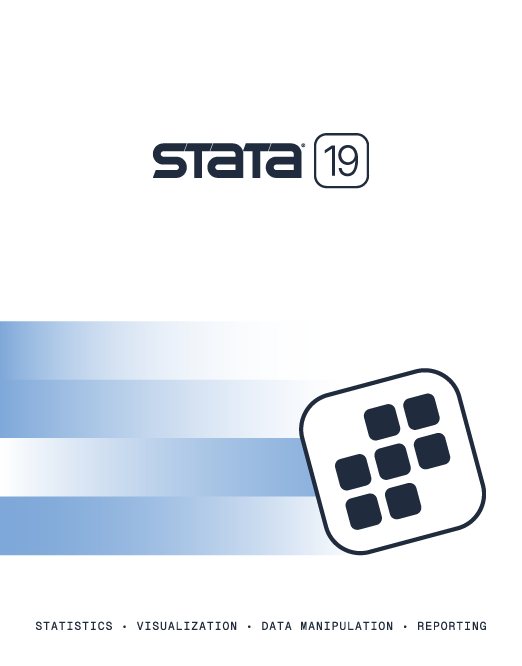
2025 Stata Conference • Nashville, TN • 31 July–01 August
Applied Longitudinal Data Analysis for Epidemiology: A Practical Guide, Second Edition |
||||||||||||||||||||||||||||||||||||
![]() Click to enlarge See the back cover |
![]()
As an Amazon Associate, StataCorp earns a small referral credit from
qualifying purchases made from affiliate links on our site.
eBook not available for this title
eBook not available for this title |
|
||||||||||||||||||||||||||||||||||
Comment from the Stata technical groupApplied Longitudinal Data Analysis for Epidemiology: A Practical Guide, Second Edition, by Jos W. R. Twisk, provides a practical introduction to the estimation techniques used by epidemiologists for longitudinal data. Topics include ANOVA, MANOVA, generalized estimating equation estimators, and mixed models. Continuous, dichotomous, and count outcomes are considered. Rather than providing a rigorous theoretical discussion of each estimator, Twisk builds an intuitive foundation for each method and then provides examples and a discussion of how to interpret the estimates. Stata is used to produce virtually all the example output in the text. Twisk’s book is suitable for use as a supplementary text in an applied statistics course for epidemiologists and is also a succinct reference for practicing epidemiological researchers. |
||||||||||||||||||||||||||||||||||||
Table of contentsView table of contents >> Table of Contents
Preface
Acknowledgements
1 Introduction
1.1 Introduction
1.2 General approach 1.3 Prior knowledge 1.4 Example 1.5 Software 1.6 Data structure 1.7 Statistical notation 1.8 What’s new in the second edition? 2 Study design
2.1 Introduction
2.2 Observational longitudinal studies
2.2.1 Period and cohort effects
2.3 Experimental (longitudinal) studies 2.2.2 Other confounding effects 2.2.3 Example 3 Continuous outcome variables
3.1 Two measurements
3.1.1 Example
3.2 Non-parametric equivalent of the paired t-test
3.2.1 Example
3.3 More than two measurements
3.3.1 The “univariate” approach: a numerical example
3.4 The “univariate” or the “multivariate” approach? 3.3.2 The shape of the relationship between an outcome variable and time 3.3.3 A numerical example 3.3.4 Example 3.5 Comparing groups
3.5.1 The “univariate” approach: a numerical example
3.6 Comments 3.5.2 Example 3.7 Post-hoc procedures
3.7.1 Example
3.8 Different contrasts
3.8.1 Example
3.9 Non-parametric equivalent of MANOVA for repeated measurements
3.9.1 Example
4 Continuous outcome variables — relationships with other variables
4.1 Introduction
4.2 “Traditional” methods 4.3 Example 4.4 Longitudinal methods 4.5 Generalized estimating equations
4.5.1 Introduction
4.6 Mixed model analysis 4.5.2 Working correlation structures 4.5.3 Interpretation of the regression coefficients derived from GEE analysis 4.5.4 Example
4.5.4.1 Introduction
4.5.4.2 Results of a GEE analysis 4.5.4.3 Different correlation structures
4.6.1 Introduction
4.7 Comparison between GEE analysis and mixed model analysis 4.6.2 Mixed models for longitudinal studies 4.6.3 Example 4.6.4 Comments
4.7.1 The “adjustment for covariance” approach
4.7.2 Extensions of mixed model analysis 4.7.3 Comments 5 The modeling of time
5.1 The development over time
5.2 Comparing groups 5.3 The adjustment for time 6 Other possibilities for modeling longitudinal data
6.1 Introduction
6.2 Alternative models
6.2.1 Time-lag model
6.3 Comments 6.2.2 Model of changes 6.2.3 Autoregressive model 6.2.4 Overview 6.2.5 Example
6.2.5.1 Introduction
6.2.5.2 Data structure for alternative models 6.2.5.3 GEE analysis 6.2.5.4 Mixed model analysis 6.4 Another example 7 Dichotomous outcome variables
7.1 Simple methods
7.1.1 Two measurements
7.2 Relationships with other variables 7.1.2 More than two measurements 7.1.3 Comparing groups 7.1.4 Example
7.1.4.1 Introduction
7.1.4.2 Development over time 7.1.4.3 Comparing groups
7.2.1 “Traditional” methods
7.2.2 Example 7.2.3 Sophisticated methods 7.2.4 Example
7.2.4.1 Generalized estimating equations
7.2.5 Comparison between GEE analysis and mixed model analysis 7.2.4.2 Mixed model analysis 7.2.6 Alternative models 7.2.7 Comments 8 Categorical and “count” outcome variables
8.1 Categorical outcome variables
8.1.1 Two measurements
8.2 “Count” outcome variables 8.1.2 More than two measurements 8.1.3 Comparing groups 8.1.4 Example 8.1.5 Relationship with other variables
8.1.5.1 “Traditional” methods
8.1.5.2 Example 8.1.5.3 Sophisticated methods 8.1.5.4 Example
8.2.1 Example
8.3 Comments
8.2.1.1 Introduction
8.2.2 Comparison between GEE analysis and mixed model analysis 8.2.1.2 GEE analysis 8.2.1.3 Mixed model analysis 9 Analysis of experimental studies
9.1 Introduction
9.2 Continuous outcome variables
9.2.1 Experimental models with only one follow-up measurement
9.3 Dichotomous outcome variables
9.2.1.1 Example
9.2.2 Experimental studies with more than one follow-up measurement
9.2.2.1 Simple analysis
9.2.3 Conclusion 9.2.2.2 Summary statistics 9.2.2.3 MANOVA for repeated measurements 9.2.2.4 MANOVA for repeated measurements adjusted for the baseline value 9.2.2.5 Sophisticated analysis
9.3.1 Introduction
9.4 Comments 9.3.2 Simple analysis 9.3.3 Sophisticated analysis 9.3.4 Other approaches 10 Missing data in longitudinal studies
10.1 Introduction
10.2 Ignorable or informative missing data? 10.3 Example
10.3.1 Generating datasets with missing data
10.4 Analysis performed on datasets with missing data 10.3.2 Analysis of determinants for missing data
10.4.1 Example
10.5 Imputation methods
10.5.1 Continuous outcome variables
10.6 GEE analysis versus mixed model analysis regarding the analysis on datasets with missing data
10.5.1.1 Cross-sectional imputation methods
10.5.2 Dichotomous and categorical outcome variables 10.5.1.2 Longitudinal imputation methods 10.5.1.3 Comment 10.5.1.4 Multiple imputation 10.5.3 Example
10.5.3.1 Continuous outcome variables
10.5.4 Comments 10.5.3.2 Should multiple imputation be used in combination with a mixed model analysis? 10.5.3.3 Additional analyses 10.5.3.4 Dichotomous outcome variables
10.5.4.1 Alternative approaches
10.7 Conclusions 11 Sample size calculations
11.1 Introduction
11.2 Example 12 Software for longitudinal data analysis
12.1 Introduction
12.2 GEE analysis with continuous outcome variables
12.2.1 Stata
12.3 GEE analysis with dichotomous outcome variables 12.2.2 SAS 12.2.3 R 12.2.4 SPSS 12.2.5 Overview
12.3.1 Stata
12.4 Mixed model analysis with continuous outcome variables 12.3.2 SAS 12.3.3 R 12.3.4 SPSS 12.3.5 Overview
12.4.1 Stata
12.5 Mixed model analysis with dichotomous outcome variables 12.4.2 SAS 12.4.3 R 12.4.4 SPSS 12.4.5 MLwiN 12.4.6 Overview
12.5.1 Introduction
12.6 Categorical and “count” outcome variables 12.5.2 Stata 12.5.3 SAS 12.5.4 R 12.5.5 SPSS 12.5.6 MLwiN 12.5.7 Overview 12.7 The “adjustment for covariance approach”
12.7.1 Example
13 One step further
13.1 Introduction
13.2 Outcome variables with upper or lower censoring
13.2.1 Introduction
13.3 Classification of subjects with different developmental trajectories 13.2.2 Example 13.2.3 Remarks References
Index
|
Learn
Free webinars
NetCourses
Classroom and web training
Organizational training
Video tutorials
Third-party courses
Web resources
Teaching with Stata
© Copyright 1996–2025 StataCorp LLC. All rights reserved.
×
We use cookies to ensure that we give you the best experience on our website—to enhance site navigation, to analyze usage, and to assist in our marketing efforts. By continuing to use our site, you consent to the storing of cookies on your device and agree to delivery of content, including web fonts and JavaScript, from third party web services.
Cookie Settings
Last updated: 16 November 2022
StataCorp LLC (StataCorp) strives to provide our users with exceptional products and services. To do so, we must collect personal information from you. This information is necessary to conduct business with our existing and potential customers. We collect and use this information only where we may legally do so. This policy explains what personal information we collect, how we use it, and what rights you have to that information.
These cookies are essential for our website to function and do not store any personally identifiable information. These cookies cannot be disabled.
This website uses cookies to provide you with a better user experience. A cookie is a small piece of data our website stores on a site visitor's hard drive and accesses each time you visit so we can improve your access to our site, better understand how you use our site, and serve you content that may be of interest to you. For instance, we store a cookie when you log in to our shopping cart so that we can maintain your shopping cart should you not complete checkout. These cookies do not directly store your personal information, but they do support the ability to uniquely identify your internet browser and device.
Please note: Clearing your browser cookies at any time will undo preferences saved here. The option selected here will apply only to the device you are currently using.