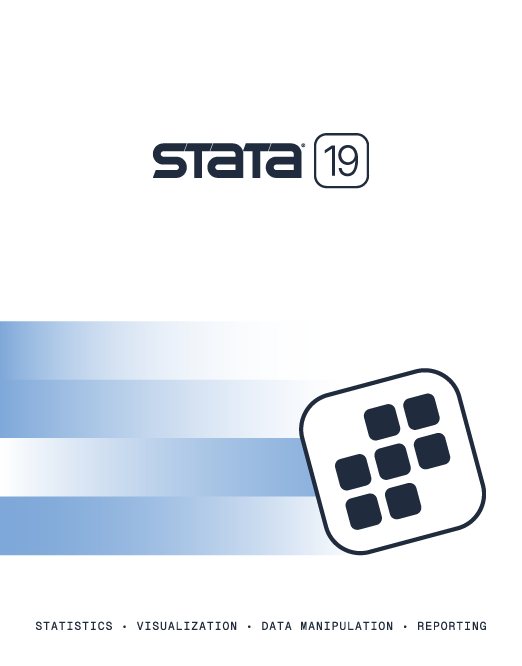
2025 Stata Conference • Nashville, TN • 31 July–01 August
Analysis of Panel Data, Fourth Edition |
||||||||||||||||||||||||||||||||||||
![]() Click to enlarge See the back cover |
![]()
As an Amazon Associate, StataCorp earns a small referral credit from
qualifying purchases made from affiliate links on our site.
eBook not available for this title
eBook not available for this title |
|
||||||||||||||||||||||||||||||||||
Comment from the Stata technical groupCheng Hsiao's Analysis of Panel Data, Fourth Edition is an essential reference on panel-data models. The fourth edition is a minor but important revision; like the previous three editions, it is a must-have reference book for researchers and graduate students. The fourth edition retains most of the content from the previous edition, although some chapters were reordered. The first two chapters of the book provide detailed introductions to static random- and fixed-effects models, including model estimation, specification testing, and treatment of heteroskedasticity and correlation. Chapter 3 is a concise overview of dynamic panel-data models, including the GMM estimators that have become quite popular in recent years, and Hsiao does a superb job of bringing the literature together into one cohesive discussion. Estimation of static simultaneous-equation models is covered in chapter 4. Chapter 5 covers dynamic panel-data systems, including vector autoregressive models, conintegrated models, and time-series-related tests. Chapters 6--8 discuss parametric and semiparametric approaches to limited dependent-variable models and provide many references to the growing literature in this field. Particularly, chapter 6 includes a clear presentation of the incidental parameters problem when it comes to nonlinear panel estimation with individual effects. Hsiao does an excellent job of covering these essential topics. Chapter 9 presents a smorgasbord of further topics, including simulation, multilevel models, pseudopanels, and missing data. The section is rather brief, but it does provide many references to the relevant literature. Chapter 10 adds new topics, such as mixed effects and factor dimension determination. Chapter 11 covers cross-sectionally dependent data. Chapter 12 provides tools for evaluating treatment for both cross-sectional and panel data. Chapter 13 is devoted to variable-coefficient models, including Swamy's random-coefficients model and its relatives: fixed-coefficients models and their dynamic counterparts. Chapter 14 is new to this edition and makes the latest edition more suitable for recent challenges regarding analyzing big and high-dimensional data and using machine learning algorithms. In short, the fourth edition of Analysis of Panel Data, just like the first three editions, will prove to be an invaluable reference to users of panel data. |
||||||||||||||||||||||||||||||||||||
Table of contentsView table of contents >> List of Figures
List of Tables
Preface to the Fourth Edition
1. Introduction
1.1 Introduction
1.2 Advantages of Panel Data 1.3 Challenges to Panel Data Analysis 1.4 Outline of the Monograph 2. Linear Static Models with Additive Effects
2.1 Introduction
2.2 Fixed-Effects Models: Dummy-Variable Approach 2.3 Random-Effects Models: Variance-Components Models 2.4 Fixed Effects or Random Effects 2.5 Tests for Misspecification 2.6 Models with Time-and/or Individual-Invariant Explanatory Variables and both Individual-and Time-Specific Effects 2.7 Analysis of Covariance Tests for the Presence of Individual-or Time-Specific Effects 2.8 Heteroscedasticity and Autocorrelation 2.9 Models with Arbitrary Error Structure: Chamberlain ℼ -Approach
Appendix 2A: Consistency and Asymptotic Normality of the Minimum-Distance Estimator
Appendix 2B: Characteristic Vectors and the Inverse of the Variance–Covariance Matrix of a Three-Component Model 3. Dynamic Models with Additive Specific Effects
3.1 Introduction
3.2 The Least Squares and the Least Squares Dummy Variable (Covariance) Estimator 3.3 Method of Moments Estimator 3.4 The Quasi-Likelihood Approach for Random-Effects Models 3.5 The Likelihood Approach-Fixed-Effects Models 3.6 Relations between the Likelihood Based Estimator and the GMM 3.7 Estimation of Dynamic Models with Arbitrary Serial Correlations in the Residuals 3.8 Models with Both Individual- and Time-Specific Additive Effects Appendix 3A: Derivation of the Asymptotic Covariance Matrix of the Feasible MDE Appendix 3B: Large N and T Asymptotics 4. Simultaneous-Equations Models
4.1 Introduction
4.2 Joint Generalized Least Squares Estimation Technique 4.3 Estimation of the Structural Equations 4.4 Triangular System Appendix 4A: The Determinant and Inverse of the Triangular System Covariance Matrix 5. Dynamic System
5.1 Introduction
5.2 Panel Vector Autoregressive Models 5.3 Cointegrated Panel Models and Vector Error Correction 5.4 Unit Root and Cointegration Tests 5.5 Dynamic Simultaneous Equation Models 6. Discrete Data
6.1 Introduction
6.2 Qualitative Response Models for Cross-Sectional Data 6.3 Panel Parametric Approach to Static Models with Heterogeneity 6.4 Semiparametric Approach to Static Models 6.5 Dynamic Models 6.6 Alternative Approaches for Identifying Dynamic Dependence 7. Limited Dependent and Sample Selection Models
7.1 Cross-Sectional Data Approach
7.2 A Sample Selection Example: Nonrandomly Missing Data in a Panel 7.3 Tobit Models with Random Individual Effects 7.4 Fixed-Effects Estimator 7.5 An Example: Housing Expenditure 7.6 Dynamic Tobit Models 8. Some Nonlinear Models
8.1 Duration Model
8.2 Count Data Model 8.3 Nonparametric Models 9. Miscellaneous Topics
9.1 Quantile Regression Analysis
9.2 Simulation Methods 9.3 Data with Multilevel Structures 9.4 Errors of Measurement 9.5 Estimating Distributed Lags in Short Panels 9.6 Rotating or Randomly Missing Data 9.7 Pseudo Panels (or Repeated Cross-Sectional Data) 9.8 Discretizing Unobserved Heterogeneity 10. Interactive Effects Models
10.1 Introduction
10.2 Fixed-Effects Linear Static Models 10.3 Fixed-Effects Linear Dynamic Models 10.4 Models with Mixed Fixed and Random Effects 10.5 Quantile Models 10.6 Factor Dimension Determination 11. Spatial Models and Tests for Cross-Sectional Dependence
11.1 Introduction– Weak or Strong Cross–Correlation
11.2 The Basic Formulation on Spatial Weight Matrix and Spatial (Dependence) Coefficients 11.3 Spatial Error Models 11.4 Spatial Regressive Models 11.5 Some Extensions 11.6 Mixed Spatial and Factor Process 11.7 Cross-Sectional Dependence Test 12. Program Evaluation Using Panel Data
12.1 Introduction
12.2 Cross-Sectional Data Approach 12.3 Panel Data Approach 12.4 Some Examples 12.5 Multiple Treated Units 12.6 Simulating or Ranking Program Outcomes with Different Policy Options 13. Variable Coefficients Models
13.1 Introduction
13.2 Coefficients that Vary over Cross-Sectional Units 13.3 Coefficients that Vary over Time and Cross-Sectional Units 13.4 A Mixed Fixed- and Random-Coefficients Model 13.5 Dynamic Random-Coefficients Models 13.6 An Example: Liquidity Constraints and Firm Investment Expenditure 13.7 Coefficients that Vary over Time 13.8 Coefficients that Are Functions of Other Exogenous Variables 13.9 Correlated Random-Coefficients Models Appendix 13A: Combination of Two Normal Distributions 14. Big Data Analysis
14.1 Machine Learning Algorithms
14.2 Inference with High-Dimensional Data 14.3 Inference for Low-Dimensional Parameters in the Presence of High-Dimensional Data 14.4 Prediction References
Author Index
Subject Index
|
Learn
Free webinars
NetCourses
Classroom and web training
Organizational training
Video tutorials
Third-party courses
Web resources
Teaching with Stata
© Copyright 1996–2025 StataCorp LLC. All rights reserved.
×
We use cookies to ensure that we give you the best experience on our website—to enhance site navigation, to analyze usage, and to assist in our marketing efforts. By continuing to use our site, you consent to the storing of cookies on your device and agree to delivery of content, including web fonts and JavaScript, from third party web services.
Cookie Settings
Last updated: 16 November 2022
StataCorp LLC (StataCorp) strives to provide our users with exceptional products and services. To do so, we must collect personal information from you. This information is necessary to conduct business with our existing and potential customers. We collect and use this information only where we may legally do so. This policy explains what personal information we collect, how we use it, and what rights you have to that information.
These cookies are essential for our website to function and do not store any personally identifiable information. These cookies cannot be disabled.
This website uses cookies to provide you with a better user experience. A cookie is a small piece of data our website stores on a site visitor's hard drive and accesses each time you visit so we can improve your access to our site, better understand how you use our site, and serve you content that may be of interest to you. For instance, we store a cookie when you log in to our shopping cart so that we can maintain your shopping cart should you not complete checkout. These cookies do not directly store your personal information, but they do support the ability to uniquely identify your internet browser and device.
Please note: Clearing your browser cookies at any time will undo preferences saved here. The option selected here will apply only to the device you are currently using.