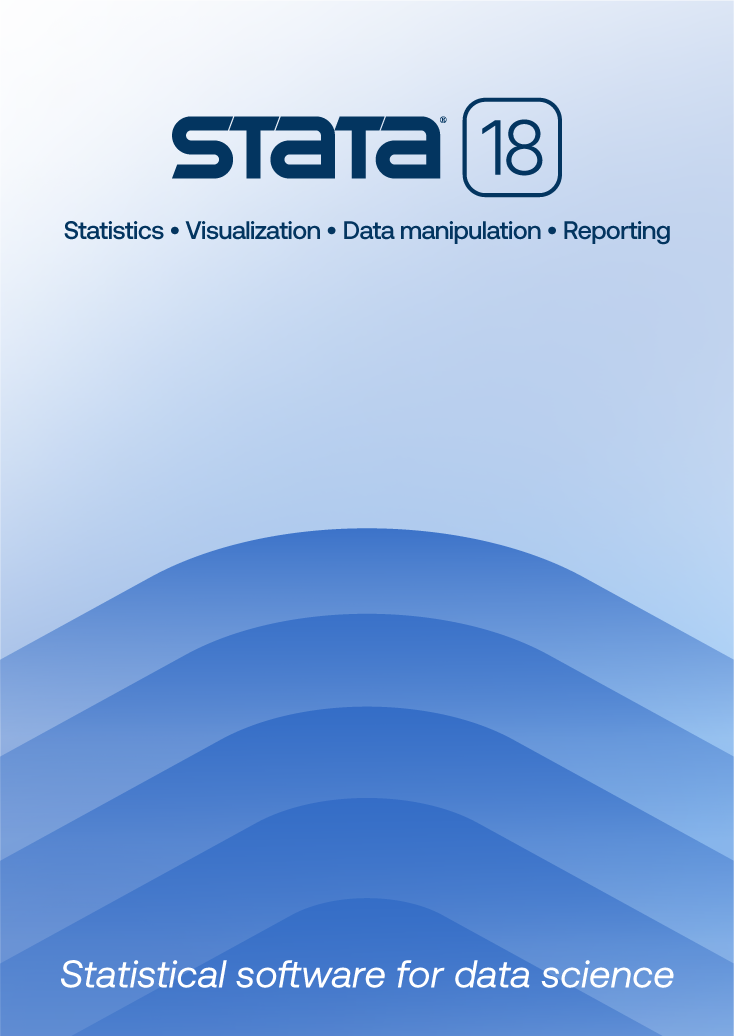
Title | Using Stata to solve a system of nonlinear equations | |
Authors |
Alan H. Feiveson, NASA Kerry Kammire, StataCorp Isabel Canette, StataCorp |
We will show you two ways of solving a system of nonlinear equations in Stata. First, we will provide a detailed explanation using nl. Then we will show you the equivalent in Mata.
Suppose you want to solve
f1(a1,...,an) = 0 f2(a1,...,an) = 0 . . . fn(a1,...,an) = 0
(n nonlinear equations in n unknowns a1,..,an).
First, rewrite the first equation so that its right-hand side is 1:
f1(a1,...,an) + 1 = 1 f2(a1,...,an) = 0 . . . fn(a1,...,an) = 0
Then set up a fake dataset with n observations as follows:
program nlfaq syntax varlist (min=1 max=1) [if], at(name) tempname a1 a2 a3 scalar `a1' = `at'[1, 1] scalar `a2' = `at'[1, 2] scalar `a3' = `at'[1, 3] tempvar yh generate double `yh' = f1(`a1', `a2', `a3') + 1 in 1 replace `yh' = f2(`a1', `a2', `a3') in 2 replace `yh' = f3(`a1', `a2', `a3') in 3 replace `varlist' = `yh' end
Here is an example. Suppose I want to solve the following system for A, B, and C:
exp(A) + B*C = 3 A/B + C^2 = log(B) A/(A+B+C) = sin(C)
Here is my nl program:
program nlfaq syntax varlist(min=1 max=1) [if], at(name) tempname A B C scalar `A' = `at'[1, 1] scalar `B' = `at'[1, 2] scalar `C' = `at'[1, 3] tempvar yh gen double `yh' = exp(`A') + `B'*`C' - 2 in 1 replace `yh' = `A'/`B' + `C'^2 - log(`B') in 2 replace `yh' = `A'/(`A'+`B'+`C') - sin(`C') in 3 replace `varlist' = `yh' end
Now I generate the dataset nl requires:
. clear . set obs 3 obs was 0, now 3 . generate y = 0 . replace y = 1 in 1 (1 real change made)
I estimate using nl:
. nl faq @ y, parameters(A B C) initial(A 1 B 1 C 1) (obs = 3) Iteration 0: residual SS = .5792985 Iteration 1: residual SS = .0364809 Iteration 2: residual SS = .0001378 Iteration 3: residual SS = 2.92e-09 Iteration 4: residual SS = 1.43e-18 Iteration 5: residual SS = 2.25e-31 Source | SS df MS -------------+------------------------------ Number of obs = 3 Model | 1 3 .333333333 R-squared = 1.0000 Residual | 2.2495e-31 0 . Adj R-squared = . -------------+------------------------------ Root MSE = . Total | 1 3 .333333333 Res. dev. = -206.4905 ------------------------------------------------------------------------------ y | Coef. Std. Err. t P>|t| [95% Conf. Interval] -------------+---------------------------------------------------------------- /A | .8973072 . . . . . /B | 1.803287 . . . . . /C | .3033412 . . . . . ------------------------------------------------------------------------------
Finally, I verify the solution:
. scalar A = [A]_b[_cons] . scalar B = [B]_b[_cons] . scalar C = [C]_b[_cons] . di exp(A) + B*C 3 . di A/B + C^2 " " log(B) .58961117 .58961117 . di A/(A+B+C) " " sin(C) .29871053 .29871053
Now let’s see how to do this with optimize():
. clear mata . mata: ------------------------------------------------- mata (type end to exit) ------ : : void mysolver(todo, p, lnf, S, H) > { > a = p[1] > b = p[2] > c = p[3] > lnf = (exp(a) + b*c - 3 )^2 \ > (a/b + c^2 - log(b))^2 \ > (a/(a+b+c) - sin(c))^2 > } note: argument todo unused note: argument S unused note: argument H unused : S = optimize_init() : optimize_init_evaluator(S, &mysolver()) : optimize_init_evaluatortype(S, "v0") : optimize_init_params(S, (1,1,1)) : optimize_init_which(S, "min" ) : optimize_init_tracelevel(S,"none") : optimize_init_conv_ptol(S, 1e-16) : optimize_init_conv_vtol(S, 1e-16) : p = optimize(S) : p 1 2 3 +-------------------------------------------+ 1 | .8973071614 1.803287112 .3033412094 | +-------------------------------------------+ : end --------------------------------------------------------------------------------
Notice that when using optimize, we don’t need to set up an independent variable y. We just define an evaluator function that, for each observation, evaluates to the square of one of the three expressions that need to be equal to zero.