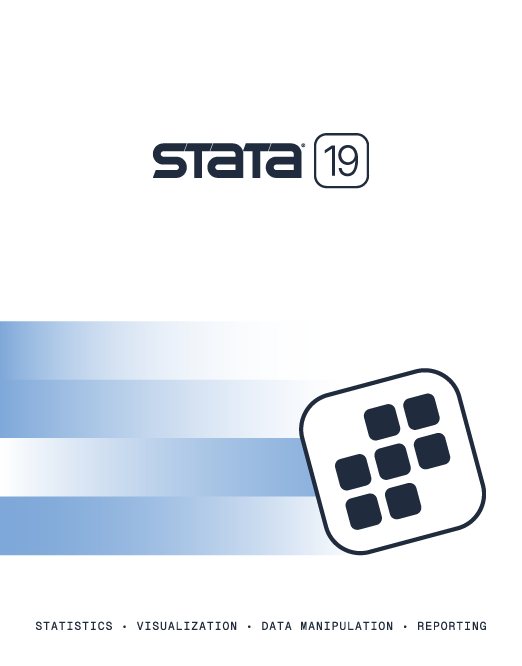
2025 Stata Conference • Nashville, TN • 31 July–01 August
Practical Multivariate Analysis, Sixth Edition |
||||||||||||||||||||||||||||||||
![]() Click to enlarge See the back cover |
![]()
As an Amazon Associate, StataCorp earns a small referral credit from
qualifying purchases made from affiliate links on our site.
eBook not available for this title
eBook not available for this title |
|
||||||||||||||||||||||||||||||
Comment from the Stata technical groupComments about the Practical Multivariate Analysis, Fifth Edition: The fifth edition of Practical Multivariate Analysis, by Afifi, May, and Clark, provides an applied introduction to the analysis of multivariate data. The preface says: “We wrote this book for investigators, specifically behavioral scientists, biomedical scientists, and industrial or academic researchers, who wish to perform multivariate statistical analyses and understand the results. We expect readers to be able to perform and understand the results, but also expect them to know when to ask for help from an expert on the subject. It can either be used as a self-guided textbook or as a text in an applied course in multivariate analysis. Sections 1 and 2, the first half of the book, review the basics: understanding the different types of data, preparing your data, selecting appropriate statistical techniques, and using and understanding regression and correlation techniques. Section 3, the second half of the book, covers canonical correlation, discriminant analysis, logistic regression, survival analysis, principal components, factor analysis, cluster analysis, log-linear analysis, and correlated outcomes regression (think xtmixed in Stata). The applied introductory nature of the book can be seen in the table of contents. Most chapters include subsections titled “Chapter outline”, “When is [this technique] used”, “Data example”, “Basic concepts”, “Discussion of computer programs”, “What to watch out for”, “Summary”, and “Problems”. The UCLA website, http://www.ats.ucla.edu/stat/examples/cama4 is another resource for readers of this book. Here many of the examples that were in the fourth edition of the book are demonstrated in Stata and in four other statistical packages. The data for the fifth edition are available for download from within Stata so that you can practice applying the techniques as you read. If you are looking for derivations and proofs, this book is not for you. If you are looking for guidance on techniques to use, when to use them, and how to interpret what they produce, this book will prove helpful. |
||||||||||||||||||||||||||||||||
Table of contentsView table of contents >> Preface
Authors
I. Preparation for Analysis
1. What is multivariate analysis?
1.1 Defining multivariate analysis
1.2 Examples of multivariate analyses 1.3 Exploratory versus confirmatory analyses 1.4 Multivariate analyses discussed in this book 1.5 Organization and content of the book 2. Characterizing data for analysis
2.1 Variables: Their definition, classification, and use
2.2 Defining statistical variables 2.3 Stevens’s classification of variables 2.4 How variables are used in data analysis 2.5 Examples of classifying variables 2.6 Other characteristics of data 2.7 Summary 2.8 Problems 3. Preparing for data analysis
3.1 Processing data so they can be analyzed
3.2 Choice of a statistical package 3.3 Techniques for data entry 3.4 Organizing the data 3.5 Reproducible research and literate programming 3.6 Example: depression study 3.7 Summary 3.8 Problems 4. Data visualization
4.1 Introduction
4.2 Univariate data 4.3 Bivariate data 4.4 Multivariate data 4.5 Discussion of computer programs 4.6 What to watch out for 4.7 Summary 4.8 Problems 5. Data screening and transformations
5.1 Transformations, assessing normality and independence
5.2 Common transformations 5.3 Selecting appropriate transformations 5.4 Assessing independence 5.5 Discussion of computer programs 5.6 Summary 5.7 Problems 6. Selecting appropriate analyses
6.1 Which analyses to perform?
6.2 Why selection is often difficult 6.3 Appropriate statistical measures 6.4 Selecting appropriate multivariate analyses 6.5 Summary 6.6 Problems II. Regression Analysis
7. Simple regression and correlation
7.1 Chapter outline
7.2 When are regression and correlation used? 7.3 Data example 7.4 Regression methods: fixed-X case 7.5 Regression and correlation: variable-X case 7.6 Interpretation: fixed-X case 7.7 Interpretation: variable-X case 7.8 Other available computer output 7.9 Robustness and transformations for regression 7.10 Other types of regression 7.11 Special applications of regression 7.12 Discussion of computer programs 7.13 What to watch out for 7.14 Summary 7.15 Problems 8. Multiple regression and correlation
8.1 Chapter outline
8.2 When are regression and correlation used? 8.3 Data example 8.4 Regression methods: fixed-X case 8.5 Regression and correlation: variable-X case 8.6 Interpretation: fixed-X case 8.7 Interpretation: variable-X case 8.8 Regression diagnostics and transformations 8.9 Other options in computer programs 8.10 Discussion of computer programs 8.11 What to watch out for 8.12 Summary 8.13 Problems 9. Variable selection in regression
9.1 Chapter outline
9.2 When are variable selection methods used? 9.3 Data example 9.4 Criteria for variable selection 9.5 A general F test 9.6 Stepwise regression 9.7 Lasso regression 9.8 Discussion of computer programs 9.9 Discussion of strategies 9.10 What to watch out for 9.11 Summary 9.12 Problems 10. Special regression topics
10.1 Chapter outline
10.2 Missing values in regression analysis 10.3 Dummy variables 10.4 Constraints on parameters 10.5 Regression analysis with multicollinearity 10.6 Ridge regression 10.7 Summary 10.8 Problems 11. Discriminant analysis
11.1 Chapter outline
11.2 When is discriminant analysis used? 11.3 Data example 11.4 Basic concepts of classification 11.5 Theoretical background 11.6 Interpretation 11.7 Adjusting the dividing point 11.8 How good is the discrimination? 11.9 Testing variable contributions 11.10 Variable selection 11.11 Discussion of computer programs 11.12 What to watch out for 11.13 Summary 11.14 Problems 12. Logistic regression
12.1 Chapter outline
12.2 When is logistic regression used? 12.3 Data example 12.4 Basic concepts of logistic regression 12.5 Interpretation: categorical variables 12.6 Interpretation: continuous variables 12.7 Interpretation: interactions 12.8 Refining and evaluating logistic regression 12.9 Nominal and ordinal logistic regression 12.10 Applications of logistic regression 12.11 Poisson regression 12.12 Discussion of computer programs 12.13 What to watch out for 12.14 Summary 12.15 Problems 13. Regression analysis with survival data
13.1 Chapter outline
13.2 When is survival analysis used? 13.3 Data examples 13.4 Survival functions 13.5 Common survival distributions 13.6 Comparing survival among groups 13.7 The log-linear regression model 13.8 The Cox regression model 13.9 Comparing regression models 13.10 Discussion of computer programs 13.11 What to watch out for 13.12 Summary 13.13 Problems 14. Principal components analysis
14.1 Chapter outline
14.2 When is principal components analysis used? 14.3 Data example 14.4 Basic concepts 14.5 Interpretation 14.6 Other uses 14.7 Discussion of computer programs 14.8 What to watch out for 14.9 Summary 14.10 Problems 15. Factor analysis
15.1 Chapter outline
15.2 When is factor analysis used? 15.3 Data example 15.4 Basic concepts 15.5 Initial extraction: principal components 15.6 Initial extraction: iterated components 15.7 Factor rotations 15.8 Assigning factor scores 15.9 Application of factor analysis 15.10 Discussion of computer programs 15.11 What to watch out for 15.12 Summary 15.13 Problems 16. Cluster analysis
16.1 Chapter outline
16.2 When is cluster analysis used? 16.3 Data example 16.4 Basic concepts: initial analysis 16.5 Analytical clustering techniques 16.6 Cluster analysis for financial data set 16.7 Discussion of computer programs 16.8 What to watch out for 16.9 Summary 16.10 Problems 17. Log-linear analysis
17.1 Chapter outline
17.2 When is log-linear analysis used? 17.3 Data example 17.4 Notation and sample considerations 17.5 Tests and models for two-way tables 17.6 Example of a two-way table 17.7 Models for multiway tables 17.8 Exploratory model building 17.9 Assessing specific models 17.10 Sample size issues 17.11 The logit model 17.12 Discussion of computer programs 17.13 What to watch out for 17.14 Summary 17.15 Problems 18. Correlated outcomes regression
18.1 Chapter outline
18.2 When is correlated outcomes regression used? 18.3 Data examples 18.4 Basic concepts 18.5 Regression of clustered data with a continuous outcome 18.6 Regression of clustered data with a binary outcome 18.7 Regression of longitudinal data 18.8 Generalized estimating equations analysis of correlated data 18.9 Discussion of computer programs 18.10 What to watch out for 18.11 Summary 18.12 Problems Appendix A
A.1 Data sets and how to obtain them
A.2 Chemical companies' financial data A.3 Depression study data A.4 Financial performance cluster-analysis data A.5 Lung cancer survival data A.6 Lung function data A.7 Paternal HIV data A.8 Northridge earthquake data A.9 School data A.10 Mice data Bibliography
Index
|
Learn
Free webinars
NetCourses
Classroom and web training
Organizational training
Video tutorials
Third-party courses
Web resources
Teaching with Stata
© Copyright 1996–2025 StataCorp LLC. All rights reserved.
×
We use cookies to ensure that we give you the best experience on our website—to enhance site navigation, to analyze usage, and to assist in our marketing efforts. By continuing to use our site, you consent to the storing of cookies on your device and agree to delivery of content, including web fonts and JavaScript, from third party web services.
Cookie Settings
Last updated: 16 November 2022
StataCorp LLC (StataCorp) strives to provide our users with exceptional products and services. To do so, we must collect personal information from you. This information is necessary to conduct business with our existing and potential customers. We collect and use this information only where we may legally do so. This policy explains what personal information we collect, how we use it, and what rights you have to that information.
These cookies are essential for our website to function and do not store any personally identifiable information. These cookies cannot be disabled.
This website uses cookies to provide you with a better user experience. A cookie is a small piece of data our website stores on a site visitor's hard drive and accesses each time you visit so we can improve your access to our site, better understand how you use our site, and serve you content that may be of interest to you. For instance, we store a cookie when you log in to our shopping cart so that we can maintain your shopping cart should you not complete checkout. These cookies do not directly store your personal information, but they do support the ability to uniquely identify your internet browser and device.
Please note: Clearing your browser cookies at any time will undo preferences saved here. The option selected here will apply only to the device you are currently using.