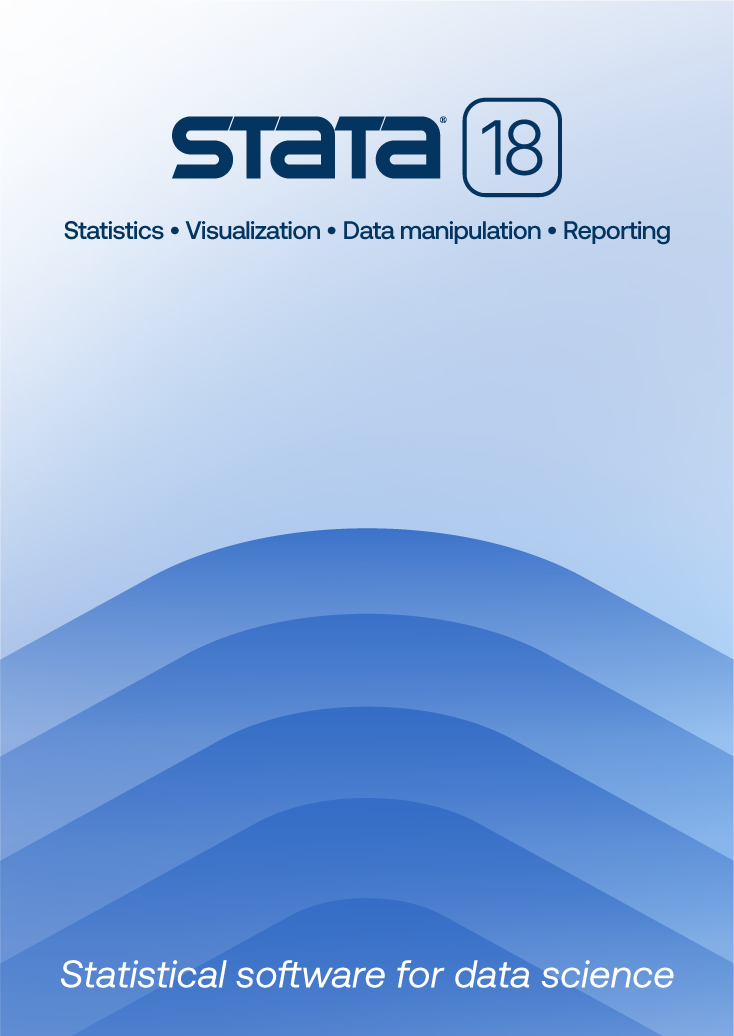
Econometric Analysis of Cross Section and Panel Data, Second Edition |
||||||||||||||||||||||||||||||||||||
![]() Click to enlarge See the back cover |
![]()
As an Amazon Associate, StataCorp earns a small referral credit from
qualifying purchases made from affiliate links on our site.
eBook not available for this title
eBook not available for this title |
|
||||||||||||||||||||||||||||||||||
Comment from the Stata technical groupThe second edition of Econometric Analysis of Cross Section and Panel Data, by Jeffrey Wooldridge, is invaluable to students and practitioners alike, and it should be on the shelf of all students and practitioners who are interested in microeconometrics. This book is more focused than some other books on microeconometrics. It delves more deeply into the intuition and the theory underlying the covered techniques. The theoretical discussions can be understood by students, practitioners, and theoreticians. This book does not provide detailed coverage of simulation-based estimation techniques, resampling methods for estimating the distributions of estimators and test statistics, or nonparametric methods. The author’s focused approach leads to outstanding treatments of the covered topics. Wooldridge’s book provides an impressive introduction to state-of-the-art methods for solving real-world problems in econometrics, including instructive examples and applied problems. In particular, the author approaches problems by applying the analogy principle and a general estimation method, by relying on the assumption that right-hand side variables are always random covariates, by paying close attention to the sampling design, and by treating interpretation as vital to the process. This textbook provides outstanding coverage of sampling design, the use of survey weights for estimation and inference, the generalized method of moments (GMM) approach to panel data, and the related issues of sample selection, stratified sampling, and attrition in panel data. The author’s list of additions to the second edition is four pages long. Among the important additions are a more complete discussion of the Mundlak–Chamberlain approach to linear and nonlinear panel-data estimators, more thorough discussions of the control function approach to models with endogenous variables, estimators for many more nonlinear models, and a thorough rewrite of the chapter on estimating average treatment effects to reflect the latest research. |
||||||||||||||||||||||||||||||||||||
Table of contentsView table of contents >> Preface
Acknowledgments
I INTRODUCTION AND BACKGROUND
1 Introduction
1.1 Causal Relationships and Ceteris Paribus Analysis
1.2 Stochastic Setting and Asymptotic Analysis
1.2.1 Data Structures
1.3 Some Examples 1.2.2 Asymptotic Analysis 1.4 Why Not Fixed Explanatory Variables? 2 Conditional Expectations and Related Concepts in Econometrics
2.1 Role of Conditional Expectations in Econometrics
2.2 Features of Conditional Expectations
2.2.1 Definition and Examples
2.3 Linear Projections 2.2.2 Partial Effects, Elasticities, and Semielasticities 2.2.3 Error Form of Models of Conditional Expectations 2.2.4 Some Properties of Conditional Expectations 2.2.5 Average Partial Effects
Problems
Appendix 2A 2.A.1 Properties of Conditional Expectations 2.A.2 Properties of Conditional Variances and Covariances 2.A.3 Properties of Linear Projections 3 Basic Asymptotic Theory
3.1 Convergence of Deterministic Sequences
3.2 Convergence in Probability and Boundedness in Probability 3.3 Convergence in Distribution 3.4 Limit Theorems for Random Samples 3.5 Limiting Behavior of Estimators and Test Statistics
3.5.1 Asymptotic Properties of Estimators
3.5.2 Asymptotic Properties of Test Statistics Problems II LINEAR MODELS
4 Single-Equation Linear Model and Ordinary Least Squares Estimation
4.1 Overview of the Single-Equation Linear Model
4.2 Asymptotic Properties of Ordinary Least Squares
4.2.1 Consistency
4.3 Ordinary Least Squares Solutions to the Omitted Variables Problem
4.2.2 Asymptotic Inference Using Ordinary Least Squares 4.2.3 Heteroskedasticity-Robust Inference 4.2.4 Lagrange Multiplier (Score) Tests
4.3.1 Ordinary Least Squares Ignoring the Omitted Variables
4.4 Properties of Ordinary Least Squares under Measurement Error
4.3.2 Proxy Variable–Ordinary Least Squares Solution 4.3.3 Models with Interactions in Unobservables: Random Coefficient Models
4.4.1 Measurement Error in the Dependent Variable
4.4.2 Measurement Error in an Explanatory Variable Problems 5 Instrumental Variables Estimation of Single-Equation Linear Models
5.1 Instrumental Variables and Two-Stage Least Squares
5.1.1 Motivation for Instrumental Variables Estimation
5.2 General Treatment of Two-Stage Least Squares
5.1.2 Multiple Instruments: Two-Stage Least Squares
5.2.1 Consistency
5.3 IV Solutions to the Omitted Variables and Measurement Error Problems
5.2.2 Asymptotic Normality of Two-Stage Least Squares 5.2.3 Asymptotic Efficiency of Two-Stage Least Squares 5.2.4 Hypothesis Testing with Two-Stage Least Squares 5.2.5 Heteroskedasticity-Robust Inference for Two-Stage Least Squares 5.2.6 Potential Pitfalls with Two-Stage Least Squares
5.3.1 Leaving the Omitted Factors in the Error Term
5.3.2 Solutions Using Indicators of the Unobservables Problems 6 Additional Single-Equation Topics
6.1 Estimation with Generated Regressors and Instruments
6.1.1 Ordinary Least Squares with Generated Regressors
6.2 Control Function Approach to Endogeneity6.1.2 Two-Stage Least Squares with Generated Instruments 6.1.3 Generated Instruments and Regressors 6.3 Some Specification Tests
6.3.1 Testing for Endogeneity
6.4 Correlated Random Coefficient Models6.3.2 Testing Overidentifying Restrictions 6.3.3 Testing Functional Form 6.3.4 Testing for Heteroskedasticity
6.4.1 When Is the Usual IV Estimator Consistent?
6.5 Pooled Cross Sections and Difference-in-Differences Estimation6.4.2 Control Function Approach
6.5.1 Pooled Cross Sections over Time
6.5.2 Policy Analysis and Difference-in-Differences Estimation Problems Appendix 6A 7 Estimating Systems of Equations by Ordinary Least
Squares and Generalized Least Squares
7.1 Introduction
7.2 Some Examples 7.3 System Ordinary Least Squares Estimation of a Multivariate Linear System
7.3.1 Preliminaries
7.4 Consistency and Asymptotic Normality of Generalized Least Squares
7.3.2 Asymptotic Properties of System Ordinary Least Squares 7.3.3 Testing Multiple Hypotheses
7.4.1 Consistency
7.5 Feasible Generalized Least Squares
7.4.2 Asymptotic Normality
7.5.1 Asymptotic Properties
7.6 Testing the Use of Feasible Generalized Least Squares 7.5.2 Asymptotic Variance of Feasible Generalized Least Squares under a Standard Assumption 7.5.3 Properties of Feasible Generalized Least Squares with (Possibly Incorrect) Restrictions on the Unconditional Variance Matrix 7.7 Seemingly Unrelated Regressions, Revisited
7.7.1 Comparison between Ordinary Least Squares and Feasible Generalized Least Squares for Seemingly Unrelated Regressions Systems
7.8 The Linear Panel Data Model, Revisited
7.7.2 Systems with Cross Equation Restrictions 7.7.3 Singular Variance Matrices in Seemingly Unrelated Regressions Systems
7.8.1 Assumptions for Pooled Ordinary Least Squares
7.8.2 Dynamic Completeness 7.8.3 Note on Time Series Persistence 7.8.4 Robust Asymptotic Variance Matrix 7.8.5 Testing for Serial Correlation and Heteroskedasticity after Pooled Ordinary Least Squares 7.8.6 Feasible Generalized Least Squares Estimation under Strict Exogeneity Problems 8 System Estimation by Instrumental Variables
8.1 Introduction and Examples
8.2 General Linear System of Equations 8.3 Generalized Method of Moments Estimation
8.3.1 General Weighting Matrix
8.4 Generalized Instrumental Variables Estimator8.3.2 System Two-Stage Least Squares Estimator 8.3.3 Optimal Weighting Matrix 8.3.4 The Generalized Method of Moments Three-Stage Least Squares Estimator
8.4.1 Derivation of the Generalized Instrumental Variables Estimator and Its Asymptotic Properties
8.5 Testing Using Generalized Method of Moments
8.4.2 Comparison of Generalized Method of Moment, Generalized Instrumental Variables, and the Traditional Three-Stage Least Squares Estimator
8.5.1 Testing Classical Hypotheses
8.6 More Efficient Estimation and Optimal Instruments 8.5.2 Testing Overidentification Restrictions 8.7 Summary Comments on Choosing an Estimator
Problems
9 Simultaneous Equations Models
9.1 Scope of Simultaneous Equations Models
9.2 Identification in a Linear System
9.2.1 Exclusion Restrictions and Reduced Forms
9.3 Estimation after Identification
9.2.2 General Linear Restrictions and Structural Equations 9.2.3 Unidentified, Just Identified, and Overidentified Equations
9.3.1 Robustness-Efficiency Trade-off
9.4 Additional Topics in Linear Simultaneous Equations Methods
9.3.2 When Are 2SLS and 3SLS Equivalent? 9.3.3 Estimating the Reduced Form Parameters
9.4.1 Using Cross Equation Restrictions to Achieve Identification
9.5 Simultaneous Equations Models Nonlinear in Endogenous Variables
9.4.2 Using Covariance Restrictions to Achieve Identification 9.4.3 Subtleties Concerning Identification and Efficiency in Linear Systems
9.5.1 Identification
9.6 Different Instruments for Different Equations 9.5.2 Estimation 9.5.3 Control Function Estimation for Triangular Systems
Problems
10 Basic Linear Unobserved Effects Panel Data Models
10.1 Motivation: Omitted Variables Problem
10.2 Assumptions about the Unobserved Effects and Explanatory Variables
10.2.1 Random or Fixed Effects?
10.3 Estimating Unobserved Effects Models by Pooled Ordinary Least Squares 10.2.2 Strict Exogeneity Assumptions on the Explanatory Variables 10.2.3 Some Examples of Unobserved Effects Panel Data Models 10.4 Random Effects Methods
10.4.1 Estimation and Inference under the Basic Random Effects Assumptions
10.5 Fixed Effects Methods
10.4.2 Robust Variance Matrix Estimator 10.4.3 General Feasible Generalized Least Squares Analysis 10.4.4 Testing for the Presence of an Unobserved Effect
10.5.1 Consistency of the Fixed Effects Estimator
10.6 First Differencing Methods
10.5.2 Asymptotic Inference with Fixed Effects 10.5.3 Dummy Variable Regression 10.5.4 Serial Correlation and the Robust Variance Matrix Estimator 10.5.5 Fixed Effects Generalized Least Squares 10.5.6 Using Fixed Effects Estimation for Policy Analysis
10.6.1 Inference
10.7 Comparison of Estimators
10.6.2 Robust Variance Matrix 10.6.3 Testing for Serial Correlation 10.6.4 Policy Analysis Using First Differencing
10.7.1 Fixed Effects versus First Differencing
10.7.2 The Relationship between the Random Effects and Fixed Effect Estimators 10.7.3 The Hausman Test Comparing Random Effects and Fixed Effects Estimators Problems 11 More Topics in Linear Unobserved Effects Models
11.1 Generalized Method of Moments Approaches to the Standard Linear Unobserved Effects Model
11.1.1 Equivalence between GMM 3SLS and Standard Estimators
11.2 Random and Fixed Effects Instrumental Variables Methods11.1.2 Chamberlain’s Approach to Unobserved Effects Models 11.3 Hausman and Taylor–Type Models 11.4 First Differencing Instrumental Variables Methods 11.5 Unobserved Effects Models with Measurement Error 11.6 Estimation under Sequential Exogeneity
11.6.1 General Framework
11.7 Models with Individual-Specific Slopes11.6.2 Models with Lagged Dependent Variables
11.7.1 Random Trend Model
11.7.2 General Models with Individual-Specific Slopes 11.7.3 Robustness of Standard Fixed Effects Methods 11.7.4 Testing for Correlated Random Slopes Problems III GENERAL APPROACHES TO NONLINEAR ESTIMATION
12 M-Estimation, Nonlinear Regression, and Quantile Regression
12.1 Introduction
12.2 Identification, Uniform Convergence, and Consistency 12.3 Asymptotic Normality 12.4 Two-Step M-Estimators
12.4.1 Consistency
12.5 Estimating the Asymptotic Variance
12.4.2 Asymptotic Normality
12.5.1 Estimation without Nuisance Parameters
12.6 Hypothesis Testing
12.5.2 Adjustments for Two-Step Estimation
12.6.1 Wald Tests
12.7 Optimization Methods
12.6.2 Score (or Lagrange Multiplier) Tests 12.6.3 Tests Based on the Change in the Objective Function 12.6.4 Behavior of the Statistics under Alternatives
12.7.1 Newton-Raphson Method
12.8 Simulation and Resampling Methods
12.7.2 Berndt, Hall, Hall, and Hausman Algorithm 12.7.3 Generalized Gauss-Newton Method 12.7.4 Concentrating Parameters out of the Objective Function
12.8.1 Monte Carlo Simulation
12.9 Multivariate Nonlinear Regression Methods12.8.2 Bootstrapping
12.9.1 Multivariate Nonlinear Least Squares
12.10 Quantile Estimation12.9.2 Weighted Multivariate Nonlinear Least Squares
12.10.1 Quantiles, the Estimation Problem, and Consistency
12.10.2 Asymptotic Inference 12.10.3 Quantile Regression for Panel Data Problems 13 Maximum Likelihood Methods
13.1 Introduction
13.2 Preliminaries and Examples 13.3 General Framework for Conditional Maximum Likelihood Estimation 13.4 Consistency of Conditional Maximum Likelihood Estimation 13.5 Asymptotic Normality and Asymptotic Variance Estimation
13.5.1 Asymptotic Normality
13.6 Hypothesis Testing 13.5.2 Estimating the Asymptotic Variance 13.7 Specification Testing 13.8 Partial (or Pooled) Likelihood Methods for Panel Data
13.8.1 Setup for Panel Data
13.9 Panel Data Models with Unobserved Effects
13.8.2 Asymptotic Inference 13.8.3 Inference with Dynamically Complete Models
13.9.1 Models with Strictly Exogenous Explanatory Variables
13.10 Two-Step Estimators Involving Maximum Likelihood 13.9.2 Models with Lagged Dependent Variables
13.10.1 Second-Step Estimator Is Maximum Likelihood Estimator
13.11 Quasi-Maximum Likelihood Estimation13.10.2 Surprising Efficiency Result When the First-Step Estimator Is Conditional Maximum Likelihood Estimator
13.11.1 General Misspecification
13.11.2 Model Selection Tests 13.11.3 Quasi-Maximum Likelihood Estimation in the Linear Exponential Family 13.11.4 Generalized Estimating Equations for Panel Data Problems Appendix 13A 14 Generalized Method of Moments and Minimum Distance Estimation
14.1 Asymptotic Properties of Generalized Method of Moments
14.2 Estimation under Orthogonality Conditions 14.3 Systems of Nonlinear Equations 14.4 Efficient Estimation
14.4.1 General Efficiency Framework
14.5 Classical Minimum Distance Estimation 14.4.2 Efficiency of Maximum Likelihood Estimator 14.4.3 Efficient Choice of Instruments under Conditional Moment Restrictions 14.6 Panel Data Applications
14.6.1 Nonlinear Dynamic Models
14.6.2 Minimum Distance Approach to the Unobserved Effects Model 14.6.3 Models with Time-Varying Coefficients on the Unobserved Effects Problems Appendix 14A IV NONLINEAR MODELS AND RELATED TOPICS
15 Binary Response Models
15.1 Introduction
15.2 The Linear Probability Model for Binary Response 15.3 Index Models for Binary Response: Probit and Logit 15.4 Maximum Likelihood Estimation of Binary Response Index Models 15.5 Testing in Binary Response Index Models
15.5.1 Testing Multiple Exclusion Restrictions
15.6 Reporting the Results for Probit and Logit 15.5.2 Testing Nonlinear Hypotheses about β 15.5.3 Tests against More General Alternatives 15.7 Specification Issues in Binary Response Models
15.7.1 Neglected Heterogeneity
15.8 Binary Response Models for Panel Data
15.7.2 Continuous Endogenous Explanatory Variables 15.7.3 Binary Endogenous Explanatory Variable 15.7.4 Heteroskedasticity and Nonnormality in the Latent Variable Model 15.7.5 Estimation under Weaker Assumptions
15.8.1 Pooled Probit and Logit
15.8.2 Unobserved Effects Probit Models under Strict Exogeneity 15.8.3 Unobserved Effects Logit Models under Strict Exogeneity 15.8.4 Dynamic Unobserved Effects Models 15.8.5 Probit Models with Heterogeneity and Endogenous Explanatory Variables 15.8.6 Semiparametric Approaches Problems 16 Multinomial and Ordered Response Model
16.1 Introduction
16.2 Multinomial Response Models
16.2.1 Multinomial Logit
16.3 Ordered Response Models16.2.2 Probabilistic Choice Models 16.2.3 Endogenous Explanatory Variables 16.2.4 Panel Data Methods
16.3.1 Ordered Logit and Ordered Probit
16.3.2 Specification Issues in Ordered Models 16.3.3 Endogenous Explanatory Variables 16.3.4 Panel Data Methods Problems 17 Corner Solution Responses
17.1 Motivation and Examples
17.2 Useful Expressions for Type I Tobit 17.3 Estimation and Inference with the Type I Tobit Model 17.4 Reporting the Results 17.5 Specification Issues in Tobit Models
17.5.1 Neglected Heterogeneity
17.6 Two-Part Models and Type II Tobit Model
17.5.2 Endogenous Explanatory Models 17.5.3 Heteroskedasticity and Nonnormality in the Latent Variable Model 17.5.4 Estimating Parameters with Weaker Assumptions
17.6.1 Truncated Normal Hurdle Model
17.7 Two-Limit Tobit Model17.6.2 Lognormal Hurdle Model and Exponential Conditional Mean 17.6.3 Exponential Type II Tobit Model 17.8 Panel Data Methods
17.8.1 Pooled Methods
17.8.2 Unobserved Effects Models under Strict Exogeneity 17.8.3 Dynamic Unobserved Effects Tobit Models Problems 18. Count, Fractional, and Other Nonnegative Responses
18.1 Introduction
18.2 Poisson Regression
18.2.1 Assumptions Used for Poisson Regression and Quantities of Interest
18.3 Other Count Data Regression Models18.2.2 Consistency of the Poisson QMLE 18.2.3 Asymptotic Normality of the Poisson QMLE 18.2.4 Hypothesis Testing 18.2.5 Specification Testing
18.3.1 Negative Binomial Regression Models
18.4 Gamma (Exponential) Regression Model18.3.2 Binomial Regression Models 18.5 Endogeneity with an Exponential Regression Function 18.6 Fractional Responses
18.6.1 Exogenous Explanatory Variables
18.7 Panel Data Models18.6.2 Endogenous Explanatory Variables
18.7.1 Pooled QMLE
18.7.2 Specifying Models of Conditional Expectations with Unobserved Effects 18.7.3 Random Effects Methods 18.7.4 Fixed Effects Poisson Estimation 18.7.5 Relaxing the Strict Exogeneity Assumption 18.7.6 Fractional Response Models for Panel Data Problems 19. Censored Data, Sample Selection, and Attrition
19.1 Introduction
19.2 Data Censoring
19.2.1 Binary Censoring
19.3 Overview of Sample Selection19.2.2 Interval Coding 19.2.3 Censoring from Above and Below 19.4 When Can Sample Selection Be Ignored?
19.4.1 Linear Models: Estimation by OLS and 2SLS
19.5 Selection on the Basis of the Response Variable: Truncated Regression19.4.2 Nonlinear Models 19.6 Incidental Truncation: A Probit Selection Equation
19.6.1 Exogenous Explanatory Variables
19.7 Incidental Truncation: A Tobit Selection Equation19.6.2 Endogenous Explanatory Variables 19.6.3 Binary Response Model with Sample Selection 19.6.4 An Exponential Response Function
19.7.1 Exogenous Explanatory Variables
19.8 Inverse Probability Weighting for Missing Data19.7.2 Endogenous Explanatory Variables 19.7.3 Estimating Structural Tobit Equations with Sample Selection 19.9 Sample Selection and Attrition in Linear Panel Data Models
19.9.1 Fixed and Random Effects Estimation with Unbalanced Panels
19.9.2 Testing and Correcting for Sample Selection Bias 19.9.3 Attrition Problems 20 Stratified Sampling and Cluster Sampling
20.1 Introduction
20.2 Stratified Sampling
20.2.1 Standard Stratified Sampling and Variable Probability Sampling
20.3 Cluster Sampling20.2.2 Weighted Estimators to Account for Stratification 20.2.3 Stratification Based on Exogenous Variables
20.3.1 Inference with a Large Number of Clusters and Small Cluster Sizes
20.4 Complex Survey Sampling20.3.2 Cluster Samples with Unit-Specific Panel Data 20.3.3 Should We Apply Cluster-Robust Inference with Large Group Sizes? 20.3.4 Inference When the Number of Clusters is Small
Problems
21 Estimating Average Treatment Effects
21.1 Introduction
21.2 A Counterfactual Setting and the Self-Selection Problem 21.3 Methods Assuming Ignorability (or Unconfoundedness) of Treatment
21.3.1 Identification
21.4 Instrumental Variables Methods
21.3.2 Regression Adjustment 21.3.3 Propensity Score Analysis 21.3.4 Combining Regression Adjustment and Propensity Score Weighting 21.3.5 Matching Methods
21.4.1 Estimating the Average Treatment Effect Using IV
21.5 Regression Discontinuity Designs21.4.2 Correction and Control Function Approaches 21.4.3 Estimating the Local Average Treatment Effect by IV
21.5.1 The Sharp Regression Discontinuity Design
21.6 Further Issues
21.5.2 The Fuzzy Regression Discontinuity Design 21.5.3 Unconfoundedness versus the Fuzzy Regression Discontinuity
21.6.1 Special Considerations for Responses with Discreteness or Limited Range
21.6.2 Multivalued Treatments 21.6.3 Multiple Treatments 21.6.4 Panel Data Problems 22 Duration Analysis
22.1 Introduction
22.2 Hazard Functions
22.2.1 Hazard Functions without Covariates
22.3 Analysis of Single-Spell Data with Time-Invariant Covariates22.2.2 Hazard Functions Conditional on Time-Invariant Covariates 22.2.3 Hazard Functions Conditional on Time-Varying Covariates
22.3.1 Flow Sampling
22.4 Analysis of Grouped Duration Data22.3.2 Maximum Likelihood Estimation with Censored Flow Data 22.3.3 Stock Sampling 22.3.4 Unobserved Heterogeneity
22.4.1 Time-Invariant Covariates
22.5 Further Issues22.4.2 Time-Varying Covariates 22.4.3 Unobserved Heterogeneity
22.5.1 Cox’s Partial Likelihood Method for the Proportional Hazard Model
22.5.2 Multiple-Spell Data 22.5.3 Competing Risks Models Problems References
Index
|
Learn
NetCourses
Classroom and web training
Organizational training
Webinars
Video tutorials
Third-party courses
Web resources
Teaching with Stata
© Copyright 1996–2024 StataCorp LLC. All rights reserved.
×
We use cookies to ensure that we give you the best experience on our website—to enhance site navigation, to analyze usage, and to assist in our marketing efforts. By continuing to use our site, you consent to the storing of cookies on your device and agree to delivery of content, including web fonts and JavaScript, from third party web services.
Cookie Settings
Last updated: 16 November 2022
StataCorp LLC (StataCorp) strives to provide our users with exceptional products and services. To do so, we must collect personal information from you. This information is necessary to conduct business with our existing and potential customers. We collect and use this information only where we may legally do so. This policy explains what personal information we collect, how we use it, and what rights you have to that information.
These cookies are essential for our website to function and do not store any personally identifiable information. These cookies cannot be disabled.
This website uses cookies to provide you with a better user experience. A cookie is a small piece of data our website stores on a site visitor's hard drive and accesses each time you visit so we can improve your access to our site, better understand how you use our site, and serve you content that may be of interest to you. For instance, we store a cookie when you log in to our shopping cart so that we can maintain your shopping cart should you not complete checkout. These cookies do not directly store your personal information, but they do support the ability to uniquely identify your internet browser and device.
Please note: Clearing your browser cookies at any time will undo preferences saved here. The option selected here will apply only to the device you are currently using.