Greedy Matching
5.5 Postmatching Analysis
5.4.2 Optimal Matching 5.4.3 Fine Balance
5.5.1 Multivariate Analysis After Greedy Matching
5.6 Propensity Score Matching With Multilevel Data
5.5.2 Computing Indices of Covariate Imbalance 5.5.3 Outcome Analysis Using the Hodges-Lehmann Aligned Rank Test After Optimal Matching 5.5.4 Regression Adjustment Based on Sample Created by Optimal Pair Matching 5.5.5 Regression Adjustment Using Hodges-Lehmann Aligned Rank Scores After Optimal Matching
5.6.1 Overview of Statistical Approaches to Multilevel Data
5.7 Overview of the Stata and R Programs 5.6.2 Perspectives Extending the Propensity Score Analysis to the Multilevel Modeling 5.6.3 Estimation of the Propensity Scores Under the Context of Multilevel Modeling 5.6.4 Multilevel Outcome Analysis 5.8 Examples
5.8.1 Greedy Matching and Subsequent Analysis of Hazard Rates
5.9 Conclusion
5.8.2 Optimal Matching 5.8.3 Post-Full Matching Analysis Using the Hodges-Lehmann Aligned Rank Test 5.8.4 Post-Pair Matching Analysis Using Regression of Difference Scores 5.8.5 Multilevel Propensity Score Analysis 5.8.6 Comparison of Rand-gbm and Stata's boost Algorithms
6.1 Overview
7. Propensity Score Weighting
6.2 The Overlap Assumption and Methods to Address Its Violation 6.3 Structural Equation Modeling With Propensity Score Subclassification
6.3.1 The Need for Integrating SEM and Propensity Score Modeling Into One Analysis
6.4 The Stratification-Multilevel Method 6.3.2 Kaplan's (1999) Work to Integrate Propensity Score Subclassification With SEM 6.3.3 Conduct SEM With Propensity Score Subclassification 6.5 Examples
6.5.1 Stratification After Greedy Matching
6.6 Conclusion
6.5.2 Subclassification Followed by a Cox Proportional Hazards Model 6.5.3 Propensity Score Subclassification in Conjunction with SEM
7.1 Overview
8. Matching Estimators
7.2 Weighting Estimators
7.2.1 Formulas for Creating Weights to Estimate ATE and ATT
7.3 Examples
7.2.2 A Corrected Version of Weights Estimating ATE 7.2.3 Steps in Propensity Score Weighting
7.3.1 Propensity Score Weighting With a Multiple Regression Outcome Analysis
7.4 Conclusion
7.3.2 Propensity Score Weighting With a Cox Proportional Hazards Model 7.3.3 Propensity Score Weighting With an SEM 7.3.4 Comparison of Models and Conclusions of the Study of the Impact of Poverty on Child Academic Achievement
8.1 Overview
9. Propensity Score Analysis With Nonparametric Regression
8.2 Methods of Matching Estimators
8.2.1 Simple Matching Estimators
8.3 Overview of the Stata Program nnmatch 8.2.2 Bias-Corrected Matching Estimator 8.2.3 Variance Estimator Assuming Homoscedasticity 8.2.4 Variance Estimator Allowing for Heteroscedasticity 8.2.5 Large Sample Properties and Correction 8.4 Examples
8.4.1 Matching With Bias-Corrected and Robust Variance Estimators
8.5 Conclusion
8.4.2 Efficacy Subset Analysis With Matching Estimators
9.1 Overview
10. Propensity Score Analysis of Categorical or Continuous Treatments: Dosage Analyses
9.2 Methods of Propensity Score Analysis With Nonparametric Regression
9.2.1 The Kernel-Based Matching Estimators
9.3 Overview of the Stata Programs psmatch2 and bootstrap 9.2.2 Review of the Basic Concepts of Local Linear Regression (lowess) 9.2.3 Asymptotic and Finite-Sample Properties of Kernel and Local Linear Matching 9.4 Examples
9.4.1 Analysis of Difference-in-Differences
9.5 Conclusion
9.4.2 Application of Kernel-Based Matching to One-Point Data
10.1 Overview
11. Selection Bias and Sensitivity Analysis
10.2 Modeling Doses With a Single Scalar Balancing Score Estimated by an Ordered Logistic Regression 10.3 Modeling Doses With Multiple Balancing Scores Estimated by a Multinomial Logit Model 10.4 The Generalized Propensity Score Estimator 10.5 Overview of the Stata gpscore Program 10.6 Examples
10.6.1 Modeling Doses of Treatment With Multiple Balancing Scores Estimated by a Multinomial Logit Model
10.7 Conclusion
10.6.2 Modeling Doses of Treatment With the Generalized Propensity Score Estimator
11.1 Selection Bias: An Overview
12. Concluding Remarks
11.1.1 Sources of Selection Bias
11.2 A Monte Carlo Study Comparing Corrective Models
11.1.2 Overt Bias Versus Hidden Bias 11.1.3 Consequences of Selection Bias 11.1.4 Strategies to Correct for Selection Bias
11.2.1 Design of the Monte Carlo Study
11.3 Rosenbaum's Sensitivity Analysis
11.2.2 Results of the Monte Carlo Study 11.2.3 Implications
11.3.1 The Basic Idea
11.4 Overview of the Stata Program rbounds 11.3.2 Illustration of Wilcoxon's Signed Rank Test for Sensitivity Analysis of a Matched Pair Study 11.5 Examples
11.5.1 Sensitivity Analysis of the Effects of Lead Exposure
11.6 Conclusion
11.5.2 Sensitivity Analysis for the Study Using Pair Matching
12.1 Common Pitfalls in Observational Studies: A Checklist for Critical Review
12.2 Approximating Experiments With Propensity Score Approaches
12.2.1 Criticism of Propensity Score Methods
12.3 Other Advances in Modeling Causality 12.2.2 Regression and Propensity Score Approaches: Do They Provide Similar Results? 12.2.3 Criticism of Sensitivity Analysis (Γ) 12.2.4 Group Randomized Trials 12.4 Directions for Future Development
References
Index |
Learn
Free webinars
NetCourses
Classroom and web training
Organizational training
Video tutorials
Third-party courses
Web resources
Teaching with Stata
© Copyright 1996–2025 StataCorp LLC. All rights reserved.
×
We use cookies to ensure that we give you the best experience on our website—to enhance site navigation, to analyze usage, and to assist in our marketing efforts. By continuing to use our site, you consent to the storing of cookies on your device and agree to delivery of content, including web fonts and JavaScript, from third party web services.
Cookie Settings
Last updated: 16 November 2022
StataCorp LLC (StataCorp) strives to provide our users with exceptional products and services. To do so, we must collect personal information from you. This information is necessary to conduct business with our existing and potential customers. We collect and use this information only where we may legally do so. This policy explains what personal information we collect, how we use it, and what rights you have to that information.
These cookies are essential for our website to function and do not store any personally identifiable information. These cookies cannot be disabled.
This website uses cookies to provide you with a better user experience. A cookie is a small piece of data our website stores on a site visitor's hard drive and accesses each time you visit so we can improve your access to our site, better understand how you use our site, and serve you content that may be of interest to you. For instance, we store a cookie when you log in to our shopping cart so that we can maintain your shopping cart should you not complete checkout. These cookies do not directly store your personal information, but they do support the ability to uniquely identify your internet browser and device.
Please note: Clearing your browser cookies at any time will undo preferences saved here. The option selected here will apply only to the device you are currently using.