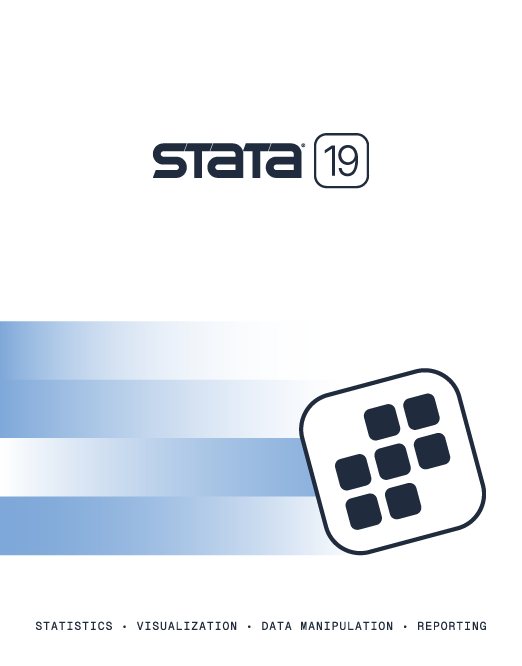
2025 Stata Conference • Nashville, TN • 31 July–01 August
Methods for Meta-Analysis in Medical Research |
||||||||||||||||||||||||||||||||||||
![]() Click to enlarge See the back cover |
![]()
As an Amazon Associate, StataCorp earns a small referral credit from
qualifying purchases made from affiliate links on our site.
eBook not available for this title
eBook not available for this title |
|
||||||||||||||||||||||||||||||||||
Comment from the Stata technical groupMeta-analysis as a statistical and scientific tool has grown immensely in popularity over the last decade as a way to systematically present new research results in the proper context, given all previous related work. This text covers much of the statistical methodology used by meta-analysts, from the most basic to the advanced, and is ideal for self-study as it requires little background knowledge of statistics. The authors do a good job of presenting their concepts free of mathematical theory, which is of little use to the practicing researcher. The book begins in part A with some basic meta-analysis terminology and biostatistical terms, such as odds, odds ratios, and relative risks. Part A then continuous with a discussion of heterogeneity, the application of fixed-effects and random-effects methods for combining study estimates, publication bias, sensitivity analysis, and graphical techniques. Part B covers Bayesian methods (which fit naturally with the concept of meta-analysis), the meta-analysis of individual patient data, missing data, the meta-analysis of nonstandard data types, multiple and correlated outcome measures, observational studies, survival data, and miscellaneous topics. |
||||||||||||||||||||||||||||||||||||
Table of contentsView table of contents >> Preface
Acknowledgments
Part A: Meta-Analysis Methodology: The Basics
1 Introduction—Meta-analysis: Its Development and Uses
1.1 Evidence-based health care
1.2 Evidence-based everything! 1.3 Pulling together the evidence—systematic reviews 1.4 Why meta-analysis? 1.5 Aim of this book 1.6 Concluding remarks References 2 Defining Outcome Measures used for Combining via Meta-analysis
2.1 Introduction
2.2 Non-comparative binary outcomes
2.2.1 Odds
2.3 Comparative binary outcomes2.2.2 Incidence rates
2.3.1 The Odds ratio
2.4 Continuous data2.3.2 Relative risk (or rate ratio/relative rate) 2.3.3 Risk differences between proportions (or the absolute risk reduction) 2.3.4 The number needed to treat 2.3.5 Comparisons of rates 2.3.6 Other scales of measurement used in summarizing binary data 2.3.7 Which scale to use?
2.4.1 Outcomes defined on their original metric (mean difference)
2.5 Ordinal outcomes2.4.2 Outcomes defined using standardized mean differences 2.6 Summary/Discussion References 3 Assessing Between Study Heterogeneity
3.1 Introduction
3.2 Hypothesis tests for presence of heterogeneity
3.2.1 Standard x2 test
3.3 Graphical informal tests/explorations of heterogeneity3.2.2 Extensions/alternative tests 3.2.3 Example: Testing for heterogeneity in the cholesterol lowering trial dataset
3.3.1 Plot of normalized (z) scores
3.4 Possible causes of heterogeneity3.3.2 Forest plot 3.3.3 Radial plot (Galbraith diagram) 3.3.4 L'Abbé plot
3.4.1 Specific factors that may cause heterogeneity in RCTs
3.5 Methods for investigating and dealing with sources of heterogeneity
3.5.1 Change scale of outcome variable
3.6 The validity of pooling studies with heterogeneous outcomes3.5.2 Include covariates in a regression model (meta-regression) 3.5.3 Exclude studies 3.5.4 Analyse groups of studies separately 3.5.5 Use of random effects models 3.5.6 Use of mixed-effect models 3.7 Summary/Discussion References 4 Fixed Effects Methods for Combining Study Estimates
4.1 Introduction
4.2 General fixed effect model — the inverse variance-weighted method
4.2.1 Example: Combining odds ratios using the inverse variance-weighted method
4.3 Specific methods for combining odds ratios4.2.2 Example: Combining standardized mean differences using a continuous outcome scale
4.3.1 Mantel–Haenszel method for combining odds ratios
4.4 Summary/Discussion4.3.2 Peto's method for combining odds ratios 4.3.3 Combining odds ratios via maximum-likelihood techniques 4.3.4 Exact methods of interval estimation 4.3.5 Discussion of the relative merits of each method References 5 Random Effects Models for Combining Study Estimates
5.1 Introduction
5.2 Algebraic derivation for random effects models by the weighted method 5.3 Maximum likelihood and restricted maximum likelihood estimate solutions 5.4 Comparison of estimation method 5.5 Example: Combining the cholesterol lowering trials using a random effects model 5.6 Extensions to the random effects model
5.6.1 Including uncertainty induced by estimating the between study variance
5.7 Comparison of random with fixed effect models5.6.2 Exact approach to random effects meta-analysis of binary data 5.6.3 Miscellaneous extensions to the random effects model 5.8 Summary/Discussion References 6 Exploring Between Study Heterogeneity
6.1 Introduction
6.2 Subgroup analyses
6.2.1 Example: Stratification by study characteristics
6.3 Regression models for meta-analysis6.2.2 Example: Stratification by patient characteristics
6.3.1 Meta-regression models (fixed-effects regression)
6.4 Summary/Discussion6.3.2 Meta-regression example: a meta-analysis of Bacillus Calmette-Guérin (BCG) vaccine for the prevention of tuberculosis (TB) 6.3.3 Mixed effect models (random-effects regression) 6.3.4 Mixed model example: A re-analysis of Bacillus Calmette-Guérin (BCG) vaccine for the prevention of tuberculosis (TB) trials 6.3.5 Mixed modelling extensions References 7 Publication Bias
7.1 Introduction
7.2 Evidence of publication and related bias
7.2.1 Survey of authors
7.3 The seriousness and consequences of publication bias for meta-analysis7.2.2 Published versus registered trials in a meta-analysis 7.2.3 Follow-up of cohorts of registered studies 7.2.4 Non-empirical evidence 7.2.5 Evidence of language bias 7.4 Predictors of publication bias (factors effecting the probability a study will get published) 7.5 Identifying publication bias in a meta-analysis
7.5.1 The funnel plot
7.6 Taking into account publication bias or adjusting the results of a meta-analysis in the presence of publication bias7.5.2 Rank correlation test 7.5.3 Linear regression test 7.5.4 Other methods to detect publication bias 7.5.5 Practical advice on methods for detecting publication bias
7.6.1 Analysing only the largest studies
7.7 Broader perspective solutions to publication bias7.6.2 Rosenthal's ‘file drawer’ method 7.6.3 Models which estimate the number of unpublished studies, but do not adjust 7.6.4 Selection models using weighted distribution theory 7.6.5 The ‘Trim and Fill’ method 7.6.6 The sensitivity approach of Copas
7.7.1 Prospective registration of trials
7.8 Including unpublished information7.7.2 Changes in publication process and journals 7.9 Summary/Discussion References 8 Study Quality
8.1 Introduction
8.2 Methodological factors that may affect the quality of studies
8.2.1 Experimental studies
8.3 Incorporating study quality into a meta-analysis8.2.2 Observational Studies
8.3.1 Graphical plot
8.4 Practical implementation8.3.2 Cumulative methods 8.3.3 Regression model 8.3.4 Weighting 8.3.5 Excluding studies 8.3.6 Sensitivity analysis 8.5 Summary/Discussion References 9 Sensitivity Analysis
9.1 Introduction
9.2 Sensitivity of results to inclusion criteria 9.3 Sensitivity of results to meta-analytic methods
9.3.1 Assessing the impact of choice of study weighting
9.4 Summary/DiscussionReferences 10 Reporting the Results of a Meta-analysis
10.1 Introduction
10.2 Overview and structure of a report 10.3 Graphical displays used for reporting the findings of a meta-analysis
10.3.1 Forest plots
10.4 Summary/Discussion10.3.2 Radial plots 10.3.3 Funnel plots 10.3.4 Displaying the distribution of effect size estimates 10.3.5 Graphs investigating length of follow-up References Part B: Advanced and Specialized Meta-analysis Topics
11 Bayesian Methods in Meta-analysis
11.1 Introduction
11.2 Bayesian methods in health research
11.2.1 General introduction
11.3 Bayesian meta-analysis of normally distributed data 11.2.2 General advantages/disadvantages of Bayesian methods 11.2.3 Example: Bayesian analysis of a single trial using a normal conjugate model
11.3.1 Example: Combining trials with continuous outcome measures using Bayesian methods
11.4 Bayesian meta-analysis of binary data
11.4.1 Example: Combining binary outcome measures using Bayesian methods
11.5 Empirical Bayes methods in meta-analysis11.6 Advantages/disadvantages of Bayesian methods in met-analysis
11.6.1 Advantages
11.7 Extensions and specific areas of application11.6.2 Disadvantages
11.7.1 Incorporating study quality
11.8 Summary/Discussion11.7.2 Inclusion of covariates 11.7.3 Model selection 11.7.4 Hierarchical models 11.7.5 Sensitivity analysis 11.7.6 Comprehensive modelling 11.7.7 Other developments References 12 Meta-analysis of Individual Patient Data
12.1 Introduction
12.2 Procedural methodology
12.2.1 Data collection
12.3 Issues involved in carrying out IPD meta-analyses12.2.2 Checking data 12.4 Comparing meta-analysis using IPD or summary data? 12.5 Combining individual patient and summary data 12.6 Summary/Discussion References 13 Missing Data
13.1 Introduction
13.2 Reasons for missing data 13.3 Categories of missing data at the study level 13.4 Analytic methods for dealing with missing data
13.4.1 General missing data methods which can be applied in the meta-analysis context
13.5 Bayesian methods for missing data13.4.2 Missing data methods specific to meta-analysis 13.4.3 Example: Dealing with missing standard deviations of estimated in a meta-analysis 13.6 Summary/Discussion References 14 Meta-analysis of Different Types of Data
14.1 Introduction
14.2 Combining ordinal data 14.3 Issues concerning scales of measurement when combining data
14.3.1 Transforming scales, maintaining same data type
14.4 Meta-analysis of diagnostic test accuracy14.3.2 Binary outcome data reported on different scales 14.3.3 Combining studies whose outcomes are reported using different data types 14.3.4 Combining summaries of binary outcomes with those of continuous outcomes 14.3.5 Non-parametric method of combining different data type effect measures
14.4.1 Combining binary test results
14.5 Meta-analysis using surrogate markers14.4.2 Combining ordered categorical test results 14.4.3 Combining continuous test results 14.6 Combining a number of cross-over trials using the patient preference outcome 14.7 Vote-counting methods 14.8 Combining p-values/significance levels
14.8.1 Minimum p method
14.9 Novel applications of meta-analysis using non-standard methods or data14.8.2 Sum of z's method 14.8.3 Sum of logs method 14.8.4 Logit method 14.8.5 Other methods of combining significance levels 14.8.6 Appraisal of the methods 14.8.7 Example of combining p-values 14.10 Summary/Discussion References 15 Meta-analysis of Multiple and Correlated Outcome Measures
15.1 Introduction
15.2 Combining multiple p-values 15.3 Method for reducing multiple outcomes to a single measure for each study 15.4 Development of a multivariate model
15.4.1 Model of Raudenbush et al.
15.5 Summary/Discussion15.4.2 Model of Gleser and Olkin 15.4.3 Multiple outcome model for clinical trials 15.4.4 Random effect multiple outcome regression model 15.4.5 DuMouchel's extended model for multiple outcomes 15.4.6 Illustration of the use of multiple outcome models References 16 Meta-analysis of Epidemiological and Other Observational Studies
16.1 Introduction
16.2 Extraction and derivation of study estimates
16.2.1 Scales of measurement used to report and combine observational studies
16.3 Analysis of summary data16.2.2 Data manipulation for data extraction 16.2.3 Methods for transforming and adjusting reported results
16.3.1 Heterogeneity of observational studies
16.4 Reporting the results of meta-analysis of observational studies16.3.2 Fixed or random effects? 16.3.3 Weighting of observational studies 16.3.4 Methods for combining estimates of observations studies 16.3.5 Dealing with heterogeneity and combining the OC and breast cancer studies 16.5 Use of sensitivity and influence analysis 16.6 Study quality considerations for observational studies 16.7 Other issues concerning meta-analysis of observational studies
16.7.1 Analysing individual patient data from observational studies
16.8 Unresolved issues concerning the meta-analysis of observational studies16.7.2 Combining dose-response data 16.7.3 Meta-analysis of single case research 16.9 Summary/Discussion References 17 Generalized Synthesis of Evidence—Combining Different Sources of Evidence
17.1 Introduction
17.2 Incorporating single-arm studies: models for incorporating historical controls
17.2.1 Example
17.3 Combining matched and unmatched data17.4 Approaches for combining studies containing multiple and/or different treatment arms
17.4.1 Approach of Gleser and Olkin
17.5 The confidence profile method17.4.2 Models of Berkey et al. 17.4.3 Method of Higgins 17.4.4 Mixed model of DuMouchel 17.6 Cross-design synthesis
17.6.1 Beginnings
17.7 Summary/Discussion17.6.2 Bayesian hierarchical models 17.6.3 Grouped random effects models of Larose and Dey 17.6.4 Synthesizing studies with disparate designs to assess the exposure effects on the incidence of a rare adverse event 17.6.5 Combining the results of cancer studies in humans and other species 17.6.6 Combining biochemical and epidemiological evidence 17.6.7 Combining information from disparate toxicological studies using stratified original regression References 18 Meta-analysis of Survival Data
18.1 Introduction
18.2 Inferring/estimating and combining (log) hazard ratios 18.3 Calculation of the ‘log-rank’ odds ratio 18.4 Calculation of pooled survival rates 18.5 Method of Hunink and Wong 18.6 Iterative generalized least squares for meta-analysis of survival data at multiple times
18.6.1 Application of the model
18.7 Identifying prognostic factors using a log (relative risk) measure18.8 Combining quality of life adjusted survival data 18.9 Meta-analysis of survival data using individual patient data
18.9.1 Pooling independent samples of survival data to form an estimator of the common survival function
18.10 Summary/Discussion18.9.2 Is obtaining and using survival data necessary? References 19 Cumulative Meta-analysis
19.1 Introduction
19.2 Example: Ordering by date of publication 19.3 Using study characteristics other than date of publication
19.3.1 Example: Ordering the cholesterol trials by baseline risk in the control group
19.4 Bayesian approaches19.5 Issues regarding uses of cumulative meta-analysis 19.6 Summary/Discussion References 20 Miscellaneous and Developing Areas of Application in Meta-analysis
20.1 Introduction
20.2 Alternatives to conventional meta-analysis
20.2.1 Estimating and extrapolating a response surface
20.3 Developing areas20.2.2 Odd man out method 20.2.3 Best evidence synthesis
20.3.1 Prospective meta-analysis
References 20.3.2 Economic evaluation through meta-analysis 20.3.3 Combining meta-analysis and decision analysis 20.3.4 Net benefit model synthesizing disparate sources of information Appendix I: Software Used for the Examples in this Book
Subject Index
|
Learn
Free webinars
NetCourses
Classroom and web training
Organizational training
Video tutorials
Third-party courses
Web resources
Teaching with Stata
© Copyright 1996–2025 StataCorp LLC. All rights reserved.
×
We use cookies to ensure that we give you the best experience on our website—to enhance site navigation, to analyze usage, and to assist in our marketing efforts. By continuing to use our site, you consent to the storing of cookies on your device and agree to delivery of content, including web fonts and JavaScript, from third party web services.
Cookie Settings
Last updated: 16 November 2022
StataCorp LLC (StataCorp) strives to provide our users with exceptional products and services. To do so, we must collect personal information from you. This information is necessary to conduct business with our existing and potential customers. We collect and use this information only where we may legally do so. This policy explains what personal information we collect, how we use it, and what rights you have to that information.
These cookies are essential for our website to function and do not store any personally identifiable information. These cookies cannot be disabled.
This website uses cookies to provide you with a better user experience. A cookie is a small piece of data our website stores on a site visitor's hard drive and accesses each time you visit so we can improve your access to our site, better understand how you use our site, and serve you content that may be of interest to you. For instance, we store a cookie when you log in to our shopping cart so that we can maintain your shopping cart should you not complete checkout. These cookies do not directly store your personal information, but they do support the ability to uniquely identify your internet browser and device.
Please note: Clearing your browser cookies at any time will undo preferences saved here. The option selected here will apply only to the device you are currently using.